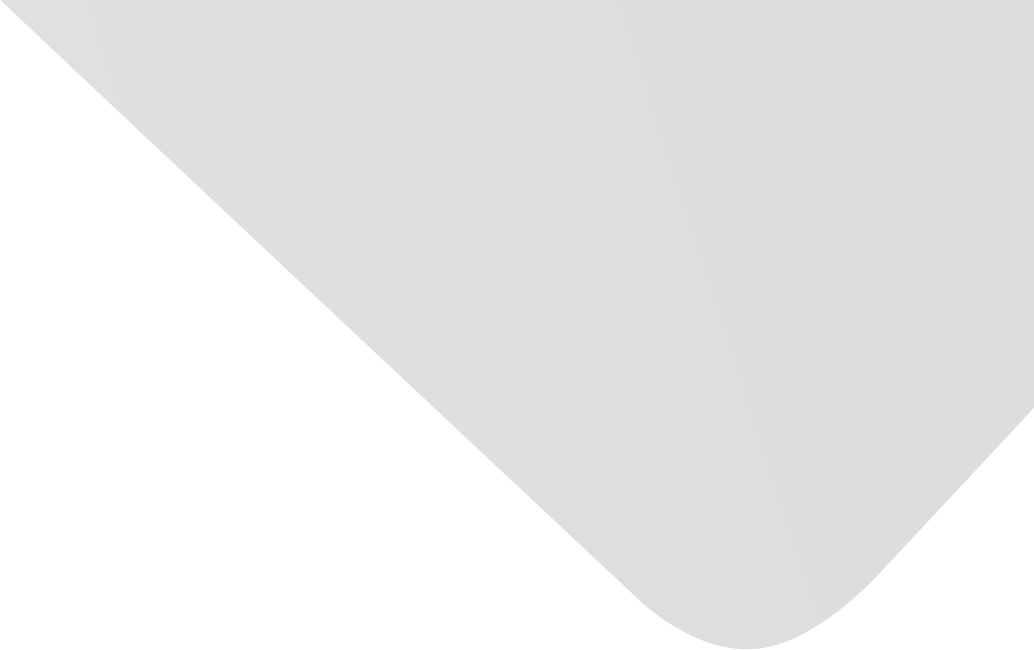
RBF neural network approach for identification and control of DC motors
Other Title(s)
استخدام الشبكات العصبية ذوات الاقترانات الشعاعية للتمييز و التحكم بالمحركات الكهربائية ذات التيار المباشر
Joint Authors
Source
The Journal of Engineering Research
Issue
Vol. 9, Issue 2 (30 Jun. 2012), pp.80-89, 10 p.
Publisher
Sultan Qaboos University College of Engineering
Publication Date
2012-06-30
Country of Publication
Oman
No. of Pages
10
Main Subjects
Topics
Abstract AR
يقدم هذا البحث استخدام الشبكات العصبية في تمييز المحركات الكهربائية ذات التيار المباشر و التحكم بها.
تم بناء شبكتين عصبيتين واحدة لتمييز المحرك و التعرف على أدائه الديناميكي، و شبكة أخرى للتحكم بسرعته و جعلها موافقة لإشارة مرجعية مختارة.
و قد تم بناء نموذجين للتحكم، أحداهما النموذج الانقلابي المباشر و الأخر النموذج الداخلي.
تم بحث أداء الشبكتين العصبيتين باستخدام الاستجابة الخطوية، و التغيير المفاجئ لمسار السرعة، و التغيير المفاجئ للحمل و تغيير ثوابت المحرك.
و قد تمت مقارنة أداء الشبكتين العصبيتين المقترحتين مع أداء شبكة عصبية مرجعية ذات انتشار مرتد، و بينت نتائج البحث أن المحكمات الشبكية تميزت بأداء ديناميكي متميز و متكيف مع التغير المفاجئ لمسار السرعة و التحمل كما تتكيف مع التغير في معاملات المحرك، و كذلك بينت الدراسة أن أداء المحكمين الشبكيين الانقلابي و النموذج الداخلي متماثلين.
Abstract EN
In this paper, a neural network approach for the identification and control of a separately excited direct (DC) motor (SEDCM) driving a centrifugal pump load is applied.
In this application, two radial basis function neural networks (RBFNN) are used : the first is a RBFNN identifier trained offline to emulate the dynamic performance of the DC motor-load system.
The second is a RBFNN controller, which is trained to make the motor speed follow a selected reference signal.
Two RBFNN control schemes are proposed using direct inverse and internal model control schemes.
The performance of the RBFNN identifier and controller is investigated in terms of step response, sharp changes in speed trajectory, and sudden load change, as well as changes in motor parameters.
The performance of RBFNN in system identification and control has been compared with the performance of the well-known back-propagation neural network (BPNN).
The simulation results show that both of the BPNN and RBFNN controllers exhibit excellent dynamic response, adapt well to changes in speed trajectory and load connected to the motor, and adapt to the variations of motor parameters.
Furthermore, the simulation results show that the step response of RBFNN internal model and direct inverse controllers are identical.
American Psychological Association (APA)
Feilat, E. A.& Maayitah, E. K.. 2012. RBF neural network approach for identification and control of DC motors. The Journal of Engineering Research،Vol. 9, no. 2, pp.80-89.
https://search.emarefa.net/detail/BIM-307562
Modern Language Association (MLA)
Feilat, E. A.& Maayitah, E. K.. RBF neural network approach for identification and control of DC motors. The Journal of Engineering Research Vol. 9, no. 2 (Jun. 2012), pp.80-89.
https://search.emarefa.net/detail/BIM-307562
American Medical Association (AMA)
Feilat, E. A.& Maayitah, E. K.. RBF neural network approach for identification and control of DC motors. The Journal of Engineering Research. 2012. Vol. 9, no. 2, pp.80-89.
https://search.emarefa.net/detail/BIM-307562
Data Type
Journal Articles
Language
English
Notes
Includes bibliographical references : p. 89
Record ID
BIM-307562