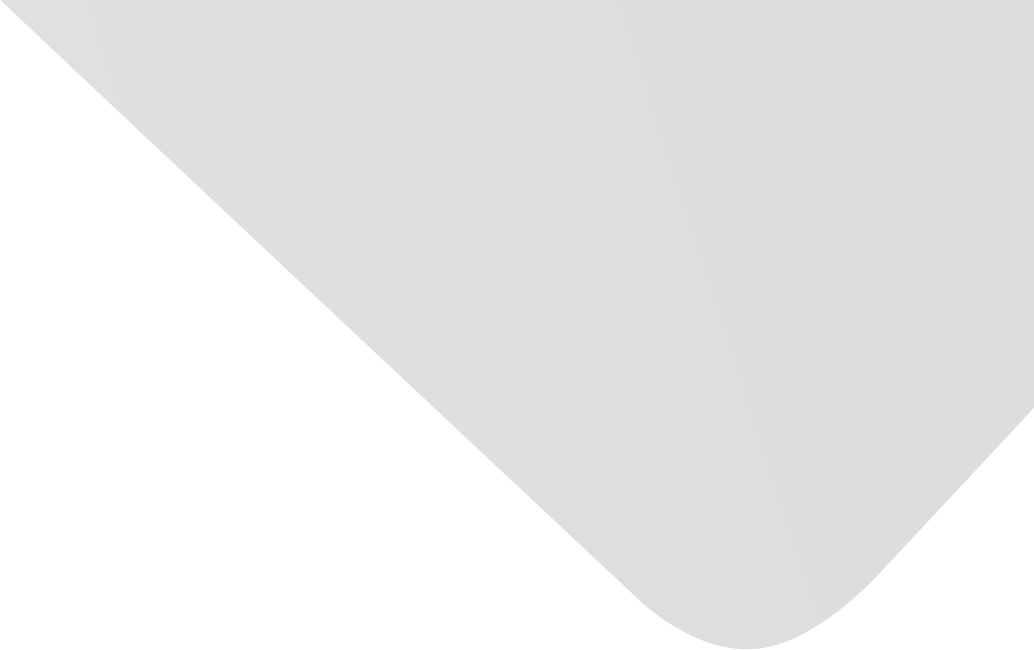
Groundwater salinity modeling using artificial neural networks : Gaza strip case study
Dissertant
Thesis advisor
University
Islamic University
Faculty
Faculty of Engineering
Department
Department of Civil Engineering
University Country
Palestine (Gaza Strip)
Degree
Master
Degree Date
2009
English Abstract
The main source of water in Gaza Strip is the shallow aquifer which is part of the coastal aquifer.
The quality of the groundwater is extremely deteriorated in terms of salinity.
Salinization of groundwater may be caused and influenced by many variables.
Studying the relation of between these variables and salinity is often a complex and nonlinear process, making it suitable for Artificial Neural Networks (ANN) application.
In order to model groundwater salinity in Gaza Strip using ANN it is necessary to gather data for training purposes.
Initially, it is assumed that the groundwater salinity (represented by chloride concentration, mg / l) may be affected by some variables as : recharge rate (R), abstraction (Q), abstraction average rate (Qr), life time (Lt), groundwater level Wl, aquifer thickness (Th), depth from surface to well screen (Dw), and distance from sea shore line (Ds).
Data were extracted from 56 wells, most of them are municipal wells and they almost cover the total area of Gaza Strip.
The initial modeling trials were made using all input variables and many trials were applied to get best performance model.
From the created ANN models, the importance and effect of each variables was studied and represented, also depending on the results of ANN models some input variables were neglected and new modeling trials are made without using neglected input variables. After a number of trials, the best neural network was determined to be Multilayer Perceptron network (MLP) with four layers: an input layer of 6 neurons, first hidden layer with 10 neurons, second hidden layer with 7 neurons and the output layer with 1 neuron.
The six input neurons are: initial chloride concentration (Clo), recharge rate (R), abstraction (Q), abstraction average rate of area (Qr), life time (Lt), aquifer thickness (Th).
The output neuron gives the final chloride concentration (Clf).
The ANN model generated very good results depending on the high correlation between the observed and simulated values of chloride concentration.
The correlation coefficient (r) was 0.9848.
The high value of (r) showed that the simulated chloride concentration values using the ANN model were in very good agreement with the observed chloride concentration which mean that ANN model is useful and applicable for groundwater salinity modeling.
ANN model was successfully utilized as analytical tool to study influence of the input variables on chloride concentration.
It proved that chloride concentration in groundwater is directly affected by abstraction (Q), abstraction average rate (Qr) and life time (Lt).
Furthermore, it was adversely affected by recharge rate (R) and aquifer thickness (Th).
Furthermore, it is utilized as simulation and prediction tool of chloride concentration in domestic wells in Gaza Strip, the prediction of chloride concentration will be based on some scenarios of abstraction from groundwater.
Also it will be used as a decision making support tool that suggests the appropriate abstraction from groundwater wells comparing with the status of salinity.
Main Subjects
Earth Sciences, Water and Environment
Topics
No. of Pages
138
Table of Contents
Tables of contents.
Abstract.
Chapter One : introduction.
Chapter Two : literature review / groundwater salinity.
Chapter Three : literature review / artificial neural networks.
Chapter Foure : study area description.
Chapter Five : methodology.
Chapter Six : results and discussions.
Chapter Seven : conclusions and recommendations.
References.
American Psychological Association (APA)
Siyam, Muhammad. (2009). Groundwater salinity modeling using artificial neural networks : Gaza strip case study. (Master's theses Theses and Dissertations Master). Islamic University, Palestine (Gaza Strip)
https://search.emarefa.net/detail/BIM-300606
Modern Language Association (MLA)
Siyam, Muhammad. Groundwater salinity modeling using artificial neural networks : Gaza strip case study. (Master's theses Theses and Dissertations Master). Islamic University. (2009).
https://search.emarefa.net/detail/BIM-300606
American Medical Association (AMA)
Siyam, Muhammad. (2009). Groundwater salinity modeling using artificial neural networks : Gaza strip case study. (Master's theses Theses and Dissertations Master). Islamic University, Palestine (Gaza Strip)
https://search.emarefa.net/detail/BIM-300606
Language
English
Data Type
Arab Theses
Record ID
BIM-300606