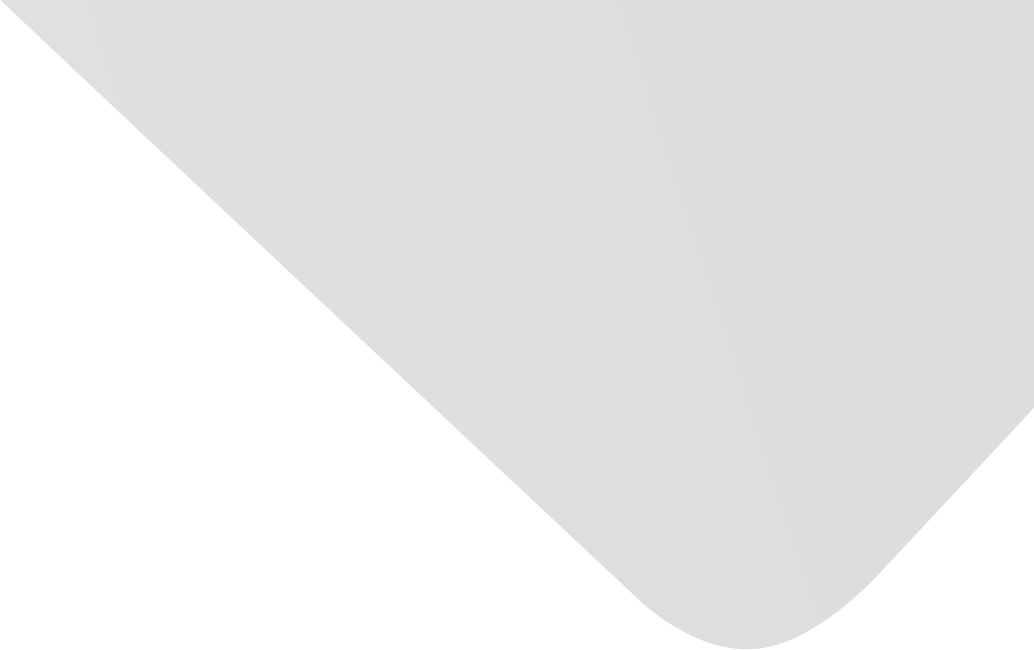
Spatial prediction of monthly precipitation in Sulaimani Governorate using artificial neural network models
Joint Authors
al-Suhaili, Rafi Hashim Shakir
Karim, Rizqar Ahmad
Source
Issue
Vol. 20, Issue 3 (31 Mar. 2014), pp.15-27, 13 p.
Publisher
University of Baghdad College of Engineering
Publication Date
2014-03-31
Country of Publication
Iraq
No. of Pages
13
Main Subjects
Topics
Abstract AR
تم استخدام تقنية نمذجة الشبكات العصبية الصناعية لتخمين بيانات الأمطار الشهرية في أحدى المحطات الهيدرولوجية المناخية من واقع ثمان محطات في شبكة المحطات المناخية في محافظة السليمانية.
تم بناء ثمان نماذج من الشبكات العصبية لكل محطة نموذج.
ثم للحصول على نماذج ذات دقة عالية لتخمين الأمطار الشهرية حيث تراوح معامل الارتباط بين الأمطار الشهرية المخمنة و تلك المقاسة من (90% - 97,2%).
كل نموذج تم إيجاده بعد محاولات عديدة لكل محطة و تم اختيار النموذج الذي يعطي أعلى معامل ارتباط.
جميع النماذج للشبكات العصبية الصناعية وجدت ذات دالة تفعيل نوع (hyperbolic tangent) و (identity) للطبقة المخفية و طبقة المخرجات على التوالي، و بمعدل تعلم للشبكة (0,4) و معامل زخم (0,9) و لكن بمختلف أنواع تقسيم البيانات إلى بيانات التدريب، الاختبار و التخمين و مختلف الأعداد للعقد في الطبقة المخفية.
كما وجد في تحليل التأثير القياسي بأنه ليس من الضروري أن تكون المحطة ذات المسافة الأقرب من المحطة تحت التخمين ذات أعلى تأثير على الأمطار الشهرية لتلك المحطة و ذلك بسبب الفروقات العالية بين منسوب المحطات.
كما وجد بأنه ليس من الضروري أن تكون المحطات ذات البيانات التي أعطت أعلى تباين أن تكون ذات أقل معامل الارتباط للقيم المخمنة مع تلك المقاسة.
Abstract EN
ANN modeling is used here to predict missing monthly precipitation data in one station of the eight weather stations network in Sulaimani Governorate.
Eight models were developed, one for each station as for prediction.
The accuracy of prediction obtain is excellent with correlation coefficients between the predicted and the measured values of monthly precipitation ranged from (90% to 97.2%).
The eight ANN models are found after many trials for each station and those with the highest correlation coefficient were selected.
All the ANN models are found to have a hyperbolic tangent and identity activation functions for the hidden and output layers respectively, with learning rate of (0.4) and momentum term of (0.9), but with different data set sub-division into training, testing and holdout data sub-sets, and different number of hidden nodes in the hidden layer.
It is found that it is not necessary that the nearest station to the station under prediction has the highest effect; this may be attributed to the high differences in elevation between the stations.
It can also found that the variance is not necessary has effect on the correlation coefficient obtained.
American Psychological Association (APA)
al-Suhaili, Rafi Hashim Shakir& Karim, Rizqar Ahmad. 2014. Spatial prediction of monthly precipitation in Sulaimani Governorate using artificial neural network models. Journal of Engineering،Vol. 20, no. 3, pp.15-27.
https://search.emarefa.net/detail/BIM-376864
Modern Language Association (MLA)
al-Suhaili, Rafi Hashim Shakir& Karim, Rizqar Ahmad. Spatial prediction of monthly precipitation in Sulaimani Governorate using artificial neural network models. Journal of Engineering Vol. 20, no. 3 (Mar. 2014), pp.15-27.
https://search.emarefa.net/detail/BIM-376864
American Medical Association (AMA)
al-Suhaili, Rafi Hashim Shakir& Karim, Rizqar Ahmad. Spatial prediction of monthly precipitation in Sulaimani Governorate using artificial neural network models. Journal of Engineering. 2014. Vol. 20, no. 3, pp.15-27.
https://search.emarefa.net/detail/BIM-376864
Data Type
Journal Articles
Language
English
Notes
Includes appendices : p. 20-27
Record ID
BIM-376864