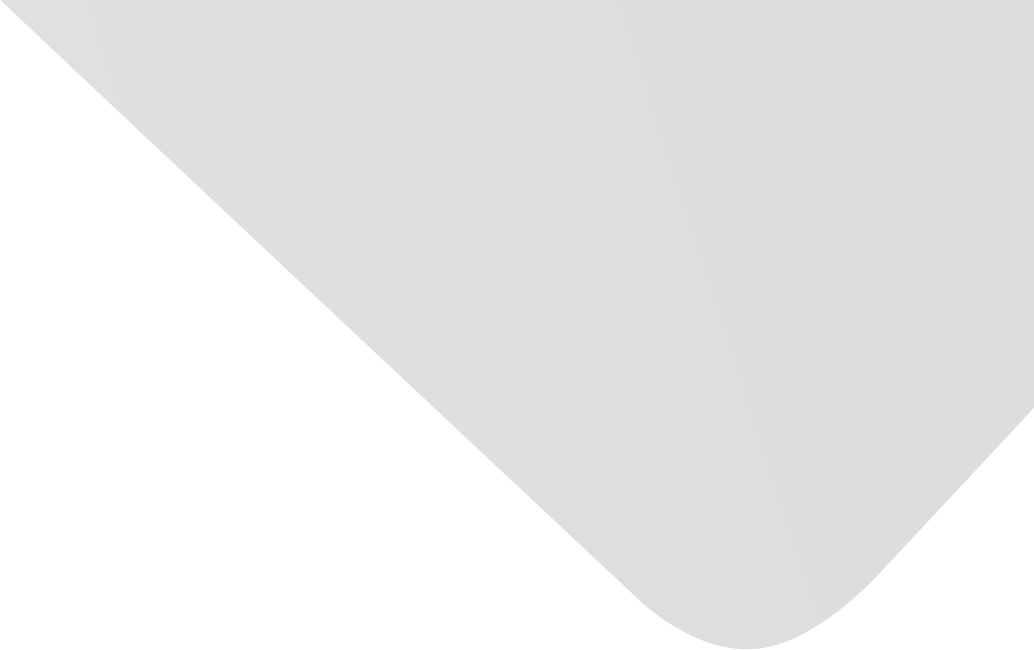
Recurrent neural network for multi-steps ahead prediction of pm10 concentration
Joint Authors
Ghazi, Sabri
Khidr, Muhammad Tariq
Source
Journal of Automation and Systems Engineering
Issue
Vol. 3, Issue 2 (30 Jun. 2009)9 p.
Publisher
Publication Date
2009-06-30
Country of Publication
Algeria
No. of Pages
9
Main Subjects
Earth Sciences, Water and Environment
Information Technology and Computer Science
Topics
Abstract EN
This paper describes the development of a Multi Layer Perceptron (MLP) recurrent neural network based model to perform a multi-steps ahead prediction of pollutant concentration.
Receiving the latest k measurements of pollutant concentration and the meteorological parameters the model is able to predict the next k hourly concentration.
The model has been applied to predict the pmio (Particulate Matter with an aerodynamic diameter ofio micrometer) concentration in Annaba city, northeast of Algeria (north of Africa).
Compared with a single step prediction MIA model the recurrent model perform best for short-term prediction and give interesting performances for long-term prediction.
American Psychological Association (APA)
Ghazi, Sabri& Khidr, Muhammad Tariq. 2009. Recurrent neural network for multi-steps ahead prediction of pm10 concentration. Journal of Automation and Systems Engineering،Vol. 3, no. 2.
https://search.emarefa.net/detail/BIM-180882
Modern Language Association (MLA)
Ghazi, Sabri& Khidr, Muhammad Tariq. Recurrent neural network for multi-steps ahead prediction of pm10 concentration. Journal of Automation and Systems Engineering Vol. 3, no. 2 (Jun. 2009).
https://search.emarefa.net/detail/BIM-180882
American Medical Association (AMA)
Ghazi, Sabri& Khidr, Muhammad Tariq. Recurrent neural network for multi-steps ahead prediction of pm10 concentration. Journal of Automation and Systems Engineering. 2009. Vol. 3, no. 2.
https://search.emarefa.net/detail/BIM-180882
Data Type
Journal Articles
Language
English
Notes
Includes bibliographical references.
Record ID
BIM-180882