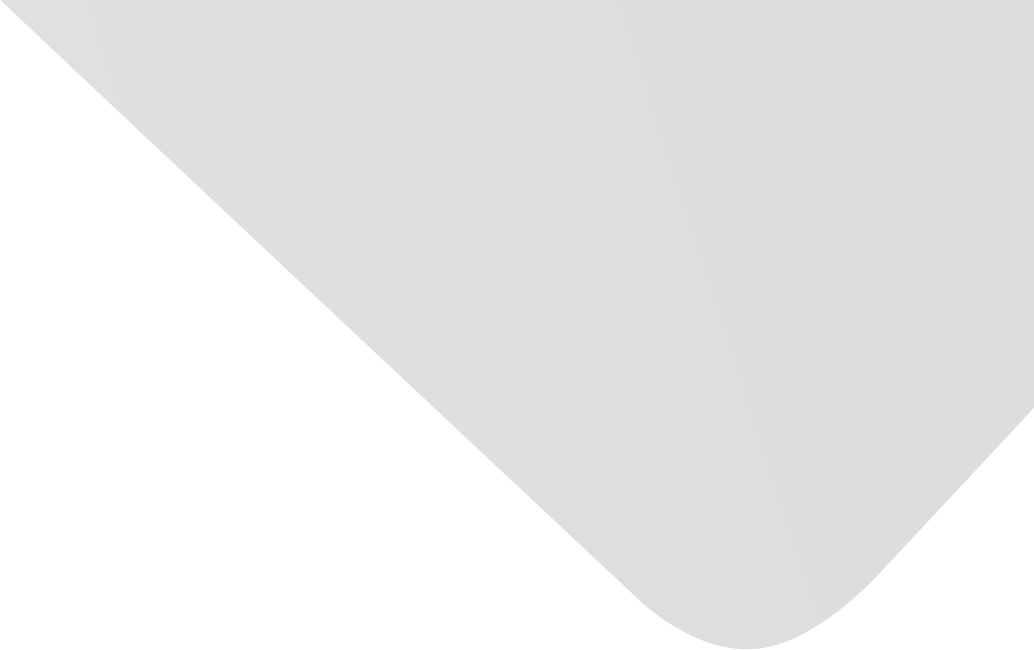
Handwritten recognition system based on machine learning
Other Title(s)
نظام التعرف بخط اليد على أساس التعلم الآلي
Joint Authors
al-Yasiri, Ali Abd al-Karim Salman
Ubays, Hadab Khalid
Source
Journal of Babylon University : Journal of Applied and Pure Sciences
Issue
Vol. 30, Issue 4 (31 Dec. 2022), pp.34-43, 10 p.
Publisher
Publication Date
2022-12-31
Country of Publication
Iraq
No. of Pages
10
Main Subjects
Abstract AR
مقدمة: يعد التعرف على خط اليد قضية مهمة في الوقت الحاضر، حيث يمكن أن تكون الكتابة اليدوية صورة أو مستندا وما إلى ذلك، تعد قدرة الكمبيوتر على التعرف على الأرقام المكتوبة بخط اليد مهمة جدا في أكثر من تطبيق مثل تطبيقات الترجمة والقراءة والتعرف على الأرقام.
يوفر المشروع المقترح نظاما يتعرف على الأرقام الإنجليزية المكتوبة بخط اليد، ويتم تنزيل بيانات الإدخال من مجموعة بيانات عالمية.
يتكون النظام المقترح من عدد من المراحل المرحلة الأولى هي المعالجة المسبقة، والتي تتضمن تغيير حجم الصور لتكون بحجم واحد 28*28، ثم يتم تطبيق خطوة تعيين البيانات).
أما بالنسبة لمرحلة التصنيف، فقد اعتمدت على استخدام خوارزميتين، خوارزمية KNN والشبكة العصبية خطأ.back propagation).
لبدء عملية تدريب الخوارزميات المختارة، تم تقسيم البيانات إلى مجموعتين، مجموعة التدريب ومجموعة الاختبار.
تم استخدام خوارزميتين لغرض اختيار أفضلها من خلال تقييم أدائها باستخدام عدد من مقاييس التقييم.
