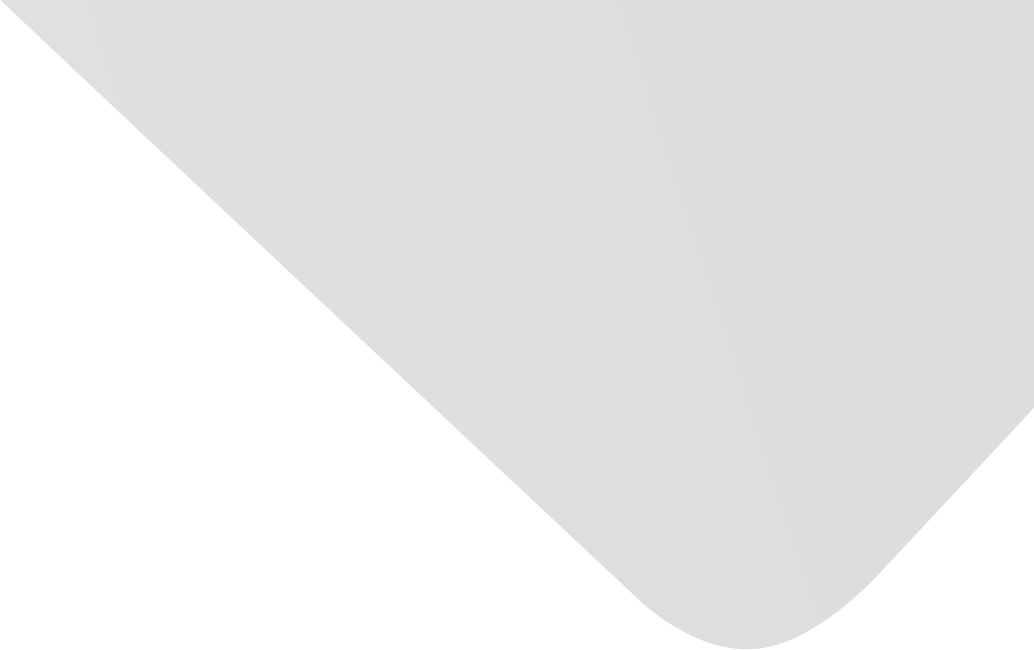
Dual stage bayesian network with dual-tree complex wavelet transformation for image denoising
Other Title(s)
استخدام شبكة بايزي ثنائية المرحلة مع التحويل المويجي المعقد ذي الشجرة الثنائية لتقليل تشويش الصور
Joint Authors
Venkata Lavanya, P.
Venkata Narasimhulu, C.
Satya Prasad, K.
Source
Journal of Engineering Research
Issue
Vol. 8, Issue 1 (31 Mar. 2020), pp.154-178, 25 p.
Publisher
Kuwait University Academic Publication Council
Publication Date
2020-03-31
Country of Publication
Kuwait
No. of Pages
25
Main Subjects
Abstract AR
إزالة التشويش من الصور تلعب دورا حيويا في العروض الهندسية المختلفة.
و كذلك في تكنولوجيا معالجة الصور، ظل الخلاف حول إحصاءات إزالة التشويش من الصورة قائما.
على مدى العقود الماضية، كشفت بعض الطرق لإزالة التشويش عن إنجازات لا تصدق.
و نظرا لعدم وجود مساهمات كبيرة في إزالة التشويش من الصور متعددة الوسائط وغير المتجانسة، فإن هذا البحث يعرض نهج ذكي لذلك.
تم استخدام التحويل المويجي المعقد ذي الشجرة الثنائية (DT-CWT) لتحويل الصور التي يتم تقدير معلمات مويجاتها باستخدام تنظيم بايزي (BR).
و لضمان تنفيذ عملية إزالة التشويش من الصور غير المتجانسة، تم استخراج الخصائص الإحصائية و المويجية.
بعد ذلك، تم دمج خصائص الصورة مع طيف التشويش لتطوير نموذج BR، و الذي يقدر معاملات المويجات لإزالة التشويش بشكل فعال.
و بالتالي، فإن خوارزمية إزالة التشويش المقترحة تستخدم مرحلتين من BR.
