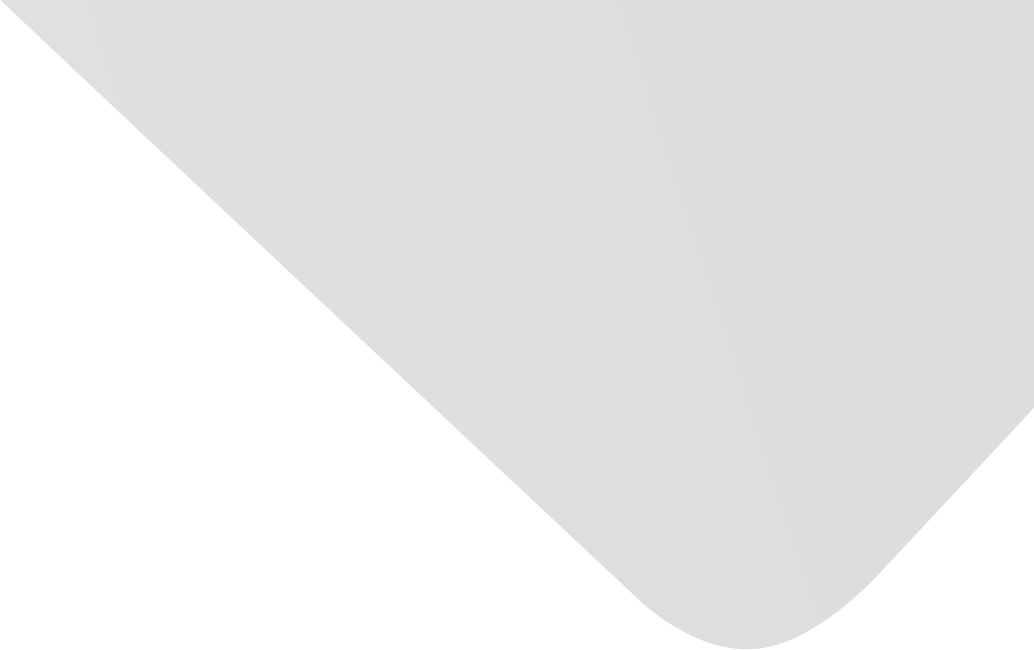
Comparison of fast regression algorithms in large datasets
Joint Authors
Cangur, Sengul
Ankarali, Handan
Source
Issue
Vol. 50, Issue 2 A (30 Apr. 2023), pp.1-17, 17 p.
Publisher
Kuwait University Academic Publication Council
Publication Date
2023-04-30
Country of Publication
Kuwait
No. of Pages
17
Main Subjects
Pharmacy, Health & Medical Sciences
Abstract EN
The aim is to compare the performances of fast regression methods, namely dimensional reduction of correlation matrix (DRCM), nonparametric dimensional reduction of correlation matrix (N-DRCM), variance inflation factor (VIF) regression, and robust VIF (R-VIF) regression in the presence of multicollinearity and outliers problems.
In all simulation-scenarios, all the target variables were chosen for final models using four methods.
The DRCM and N-DRCM are the methods that reach the final model in the shortest time, respectively.
The time to reach the final model using R-VIF regression was approximately twice shorter than that of VIF regression.
In each method, as the number of variables and the level of outliers increased, the time taken to reach the final model increased.
When the level of multicollinearity and the number of variables (p > 500) increased, the times to reach the final models using DRCM in datasets with outliers were slightly shorter than the those of N-DRCM.
The largest numbers of noise variables were selected to the model using DRCM and N-DRCM, but the least number of them were selected to the model using the R-VIF regression.
The RMSE values obtained using DRCM, N-DRCM and VIF regression were similar in each scenario.
As a result of the real dataset, the final model selected using R-VIF regression had the highest R 2 .
It also had the lowest RMSE value among those obtained with other approaches excluding VIF regression.
As such, the R-VIF regression method demonstrated a better performance than the others in all datasets.
American Psychological Association (APA)
Cangur, Sengul& Ankarali, Handan. 2023. Comparison of fast regression algorithms in large datasets. Kuwait Journal of Science،Vol. 50, no. 2 A, pp.1-17.
https://search.emarefa.net/detail/BIM-1501119
Modern Language Association (MLA)
Cangur, Sengul& Ankarali, Handan. Comparison of fast regression algorithms in large datasets. Kuwait Journal of Science Vol. 50, no. 2 A (Apr. 2023), pp.1-17.
https://search.emarefa.net/detail/BIM-1501119
American Medical Association (AMA)
Cangur, Sengul& Ankarali, Handan. Comparison of fast regression algorithms in large datasets. Kuwait Journal of Science. 2023. Vol. 50, no. 2 A, pp.1-17.
https://search.emarefa.net/detail/BIM-1501119
Data Type
Journal Articles
Language
English
Notes
Includes bibliographical references : p. 16-17
Record ID
BIM-1501119