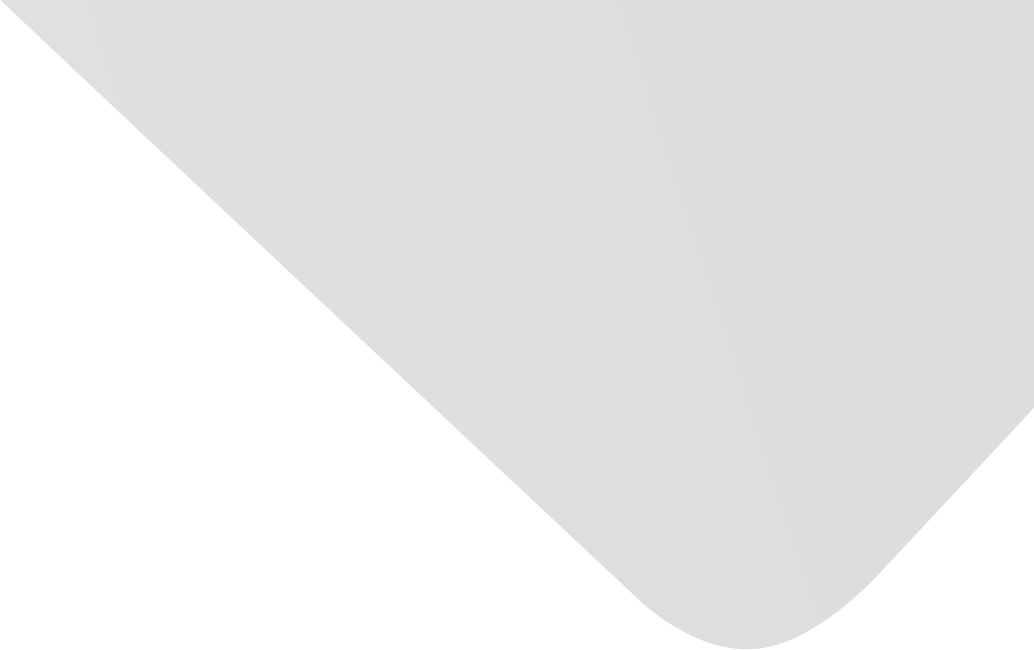
Improvement the community detection with graph auto encoder in social network using correlation- based feature selection method
Other Title(s)
في الشبكات Graph Autoencoder تحسين اكنشاف المجتمع ب الاجنماعية باستخدام طريقة تحديد الميزات القائمة على الارتباط
Joint Authors
al-Zubaydi, Asia al-Mahdi Nasir
Ahmad, Hawraa Zuhayr
Source
Journal of Babylon University : Journal of Applied and Pure Sciences
Issue
Vol. 30, Issue 4 (31 Dec. 2022), pp.20-33, 14 p.
Publisher
Publication Date
2022-12-31
Country of Publication
Iraq
No. of Pages
14
Main Subjects
Information Technology and Computer Science
Abstract AR
مقدمة: في هذا البحث، نهدف إلى تحسين طرق اكتشاف المجتمع باستخدام Graph Auto encoder.
يعد اكتشاف المجتمع مرحلة حاسمة لفهم الشبكات الاجتماعية وتكوينها.
طرق العمل: نقترح إطار عمل اكتشاف المجتمع باستخدام نموذج (Graph Auto encoder (CDGAE، حيث قمنا بدمج ميزة العقد مع هيكل الشبكة كمدخل لطريقتنا.
تستخدم CDGAE استراتيجية قائمة على قياس المركزية للتعامل مع مجموعة البيانات الخالية من الميزات من خلال توفير ميزات اصطناعية لعقدها.
تم تحسين أداء النموذج من خلال تطبيق تحديد الميزة على ميزات العقدة.
يتمثل الابتكار الأساسي لـ CDGAE في إضافة عدد المجتمعات التي تم حسابها باستخدام Bethe Hessian Matrix في طبقة عنق الزجاجة لبنية (Graph Auto encoder (GAE، لاستخراج المجتمعات مباشرة دون استخدام أي خوارزميات تجميع.
الاستنتاجات: وفقا للنتائج التجريبية، تؤدي إضافة ميزات اصطناعية إلى عقد مجموعة البيانات إلى تحسين الأداء.
بالإضافة إلى ذلك، حصلنا على نتائج أفضل بكثير في اكتشاف المجتمع باستخدام طريقة اختيار الميزة وبتعميق نموذج أظهرت النتائج التجريبية أن نهجنا يتفوق على الخوارزميات الموجودة.
Abstract EN
Background: In this paper, we aim to improve community detection methods using Graph Auto encoder.
Community detection is a crucial stage in comprehend the purpose and composition of social networks.
Materials and Methods: We propose a Community Detection framework using the Graph Auto encoder (CDGAE) model; we combined the nodes feature with the network topology as input to our method.
A centrality measurement- based strategy is used by CDGAE to deal with the featureless dataset by providing artificial attributes to its nodes.
The performance of the model was improved by applying feature selection to node features The basic innovation of CDGAE is that added the number of communities counted using the Bethe Hessian Matrix in the bottleneck layer of the graph auto encoder (GAE) structure, to directly extract communities without using any clustering algorithms.
Results: According to experimental findings, adding artificial features to the dataset's nodes improves performance.
Additionally, the outcomes in community detection were much better with the feature selection method and a deeper model.
Experimental evidence has shown that our approach outperforms existing algorithms.
Conclusion: In this study, we suggest a community detection framework using graph autoencoder (CDMEC).
In order to take advantage of GAE's ability to combine node features with the network topology, we add node features to the featureless graph nodes using centrality measurement.
By applying the feature selection to the features of the nodes, the performance of the model has improved significantly, due to the elimination of data noise.
Additionally, the inclusion of the number of communities in the bottleneck layer of the GAE structure allowed us to do away with clustering algorithms, which helped decrease the complexity time.
Deepening the model also improved the community detection.
Because social media platforms are dynamic.
American Psychological Association (APA)
Ahmad, Hawraa Zuhayr& al-Zubaydi, Asia al-Mahdi Nasir. 2022. Improvement the community detection with graph auto encoder in social network using correlation- based feature selection method. Journal of Babylon University : Journal of Applied and Pure Sciences،Vol. 30, no. 4, pp.20-33.
https://search.emarefa.net/detail/BIM-1595385
Modern Language Association (MLA)
Ahmad, Hawraa Zuhayr& al-Zubaydi, Asia al-Mahdi Nasir. Improvement the community detection with graph auto encoder in social network using correlation- based feature selection method. Journal of Babylon University : Journal of Applied and Pure Sciences Vol. 33, no. 4 (2022), pp.20-33.
https://search.emarefa.net/detail/BIM-1595385
American Medical Association (AMA)
Ahmad, Hawraa Zuhayr& al-Zubaydi, Asia al-Mahdi Nasir. Improvement the community detection with graph auto encoder in social network using correlation- based feature selection method. Journal of Babylon University : Journal of Applied and Pure Sciences. 2022. Vol. 30, no. 4, pp.20-33.
https://search.emarefa.net/detail/BIM-1595385
Data Type
Journal Articles
Language
English
Notes
Includes bibliographical references : p. 31-33
Record ID
BIM-1595385