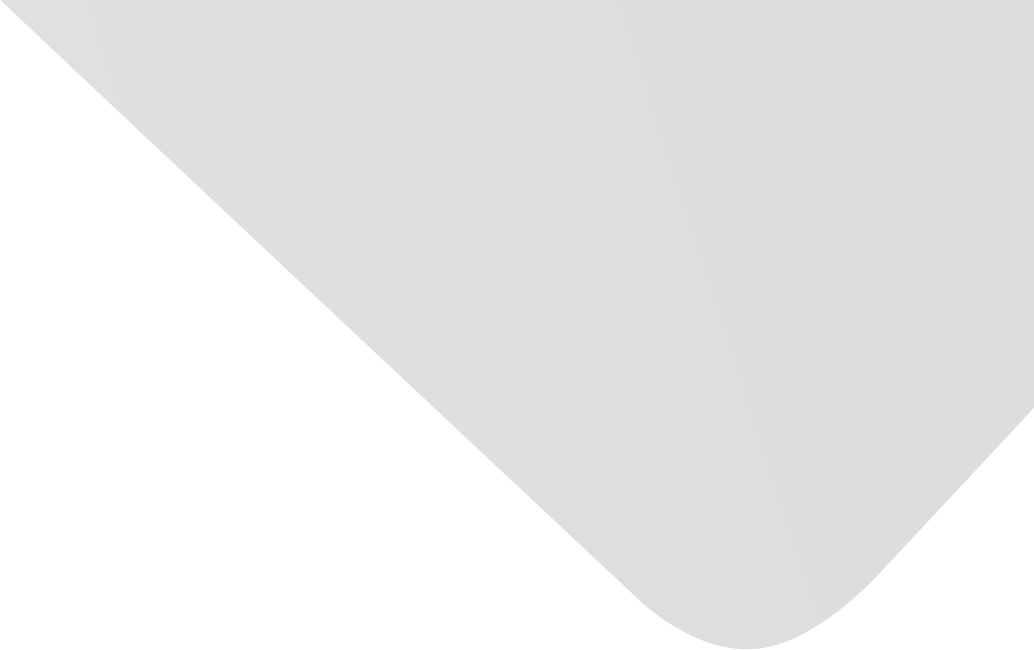
Breast cancer diagnosis using artificial intelligence neural networks
Joint Authors
Abd al-Qadir, Howida Ali
Hamzah, Muhammad Hasan
Source
Journal of Science and Technology
Issue
Vol. 12, Issue 1 (30 Jun. 2011), pp.159-171, 13 p.
Publisher
Sudan University of Science and Technology Deanship of Scientific Research
Publication Date
2011-06-30
Country of Publication
Sudan
No. of Pages
13
Main Subjects
Medicine
Information Technology and Computer Science
Topics
Abstract EN
Breast cancer is the second largest cause of cancer deaths among women.
Breast cancer is one of the major tumor related cause of death in women.
Various artificial intelligence techniques have been used to improve the diagnoses procedures.
The present study aimed at detecting breast cancer by using three neural network topologies which are Multi Layer Perceptron (MLP), Generalized Regression (GRNN) and Probabilistic (PNN).
Three Wisconsin breast cancer data sets (WBCD) were examined by these networks.
It was seen that the most suitable neural network model for classifying WBCD are MLP and GRNN, and observing that PNN performance was low in case of small datasets.
KEYWORDS: Artificial neural networks, breast cancer, MLP, GRNN, PNN
American Psychological Association (APA)
Abd al-Qadir, Howida Ali& Hamzah, Muhammad Hasan. 2011. Breast cancer diagnosis using artificial intelligence neural networks. Journal of Science and Technology،Vol. 12, no. 1, pp.159-171.
https://search.emarefa.net/detail/BIM-298736
Modern Language Association (MLA)
Abd al-Qadir, Howida Ali& Hamzah, Muhammad Hasan. Breast cancer diagnosis using artificial intelligence neural networks. Journal of Science and Technology Vol. 12, no. 1 (2011), pp.159-171.
https://search.emarefa.net/detail/BIM-298736
American Medical Association (AMA)
Abd al-Qadir, Howida Ali& Hamzah, Muhammad Hasan. Breast cancer diagnosis using artificial intelligence neural networks. Journal of Science and Technology. 2011. Vol. 12, no. 1, pp.159-171.
https://search.emarefa.net/detail/BIM-298736
Data Type
Journal Articles
Language
English
Notes
Includes bibliographical references : p. 170-171
Record ID
BIM-298736