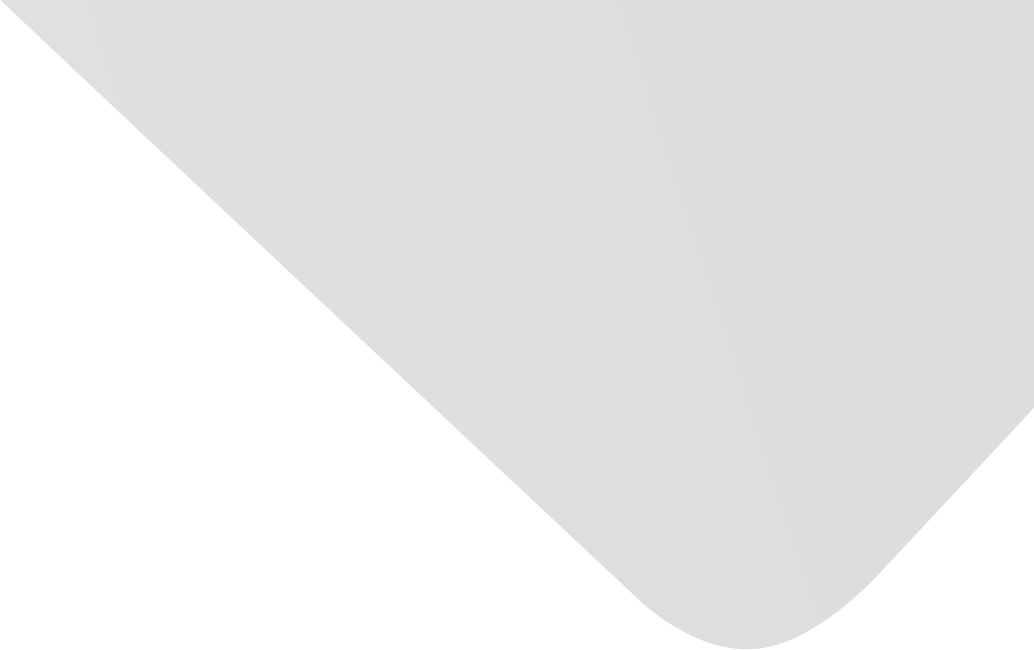
PleasantUnpleasant Filtering for Affective Image Retrieval Based on Cross-Correlation of EEG Features
Joint Authors
Kusumoto, Yusuke
Xielifuguli, Keranmu
Matsumoto, Kazuyuki
Fujisawa, Akira
Kita, Kenji
Source
Applied Computational Intelligence and Soft Computing
Issue
Vol. 2014, Issue 2014 (31 Dec. 2014), pp.1-10, 10 p.
Publisher
Hindawi Publishing Corporation
Publication Date
2014-03-05
Country of Publication
Egypt
No. of Pages
10
Main Subjects
Information Technology and Computer Science
Abstract EN
People often make decisions based on sensitivity rather than rationality.
In the field of biological information processing, methods are available for analyzing biological information directly based on electroencephalogram: EEG to determine the pleasant/unpleasant reactions of users.
In this study, we propose a sensitivity filtering technique for discriminating preferences (pleasant/unpleasant) for images using a sensitivity image filtering system based on EEG.
Using a set of images retrieved by similarity retrieval, we perform the sensitivity-based pleasant/unpleasant classification of images based on the affective features extracted from images with the maximum entropy method: MEM.
In the present study, the affective features comprised cross-correlation features obtained from EEGs produced when an individual observed an image.
However, it is difficult to measure the EEG when a subject visualizes an unknown image.
Thus, we propose a solution where a linear regression method based on canonical correlation is used to estimate the cross-correlation features from image features.
Experiments were conducted to evaluate the validity of sensitivity filtering compared with image similarity retrieval methods based on image features.
We found that sensitivity filtering using color correlograms was suitable for the classification of preferred images, while sensitivity filtering using local binary patterns was suitable for the classification of unpleasant images.
Moreover, sensitivity filtering using local binary patterns for unpleasant images had a 90% success rate.
Thus, we conclude that the proposed method is efficient for filtering unpleasant images.
American Psychological Association (APA)
Xielifuguli, Keranmu& Fujisawa, Akira& Kusumoto, Yusuke& Matsumoto, Kazuyuki& Kita, Kenji. 2014. PleasantUnpleasant Filtering for Affective Image Retrieval Based on Cross-Correlation of EEG Features. Applied Computational Intelligence and Soft Computing،Vol. 2014, no. 2014, pp.1-10.
https://search.emarefa.net/detail/BIM-470325
Modern Language Association (MLA)
Xielifuguli, Keranmu…[et al.]. PleasantUnpleasant Filtering for Affective Image Retrieval Based on Cross-Correlation of EEG Features. Applied Computational Intelligence and Soft Computing No. 2014 (2014), pp.1-10.
https://search.emarefa.net/detail/BIM-470325
American Medical Association (AMA)
Xielifuguli, Keranmu& Fujisawa, Akira& Kusumoto, Yusuke& Matsumoto, Kazuyuki& Kita, Kenji. PleasantUnpleasant Filtering for Affective Image Retrieval Based on Cross-Correlation of EEG Features. Applied Computational Intelligence and Soft Computing. 2014. Vol. 2014, no. 2014, pp.1-10.
https://search.emarefa.net/detail/BIM-470325
Data Type
Journal Articles
Language
English
Notes
Includes bibliographical references
Record ID
BIM-470325