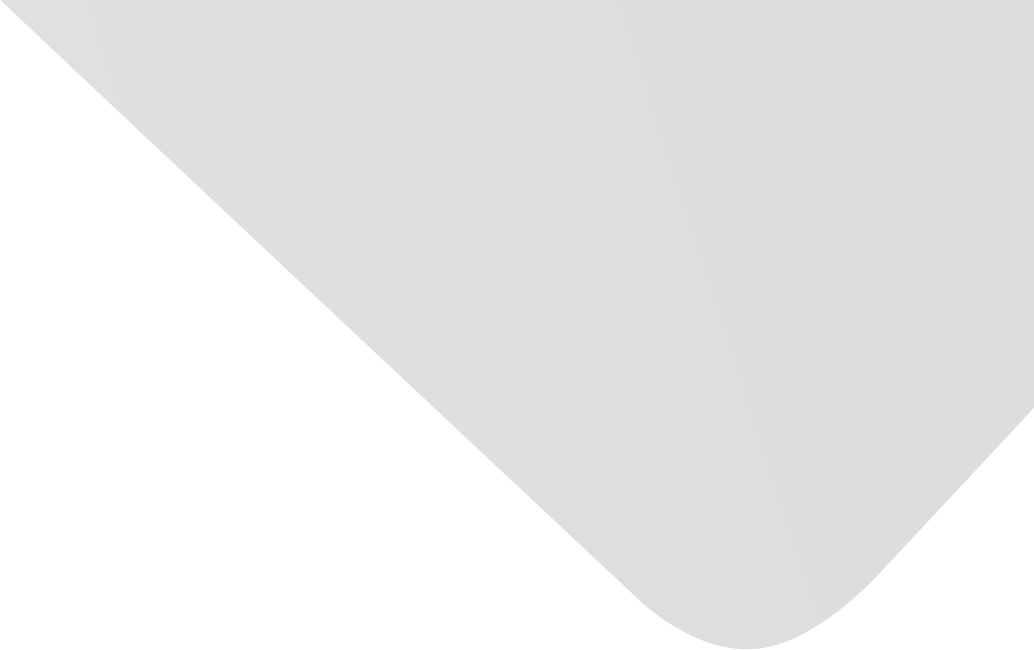
Training artificial neural network using back-propagation & particle swarm optimization for image skin diseases
Other Title(s)
تدريب الشبكة العصبية الاصطناعية باستخدام أمثلية الحشد الجزيئي و الانتشار العكسي للصور أمراض الجلد
Joint Authors
Akkar, Hanan Abd al-Rida
Salman, Samim Abbas
Source
Engineering and Technology Journal
Issue
Vol. 29, Issue 13 (31 Jan. 2011), pp.2739-2752, 14 p.
Publisher
Publication Date
2011-01-31
Country of Publication
Iraq
No. of Pages
14
Main Subjects
Engineering & Technology Sciences (Multidisciplinary)
Topics
Abstract AR
هذا العمل مكرس لدراسة ضغط صور لأمراض الجلد بواسطة استخدام محول الموجات المنفصلة (DWT) ثم يتم تدريبها بواسطة الشبكة العصبية الاصطناعية باستخدام أمثلية الحشد الجزيئي (PSO) و مقارنتها بالانتشار العكسي (BP) من حيث (الدقة–عدد hidden layer–الزمن–الأوزان–عدد التكرار للتدريب).
استخدام برنامج الماتلاب 6.5 للحصول على النتائج.
تم اقتراح مجموعة من تصاميم الشبكات العصبية الاصطناعية التي تدرب عدد من صور أمراض الجلد و هي كما يلي : 1- التصميم المقترح للشبكة العصبية الاصطناعية التي تنفذ ثلاثة صور من أمراض الجلد بواسطة (BP) الانتشار العكسي.
2- التصميم المقترح للشبكة العصبية الاصطناعية التي تنفذ ثلاثة صور من أمراض الجلد بواسطة (PSO) أمثلية الحشد الجزيئي.
و بمقارنة النتائج التي ظهرت باستخدام خوارزمية PSO المقترحة مع تلك النتائج التي ظهرت باستخدام خوارزمية الانتشار العكسي و هي (التكرار) (4700-602 دورة)، الزمن (1 ثانية إلى 100 ثانية)، عدد الأوزان الابتدائية (1 مجموعة إلى 75 مجموعة)، عدد المشتقات (0 إلى 38 مشتقة) و الدقة (60 % إلى 100 %) استخدمت كمقاييس للمقارنة.
و بمعدل مربع الخطأ.
10-7 وجدت أن نتائج الشبكات العصبية الاصطناعية المقترحة بواسطة أفضلية الحشد الجزيئي أفضل خوارزمية لتدريب الشبكات العصبية الاصطناعية و التي تطابق البحث في هذا المجال من حيث السرعة، الدقة و الكفاءة.
Abstract EN
This work is devoted to compression Image Skin Diseases by using Discrete Wavelet Transform (DWT) and training Feed-Forward Neural Networks (FFNN) by using Particle Swarm Optimization(PSO) and compares it with Back-Propagation (BP) neural networks in terms of convergence rate and accuracy of results .The comparison between the two techniques will be mentioned.
A MATLAB 6.5 program is used in simulation.
The structure Artificial Neural Network (ANN) of training image skin diseases is proposed as follows: 1- The proposed structure of NN that performs three compressions Images Skin training by BP algorithms with log sigmoid activation function, and three neurons in output layer.
2- The proposed structure of FFNN using PSO that performs three compressions Images Skin with hardlim activation function, and three neurons in output layer.
The results obtained using PSO are compared to those obtained using BP.
Learning iterations (602-4700 epoch), convergence time (1sec.- 100 sec.), number of initial weights (1set - 75set), number of derivatives (0 - 38 derivatives) and accuracy (60% - 100%) are used as performance measurements.
The obtained Mean Square Error (MSE) is 7 10 - to check the performance of algorithms.
The results of the proposed neural networks performed indicate that PSO can be a superior training algorithm for neural networks, which is consistent with other research in the area.
American Psychological Association (APA)
Akkar, Hanan Abd al-Rida& Salman, Samim Abbas. 2011. Training artificial neural network using back-propagation & particle swarm optimization for image skin diseases. Engineering and Technology Journal،Vol. 29, no. 13, pp.2739-2752.
https://search.emarefa.net/detail/BIM-289615
Modern Language Association (MLA)
Akkar, Hanan Abd al-Rida& Salman, Samim Abbas. Training artificial neural network using back-propagation & particle swarm optimization for image skin diseases. Engineering and Technology Journal Vol. 29, no. 13 (2011), pp.2739-2752.
https://search.emarefa.net/detail/BIM-289615
American Medical Association (AMA)
Akkar, Hanan Abd al-Rida& Salman, Samim Abbas. Training artificial neural network using back-propagation & particle swarm optimization for image skin diseases. Engineering and Technology Journal. 2011. Vol. 29, no. 13, pp.2739-2752.
https://search.emarefa.net/detail/BIM-289615
Data Type
Journal Articles
Language
English
Notes
Includes appindix : p. 2748-2752
Record ID
BIM-289615