تتوقع المرحلة الأولى نوع الصورة، في حين أن المرحلة الثانية تقدر معاملات المويجات المناسبة للتحويل المويجي المعقد ذي الشجرة الثنائية لإزالة التشويش كمساهمة رئيسية، تم تحسين معاملات فلتر DT-CWT بواسطة الخوارزمية الجينية (GA).
تم تحليل أداء النموذج المقترح بواسطة مقياس نسبة قمة الإشارة إلى التشويش (PSNR)، الاشتقاق الثاني لقياس التحسين (SDME)، التشابه الهيكلي (SSIM)، خطأ التربيع المتوسط (MSE)، خطأ الجذر التربيعي (RMSE)، خطأ المتوسط المطلق (MAE)، و معامل بيرسون (PC)، وخطأ النسبة المئوية المطلقة لمتوسط متشابه (SMAPE) على التوالي.
وتم مقارنة النموذج المقترح بالنماذج التقليدية، و تم توضيح أهمية النموذج المطور بوضوح.
و لوحظ من التحليل أن PSNR للنموذج المطور هو %69.97، %5.85، %76.91، %33.38، %46.40 و 46.44% أفضل من DT- ،DT-CDWT ،DT-CWT 2D SMCWT W-ST ،RDWT و W-HT، على التوالي.
و بالمثل بالنسبة لقياس SSIM، فإن النموذج المقترح له انحراف كبير على الطرق التقليدية، و هو 19.17% 83.66%، 24.65%، 72.99% و 73.15% أفضل من DT-RDWT ، DT-CDWT DT-CWT ، W-ST و W-HT، على التوالي.
Abstract EN
Image de-noising always plays a vital role in various engineering bids.
Moreover, in image processing technology, image de-noising statistics is persisted as a substantial dispute.
Over the past decades, certain de-noising methods have been exposed incredible accomplishments.
This paper intends to develop a de-noising algorithm for multimodal and heterogeneous images, while the conventional de-noising algorithms handle a specific image type.
The filtered information is reversed to spatial domain to recover the de-noised image.
Dual tree Complex Wavelet Transform (DT-CWT) is exploited for image transformation for which the wavelet coefficients are estimated using Bayesian Regularization (BR).
To ensure the de-noising performance for heterogeneous images, the statistical and wavelet features are extracted.
Subsequently, the image characteristics are combined with noise spectrum to develop BR model, which estimates the wavelet coefficients for effective de-noising.
Hence, the proposed de-noising algorithm exploits two stages of BR.
The first stage predicts the image type, whereas the second stage estimates appropriate wavelet coefficients to DT-CWT for de-noising.
The performance of the proposed model is analysed in terms of Peak Signal to Noise Ratio (PSNR), Second derivative Measure of Enhancement (SDME), Structural Similarity (SSIM), Mean Squared Error (MSE), Root Mean Square Error (RMSE), Mean Absolute Error (MAE), Pearson Coefficient (PC), and Symmetric Mean Absolute Percentage Error (SMAPE) respectively.
The proposed model is compared to the conventional models, and the significance of the developed model is clearly described.
American Psychological Association (APA)
Venkata Lavanya, P.& Venkata Narasimhulu, C.& Satya Prasad, K.. 2020. Dual stage bayesian network with dual-tree complex wavelet transformation for image denoising. Journal of Engineering Research،Vol. 8, no. 1, pp.154-178.
https://search.emarefa.net/detail/BIM-1494878
Modern Language Association (MLA)
Venkata Lavanya, P.…[et al.]. Dual stage bayesian network with dual-tree complex wavelet transformation for image denoising. Journal of Engineering Research Vol. 8, no. 1 (Mar. 2020), pp.154-178.
https://search.emarefa.net/detail/BIM-1494878
American Medical Association (AMA)
Venkata Lavanya, P.& Venkata Narasimhulu, C.& Satya Prasad, K.. Dual stage bayesian network with dual-tree complex wavelet transformation for image denoising. Journal of Engineering Research. 2020. Vol. 8, no. 1, pp.154-178.
https://search.emarefa.net/detail/BIM-1494878
Data Type
Journal Articles
Language
English
Notes
Includes bibliographical references : p. 174-177
Record ID
BIM-1494878