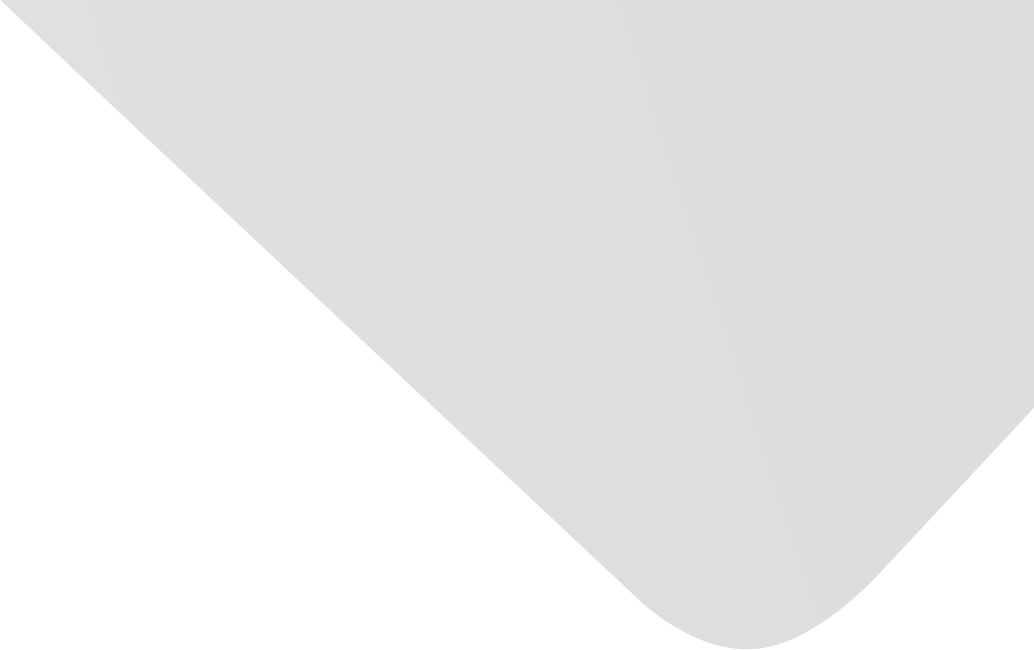
Multiple Kernel Spectral Regression for Dimensionality Reduction
Joint Authors
Xia, Shixiong
Zhou, Yong
Liu, Bing
Source
Journal of Applied Mathematics
Issue
Vol. 2013, Issue 2013 (31 Dec. 2013), pp.1-8, 8 p.
Publisher
Hindawi Publishing Corporation
Publication Date
2013-10-23
Country of Publication
Egypt
No. of Pages
8
Main Subjects
Abstract EN
Traditional manifold learning algorithms, such as locally linear embedding, Isomap, and Laplacian eigenmap, only provide the embedding results of the training samples.
To solve the out-of-sample extension problem, spectral regression (SR) solves the problem of learning an embedding function by establishing a regression framework, which can avoid eigen-decomposition of dense matrices.
Motivated by the effectiveness of SR, we incorporate multiple kernel learning (MKL) into SR for dimensionality reduction.
The proposed approach (termed MKL-SR) seeks an embedding function in the Reproducing Kernel Hilbert Space (RKHS) induced by the multiple base kernels.
An MKL-SR algorithm is proposed to improve the performance of kernel-based SR (KSR) further.
Furthermore, the proposed MKL-SR algorithm can be performed in the supervised, unsupervised, and semi-supervised situation.
Experimental results on supervised classification and semi-supervised classification demonstrate the effectiveness and efficiency of our algorithm.
American Psychological Association (APA)
Liu, Bing& Xia, Shixiong& Zhou, Yong. 2013. Multiple Kernel Spectral Regression for Dimensionality Reduction. Journal of Applied Mathematics،Vol. 2013, no. 2013, pp.1-8.
https://search.emarefa.net/detail/BIM-471384
Modern Language Association (MLA)
Liu, Bing…[et al.]. Multiple Kernel Spectral Regression for Dimensionality Reduction. Journal of Applied Mathematics No. 2013 (2013), pp.1-8.
https://search.emarefa.net/detail/BIM-471384
American Medical Association (AMA)
Liu, Bing& Xia, Shixiong& Zhou, Yong. Multiple Kernel Spectral Regression for Dimensionality Reduction. Journal of Applied Mathematics. 2013. Vol. 2013, no. 2013, pp.1-8.
https://search.emarefa.net/detail/BIM-471384
Data Type
Journal Articles
Language
English
Notes
Includes bibliographical references
Record ID
BIM-471384