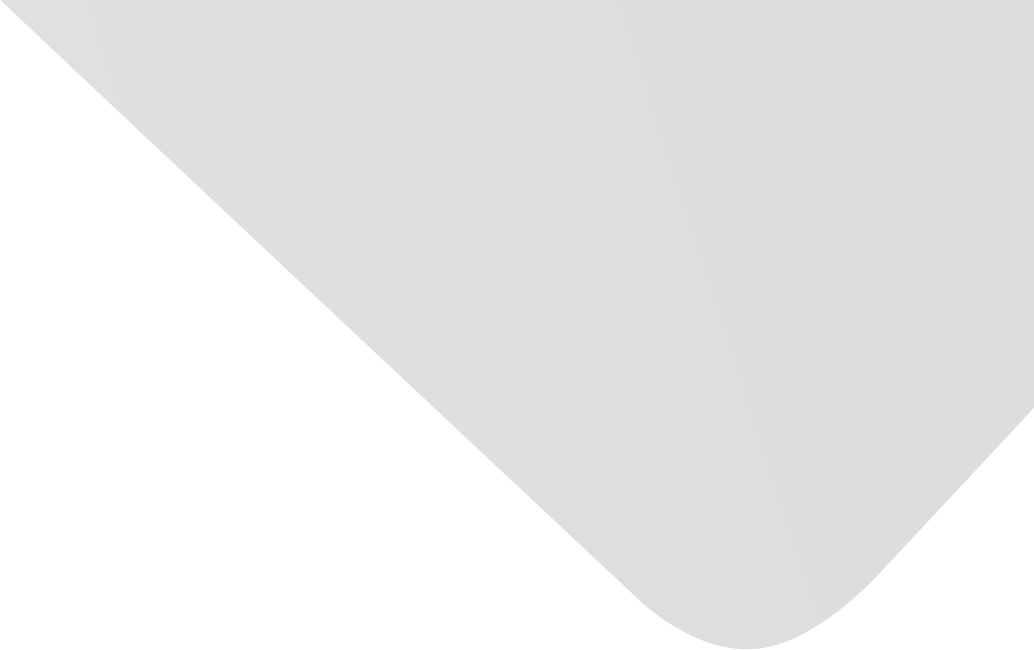
Kernel logistic regression algorithm for large-scale data classification
Joint Authors
Wang, Jianxin
al-Bashir, Murtada
Source
The International Arab Journal of Information Technology
Issue
Vol. 12, Issue 5 (30 Sep. 2015)8 p.
Publisher
Publication Date
2015-09-30
Country of Publication
Jordan
No. of Pages
8
Main Subjects
Abstract EN
Kernel Logistic Regression (KLR) is a powerful classification technique that has been applied successfully in many classification problems.
However, it is often not found in large-scale data classification problems, and this is mainly because it is computationally expensive.
In this paper, we present a new KLR algorithm based on Truncated Regularized Iteratively Re-Weighted Least Squares(TR-IRLS) algorithm to obtain sparse large-scale data classification in short evolution time.
This new algorithm is called Nystrom Truncated Kernel Logistic Regression (NTR-KLR).
The performance achieved using NTR-KLR algorithm is comparable to that of Support Vector Machines (SVMs) methods.
The advantage is NTR-KLR can yield probabilistic outputs, and its extension to the multi-class case is well-defined.
In addition, its computational complexity is lower than that of SVMs methods, and it is easy to implement.
American Psychological Association (APA)
al-Bashir, Murtada& Wang, Jianxin. 2015. Kernel logistic regression algorithm for large-scale data classification. The International Arab Journal of Information Technology،Vol. 12, no. 5.
https://search.emarefa.net/detail/BIM-430970
Modern Language Association (MLA)
al-Bashir, Murtada& Wang, Jianxin. Kernel logistic regression algorithm for large-scale data classification. The International Arab Journal of Information Technology Vol. 12, no. 5 (Sep. 2015).
https://search.emarefa.net/detail/BIM-430970
American Medical Association (AMA)
al-Bashir, Murtada& Wang, Jianxin. Kernel logistic regression algorithm for large-scale data classification. The International Arab Journal of Information Technology. 2015. Vol. 12, no. 5.
https://search.emarefa.net/detail/BIM-430970
Data Type
Journal Articles
Language
English
Notes
Includes bibliographical references
Record ID
BIM-430970