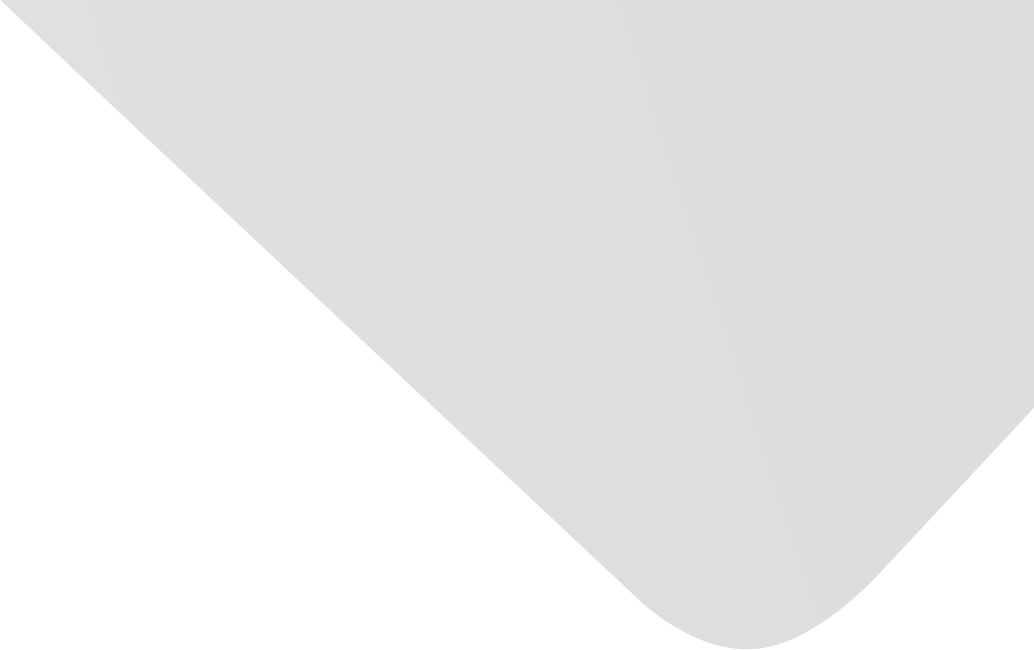
Comparison of various machine learning regression models based on human age prediction
Author
Source
Wasit Journal of Engineering Sciences
Issue
Vol. 10, Issue 3 (30 Sep. 2022), pp.1-16, 16 p.
Publisher
Wasit University College of Engineering
Publication Date
2022-09-30
Country of Publication
Iraq
No. of Pages
16
Main Subjects
Abstract AR
إن التطور الحاصل في مجال التعلم الآلي جعل من الممكن تشخيص بعض الأمراض تلقائيا بناء على البيانات التي تم الحصول عليها من التصوير الطبي.
يعد عمر الدماغ أحد العوامل التي يمكن استخدامها كمؤشر على الرفاهية المعرفية.
حيث من خلال معرفة عمر الدماغ ومقارنته مع العمر الحقيقي للفرد يمكن معرفة صحة عمر الدماغ وإمكانية الإصابة بمصطلح ما يعرف بالشيخوخة المتقدمة أو المتأخرة والتي تعتبر كمؤشر حيوي للكثير من الوظائف الإدراكية.
لقد جعلت التطورات الحديثة في التعلم الآلي من الممكن لأجهزة الكمبيوتر توقع النتائج بشكل أكثر دقة من البشر.
في هذه الدراسة، تم استخدام خمسة نماذج مستخدمة على نطاق واسع من تحليل الانحدار الموجودة ضمن نظمة التعلم الآلي وهي انحدار ناقل الدعم الخطي (L-SVR)، استخدام خاصية وظيفة الأساس الشعاعي التي تدعم الانحدار المتجه (RBF-SVR)، انحدار متجه الصلة (RVR)، الشبكة المرنة وانحدار العملية الغاوسية (GPR).
تم تدريب هذه النماذج للحصول على تنبؤ لعمر الدماغ البشري باستخدام بيانات مأخوذة من مناطق الدماغ.
علاوة على ذلك، تم استخدام تقنية تقليل الخصائص لتقليل خصائص بيانات الإدخال.
تم جمع البيانات من مئة واحد عشر مشاركا.
أظهرت النتائج عدم وجود فرق في الأداء بين النماذج المدربة على نفس النوع من البيانات، مما يشير إلى أن نوع بيانات الإدخال كان له تأثير أقوى على الأداء من اختيار النموذج.
تشير النتائج إلى أن GPR كان أفضل نموذج ملائم للتنبوء 0.57 = 0.75 = R من بين نماذج الانحدار الأخرى بينما كان G-SVR هو النموذج الأسوأ من حيث دقة التنبؤ (0.025 = 0.0006 =R) مع هذا العدد من المدخلات بيانات.
Abstract EN
The development of machine learning strategies has made it possible to diagnose some disease automatically based on data obtained from medical imaging.
Brain age is one of the factors that can be used as an indicator of cognitive well-being.
Recent advancements in machine learning have made it possible for computers to anticipate classification and prediction outcomes more accurately than humans.
In this study, five widely used machine learning regression models (Linear support vector regression (L-SVR), radial basis function support vector regression (RBF-SVR), relevance vector regression (RVR), Elastic Net and Gaussian process regression (GPR)) were trained and evaluated to predict brain age using volumes of brain regions data.
Moreover, a dimensionality reduction technique was utilized to reduce the dimensionality of the input feature space.
The data were collected from one hundred and eleven participants.
The results showed no performance difference amongst models trained on the same type of data, suggesting that the type of input data had a stronger influence on prediction performance than the model choice.
The experimental results indicated that the GPR was the best fit model (R2=0.57, R=0.75) among the other regression models while the G-SVR was the worst fit model (R2=0.0006, R=0.025) with such number of the input data.
American Psychological Association (APA)
al-Talib, Munaf Kazim Husayn. 2022. Comparison of various machine learning regression models based on human age prediction. Wasit Journal of Engineering Sciences،Vol. 10, no. 3, pp.1-16.
https://search.emarefa.net/detail/BIM-1572708
Modern Language Association (MLA)
al-Talib, Munaf Kazim Husayn. Comparison of various machine learning regression models based on human age prediction. Wasit Journal of Engineering Sciences Vol. 10, no. 3 (2022), pp.1-16.
https://search.emarefa.net/detail/BIM-1572708
American Medical Association (AMA)
al-Talib, Munaf Kazim Husayn. Comparison of various machine learning regression models based on human age prediction. Wasit Journal of Engineering Sciences. 2022. Vol. 10, no. 3, pp.1-16.
https://search.emarefa.net/detail/BIM-1572708
Data Type
Journal Articles
Language
English
Notes
Includes bibliographical references : p. 14-16
Record ID
BIM-1572708