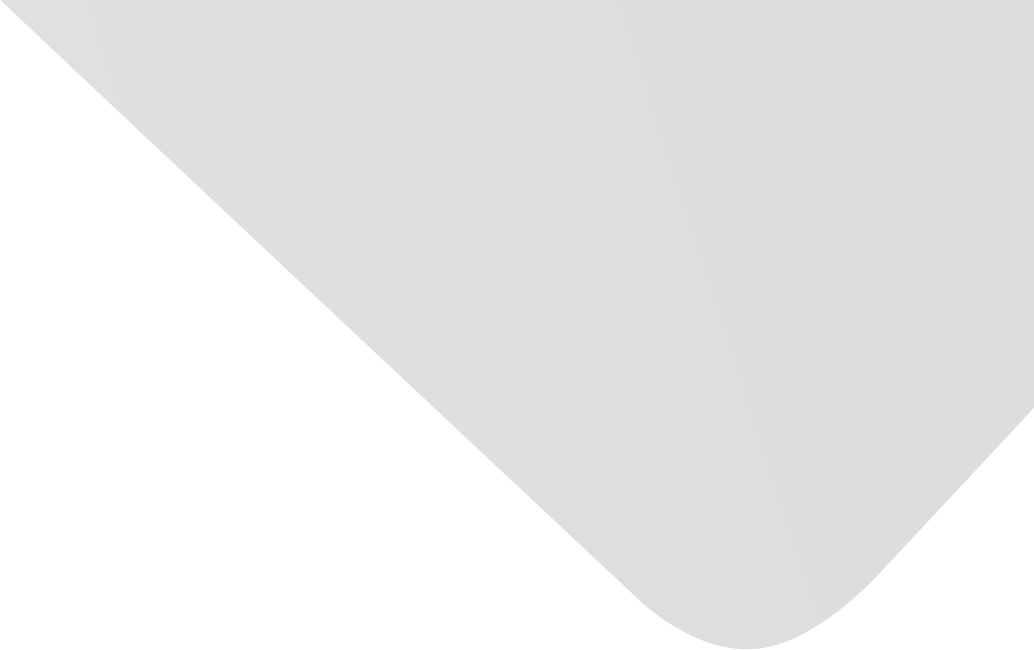
Deep learning model for Cutaneous leishmaniasis detection and classification using Yolov5
Other Title(s)
نموذج التعلم العميق للكشف عن داء الليشمانيا ت الجلدي وتصنيفه باستخدام Yolov5
Joint Authors
Nur al-Din, Abu Bakr Muhammad
Masud, Khalifah Salim
al-Makhzum, Usamah Abu al-Qasim
Source
African Journal of Advanced Pure and Applied Sciences
Issue
Vol. 2, Issue 2 (30 Apr. 2023), pp.270-280, 11 p.
Publisher
African Academy of Advanced Studies
Publication Date
2023-04-30
Country of Publication
Libya
No. of Pages
11
Main Subjects
Information Technology and Computer Science
Abstract AR
الندبات وتقرحات الجلدية هي سمة من سمات العديد من الأمراض الجلدية بما في ذلك داء الليشمانيات الجلدي (CL) في المناطق الموبوءة و الثانية، يظل تحديد العدوى يمثل تحديا للطبيب و النظام الصحي و المريض.
تقدم هذه الدراسة طريقة تشخيصية جديدة مهمة للكشف السريع و التشخيص الدقيق من خلال إنشاء نموذج تدريب YOLOv5 للتعرف على داء الليشمانيات الجلدي (CL) في ظل الظروف العادية بناء على شبكة YOLOv5 التي تعتمد على نهج التعلم العميق.
ترتبط بيئة التطوير بلغة بايثون.
تحتوي مجموعة بيانات التدريب على إجمالي 160 صورة تم التقاطها من كاميرا هاتف محمول و تحويلها إلى صور ذات تدرج رمادي لاستخراج الميزات المميزة ثم تطبيق تقنيات معالجة الصور مثل التقليب و التدوير و تغيير الحجم لزيادة معلومات مجموعة البيانات.
تم عنونة الصور مع طبيب الأمراض الجلدية للتأكد من إصابة داء الليشمانيات الجلدي (CL).
في نهجنا، تم تصنيف الصور باستخدام مربعات إحاطة متعددة الأضلاع لتحديد مجالات الاهتمام بحيث تم تقسيم مجموعة البيانات إلى التحقق من الصحة و التدريب و الاختبار.
تدفقت عن طريق تغذية مجموعة البيانات في YOLOv5s، استطاع نموذجنا تحديد موقع داء الليشمانيات الجلدي (CL) و حقق دقة عالية في العدوى و تصنيفها بمتوسط دقة بلغة 70%، مما توفر تقنية سريعة للكشف.
Abstract EN
Scars and skin ulcers are a feature of many skin diseases, including cutaneous leishmaniasis (CL).
in endemic areas and developing countries, infection identification remains a challenge for the physician, the health system, and the patient.
this study introduces an important new diagnostic method for rapid detection and accurate diagnosis by establishing a YOLOv5 training model to recognize cutaneous leishmaniasis (CL), under normal conditions based on the YOLOv5 network depends upon a deep learning approach.
the development environment is related to python language.
the training data set contains a total of 160 photos taken from a mobile phone camera and converted to grayscale images to extract characteristic features and then applied image processing techniques such as flipping, rotating, and resizing to increase the dataset information.
image labeling was identified with a dermatologist to ensure the injury of cutaneous leishmaniasis (CL).in our approach, images were classified using polygonal bounding boxes to identify areas of interest so that dataset was divided into validation, training, and testing.
flowed by feeding the dataset in the YOLOv5s.
our model was able to locate cutaneous leishmaniasis (CL) and achieved high accuracy in detecting and classifying infections, with an average accuracy of 70%.
which provides a speed technology for detection.
American Psychological Association (APA)
Nur al-Din, Abu Bakr Muhammad& Masud, Khalifah Salim& al-Makhzum, Usamah Abu al-Qasim. 2023. Deep learning model for Cutaneous leishmaniasis detection and classification using Yolov5. African Journal of Advanced Pure and Applied Sciences،Vol. 2, no. 2, pp.270-280.
https://search.emarefa.net/detail/BIM-1508463
Modern Language Association (MLA)
Nur al-Din, Abu Bakr Muhammad…[et al.]. Deep learning model for Cutaneous leishmaniasis detection and classification using Yolov5. African Journal of Advanced Pure and Applied Sciences Vol. 2, no. 2 (Apr. / Jun. 2023), pp.270-280.
https://search.emarefa.net/detail/BIM-1508463
American Medical Association (AMA)
Nur al-Din, Abu Bakr Muhammad& Masud, Khalifah Salim& al-Makhzum, Usamah Abu al-Qasim. Deep learning model for Cutaneous leishmaniasis detection and classification using Yolov5. African Journal of Advanced Pure and Applied Sciences. 2023. Vol. 2, no. 2, pp.270-280.
https://search.emarefa.net/detail/BIM-1508463
Data Type
Journal Articles
Language
English
Notes
Includes bibliographical references : p. 278-280
Record ID
BIM-1508463