تم استخدام الدقة والدقة لغرض تقييم أداء الخوارزميات كان أداء خوارزمية 0.94 KNN و0.942 على التوالي عند 4 -k.
بينما كان أفضل أداء وصلت إليه آلية الشبكة العصبية 0.98673333 و0.9698 على التوالي، في العصر-15.
تظهر الشبكة العصبية (خطأ back propagation) أفضل نتيجة في مرحلة الاعتراف طرق العمل: لا تقدم تقنية (KNN) أي افتراضات حول مجموعة البيانات الأساسية.
إنه معروف بفعاليته وسهولة استخدامه إنها خوارزمية تعلم خاضعة للإشراف لتقدير فئة البيانات غير المسماة، يتم توفير مجموعة تدريب معنونه تحتوي على نقاط بيانات مقسمة إلى مجموعات عديدة.
الاستنتاجات: توضح مؤشرات الدقة والدقة وصفا دقيقا لأداء الخوارزميات المستخدمة في النظام المقترح.
وصف المؤشرين أداء الخوارزمية (KNN) والتي أعطت النتائج (0.94) و (0.942(على التوالي.
Abstract EN
Background: Handwriting recognition is an important issue nowadays, where handwriting can be a image, document, etc., the ability of a computer to recognize handwritten numbers is very important in more than one application such as translation, reading and number recognition applications.
The proposed project provides a system that recognizes handwritten English numbers, the input data being images downloaded from a global dataset.
The proposed system consists of a number of stages.
The first stage is the preprocessing, which includes resizing of the images to be one size (28 * 28), and then a step (data mapping) is applied.
As for the classification stage, it relied on the use of two algorithms, the KNN algorithm and the neural network (error back propagation).
To start the process of training the selected algorithms, the data was divided into two sets, the training set and the test set.
Two algorithms were used for the purpose of choosing the best of them, by evaluating their performance using a number of evaluation metrics.
Accuracy and Precision were used for the purpose of evaluating the performance of the algorithms.
The performance of the KNN algorithm was 0.94 and 0.942 respectively when k = 4.
While the best performance reached by the neural network mechanism was 0.98673333 and 0.9698, respectively, at epoch=15.
The neural network (error back propagation) is shows the best result in the recognition stage.
Materials and Methods: K-Nearest Neighbors (KNN) technique makes no assumptions about the basic dataset.
It is recognized for its effectiveness and ease of use.
It is a supervised learning algorithm.
To estimate the category of the unlabeled data, a labeled training set containing data points separated into many groups is supplied.
Results: The performance of the KNN model with various values for "K." Since the high value of model accuracy was "0.94", the "4" parameter value is the one that provides the best results and precision was "0.94".
Conclusion: The problem of handwritten recognition needs high accuracy and precision indicators show an accurate description of the performance of the algorithms that were employed in the proposed system.
The two indicators described the performance of the algorithm (KNN), which gave results (0.94 and 0.942).
American Psychological Association (APA)
al-Yasiri, Ali Abd al-Karim Salman& Ubays, Hadab Khalid. 2022. Handwritten recognition system based on machine learning. Journal of Babylon University : Journal of Applied and Pure Sciences،Vol. 30, no. 4, pp.34-43.
https://search.emarefa.net/detail/BIM-1595392
Modern Language Association (MLA)
al-Yasiri, Ali Abd al-Karim Salman& Ubays, Hadab Khalid. Handwritten recognition system based on machine learning. Journal of Babylon University : Journal of Applied and Pure Sciences Vol. 33, no. 4 (2022), pp.34-43.
https://search.emarefa.net/detail/BIM-1595392
American Medical Association (AMA)
al-Yasiri, Ali Abd al-Karim Salman& Ubays, Hadab Khalid. Handwritten recognition system based on machine learning. Journal of Babylon University : Journal of Applied and Pure Sciences. 2022. Vol. 30, no. 4, pp.34-43.
https://search.emarefa.net/detail/BIM-1595392
Data Type
Journal Articles
Language
English
Notes
Includes bibliographical references : p. 42-43
Record ID
BIM-1595392