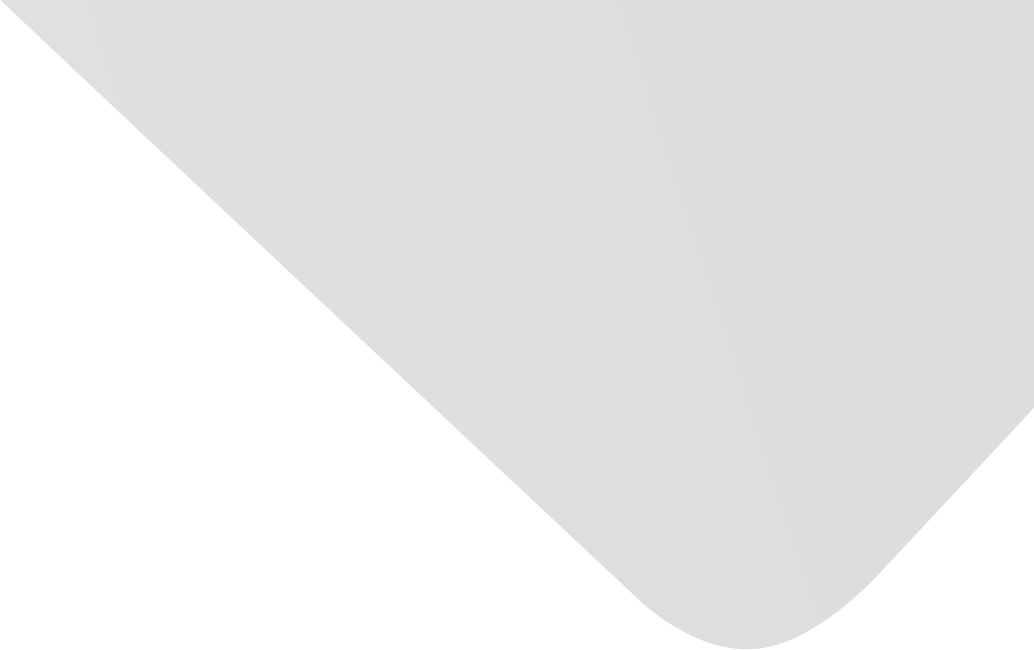
Bidirectional LSTM with saliency-aware 3D-CNN features for human action recognition
Joint Authors
Wang, Jing
al-Farra, Fida Husayn
Arif, Sheeraz
Siddiqui, Adnan Ahmed
Husayn, Fida
Source
Journal of Engineering Research
Issue
Vol. 9, Issue 3 A (30 Sep. 2021), pp.115-133, 19 p.
Publisher
Kuwait University Academic Publication Council
Publication Date
2021-09-30
Country of Publication
Kuwait
No. of Pages
19
Main Subjects
Abstract EN
Deep convolutional neural network (DCNN) and recurrent neural network (RNN) have been proved as an imperious research area in multimedia understanding and obtained remarkable action recognition performance.
However, videos contain rich motion information with varying dimensions.
Existing recurrent based pipelines fail to capture long-term motion dynamics in videos with various motion scales and complex actions performed by multiple actors.
Consideration of contextual and salient features is more important than mapping a video frame into a static video representation.
This research work provides a novel pipeline by analyzing and processing the video information using a 3D convolution (C3D) network and newly introduced deep bidirectional LSTM.
Like popular two-stream convent, we also introduce a two-stream framework with one modification; that is, we replace the optical flow stream by saliency-aware stream to avoid the computational complexity.
First, we generate a saliency-aware video stream by applying the saliency-aware method.
Secondly, a two-stream 3D-convolutional network (C3D) is utilized with two different types of streams, i.e., RGB stream and saliency-aware video stream, to collect both spatial and semantic temporal features.
Next, a deep bidirectional LSTM network is used to learn sequential deep temporal dynamics.
Finally, time-series-pooling-layer and softmax-layers classify human activity and behavior.
The introduced system can learn long-term temporal dependencies and can predict complex human actions.
Experimental results demonstrate the significant improvement in action recognition accuracy on different benchmark datasets.
American Psychological Association (APA)
Arif, Sheeraz& Wang, Jing& Siddiqui, Adnan Ahmed& al-Farra, Fida Husayn& Husayn, Fida. 2021. Bidirectional LSTM with saliency-aware 3D-CNN features for human action recognition. Journal of Engineering Research،Vol. 9, no. 3 A, pp.115-133.
https://search.emarefa.net/detail/BIM-1494898
Modern Language Association (MLA)
Arif, Sheeraz…[et al.]. Bidirectional LSTM with saliency-aware 3D-CNN features for human action recognition. Journal of Engineering Research Vol. 9, no. 3 A (Sep. 2021), pp.115-133.
https://search.emarefa.net/detail/BIM-1494898
American Medical Association (AMA)
Arif, Sheeraz& Wang, Jing& Siddiqui, Adnan Ahmed& al-Farra, Fida Husayn& Husayn, Fida. Bidirectional LSTM with saliency-aware 3D-CNN features for human action recognition. Journal of Engineering Research. 2021. Vol. 9, no. 3 A, pp.115-133.
https://search.emarefa.net/detail/BIM-1494898
Data Type
Journal Articles
Language
English
Notes
Includes bibliographical references : p. 131-133
Record ID
BIM-1494898