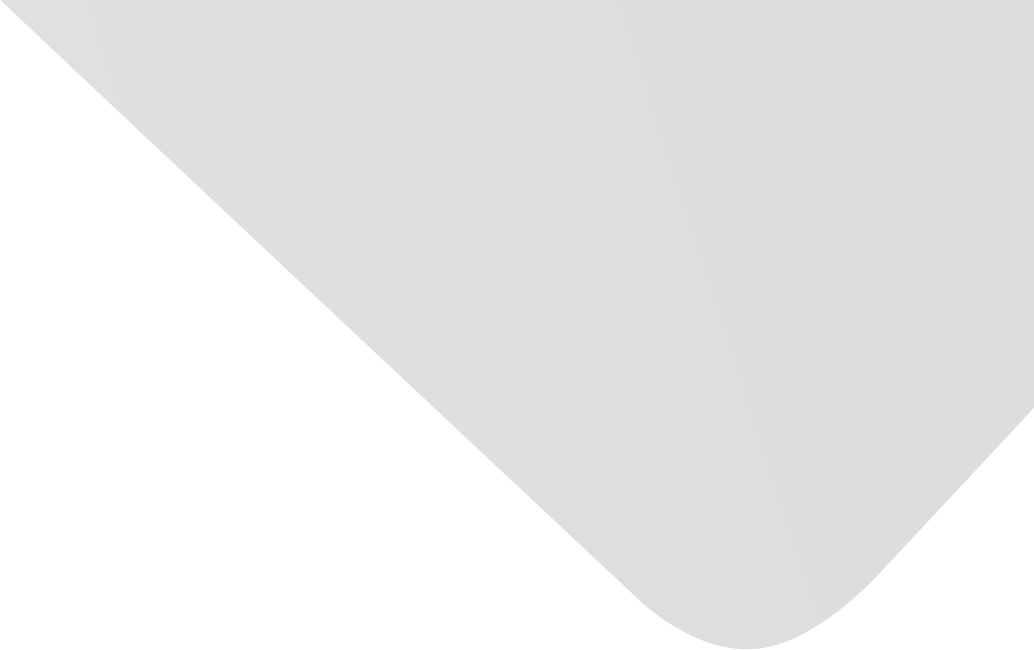
Worm detection by combination of classification with neural networks
Joint Authors
Source
International Arab Journal of E-Technology
Issue
Vol. 3, Issue 2 (30 Jun. 2013), pp.107-113, 7 p.
Publisher
Publication Date
2013-06-30
Country of Publication
Jordan
No. of Pages
7
Main Subjects
Topics
Abstract EN
Security has become ubiquitous in every domain today as newly emerging malware pose an ever increasing perilous threat to systems.
Worms are on the top of malware threats attacking computer system although of the evolution of worm’s detection techniques.
Early detection of unknown worms is still a problem.
In this paper, we proposed and implemented a new approach for worm’s detection.
The proposed model uses combination of Decision Tree and Neural Network (NN) as classifying worm/non worm traffic network.
Our results showed that the detection rates of classification and detection known worms are at least 93.51 % with NN, and 92.87 % with Decision Tree, while the unknown worm detection rates was about 97.27 % with NN, and 93.2 % with Decision Tree.
The detection rate of our proposed model in known worm was 95.59% while the unknown worms detection rate was 97.74 %.
American Psychological Association (APA)
Barhum, Tawfiq& Qishtah, Hana. 2013. Worm detection by combination of classification with neural networks. International Arab Journal of E-Technology،Vol. 3, no. 2, pp.107-113.
https://search.emarefa.net/detail/BIM-340379
Modern Language Association (MLA)
Barhum, Tawfiq& Qishtah, Hana. Worm detection by combination of classification with neural networks. International Arab Journal of E-Technology Vol. 3, no. 2 (Jun. 2013), pp.107-113.
https://search.emarefa.net/detail/BIM-340379
American Medical Association (AMA)
Barhum, Tawfiq& Qishtah, Hana. Worm detection by combination of classification with neural networks. International Arab Journal of E-Technology. 2013. Vol. 3, no. 2, pp.107-113.
https://search.emarefa.net/detail/BIM-340379
Data Type
Journal Articles
Language
English
Notes
Includes bibliographical references : p. 112-113
Record ID
BIM-340379