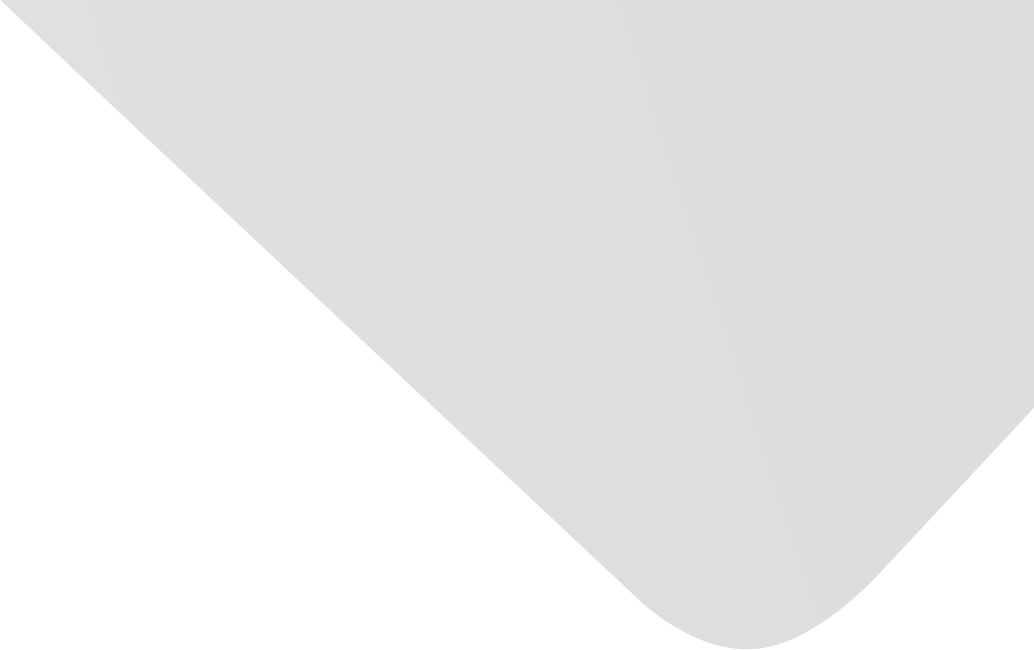
Sparse Representation for Tumor Classification Based on Feature Extraction Using Latent Low-Rank Representation
Joint Authors
Zheng, Chun-Hou
Gan, Bin
Zhang, Jun
Wang, Hong-Qiang
Source
Issue
Vol. 2014, Issue 2014 (31 Dec. 2014), pp.1-7, 7 p.
Publisher
Hindawi Publishing Corporation
Publication Date
2014-02-11
Country of Publication
Egypt
No. of Pages
7
Main Subjects
Abstract EN
Accurate tumor classification is crucial to the proper treatment of cancer.
To now, sparse representation (SR) has shown its great performance for tumor classification.
This paper conceives a new SR-based method for tumor classification by using gene expression data.
In the proposed method, we firstly use latent low-rank representation for extracting salient features and removing noise from the original samples data.
Then we use sparse representation classifier (SRC) to build tumor classification model.
The experimental results on several real-world data sets show that our method is more efficient and more effective than the previous classification methods including SVM, SRC, and LASSO.
American Psychological Association (APA)
Gan, Bin& Zheng, Chun-Hou& Zhang, Jun& Wang, Hong-Qiang. 2014. Sparse Representation for Tumor Classification Based on Feature Extraction Using Latent Low-Rank Representation. BioMed Research International،Vol. 2014, no. 2014, pp.1-7.
https://search.emarefa.net/detail/BIM-470865
Modern Language Association (MLA)
Gan, Bin…[et al.]. Sparse Representation for Tumor Classification Based on Feature Extraction Using Latent Low-Rank Representation. BioMed Research International No. 2014 (2014), pp.1-7.
https://search.emarefa.net/detail/BIM-470865
American Medical Association (AMA)
Gan, Bin& Zheng, Chun-Hou& Zhang, Jun& Wang, Hong-Qiang. Sparse Representation for Tumor Classification Based on Feature Extraction Using Latent Low-Rank Representation. BioMed Research International. 2014. Vol. 2014, no. 2014, pp.1-7.
https://search.emarefa.net/detail/BIM-470865
Data Type
Journal Articles
Language
English
Notes
Includes bibliographical references
Record ID
BIM-470865