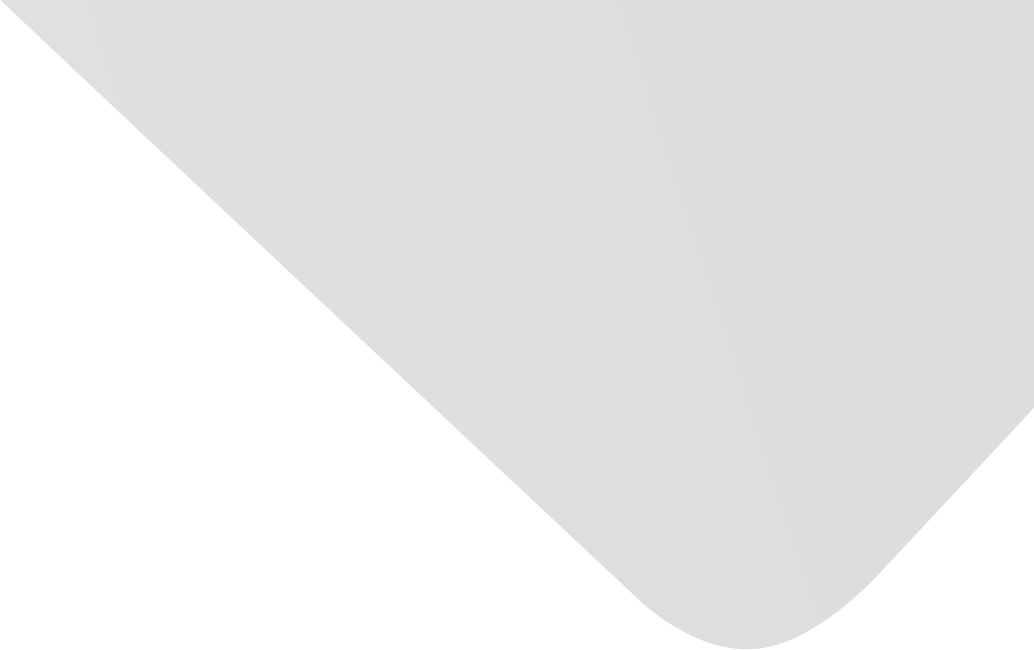
Detection of static air-gap eccentricity in three phase induction motor by using artificial neural network (ANN)
Joint Authors
al-Sabbagh, Qays S.
Ulwan, Haydar E.
Source
Issue
Vol. 15, Issue 4 (31 Dec. 2009), pp.4176-4192, 17 p.
Publisher
University of Baghdad College of Engineering
Publication Date
2009-12-31
Country of Publication
Iraq
No. of Pages
17
Main Subjects
Topics
Abstract AR
تم استخدام تقنية هذا البحث يوضح تأثير اللامركزية الساكنة في الفجوة الهوائية على أداء محرك حثي ثلاثي أطوار الشبكة العصبية الاصطناعية لكشف هذا العطل أن هذه التقنية تعتمد على سعة التوافقيات السالبة و الموجبة للترددات.
في هذا البحث تم استخدام محركين اثنين و بقدرة (2.2 كيلو واط) لتحقيق العطل بصورة حقيقية و للحصول على البيانات المطلوبة و لثلاث اختبارات (اللاحمة نصف الحمل و الحمل الكامل).
تم اعتماد بصمة التيار الساكن لغرض التحليل و الكشف عن وجود اللامركزية.
تم اعتماد الشبكة العصبية ذات التغذية أمامية و تصحيح أخطاء بطريقة إرجاع العكسي أن سعة التوافقيات السالبة و الموجبة للترددات تم اعتمادها لتكون بيانات إدخال لتدريب الشبكة اصطناعية لغرض الكشف و التمييز بين المحرك العاطل و المحرك الخالي من العطل.
Abstract EN
This paper presents the effect of the static air-gap eccentricity on the performance of a three phase induction motor .The Artificial Neural Network (ANN) approach has been used to detect this fault .This technique depends upon the amplitude of the positive and negative harmonics of the frequency.
Two motors of (2.2 kW) have been used to achieve the actual fault and desirable data at no-load, half-load and full-load conditions.
Motor Current Signature analysis (MCSA) based on stator current has been used to detect eccentricity fault.
Feed forward neural network and error back propagation training algorithms are used to perform the motor fault detection.
The inputs of artificial neural network are the amplitudes of the positive and negative harmonics and the speed, and the output is the type of fault.
The training of neural network is achieved by data through the experiments test on healthy and faulty motor and the diagnostic system can discriminate between “healthy” and “faulty” machine.
American Psychological Association (APA)
al-Sabbagh, Qays S.& Ulwan, Haydar E.. 2009. Detection of static air-gap eccentricity in three phase induction motor by using artificial neural network (ANN). Journal of Engineering،Vol. 15, no. 4, pp.4176-4192.
https://search.emarefa.net/detail/BIM-327131
Modern Language Association (MLA)
al-Sabbagh, Qays S.& Ulwan, Haydar E.. Detection of static air-gap eccentricity in three phase induction motor by using artificial neural network (ANN). Journal of Engineering Vol. 15, no. 4 (Dec. 2009), pp.4176-4192.
https://search.emarefa.net/detail/BIM-327131
American Medical Association (AMA)
al-Sabbagh, Qays S.& Ulwan, Haydar E.. Detection of static air-gap eccentricity in three phase induction motor by using artificial neural network (ANN). Journal of Engineering. 2009. Vol. 15, no. 4, pp.4176-4192.
https://search.emarefa.net/detail/BIM-327131
Data Type
Journal Articles
Language
English
Notes
Includes appendixes : p. 4192
Record ID
BIM-327131