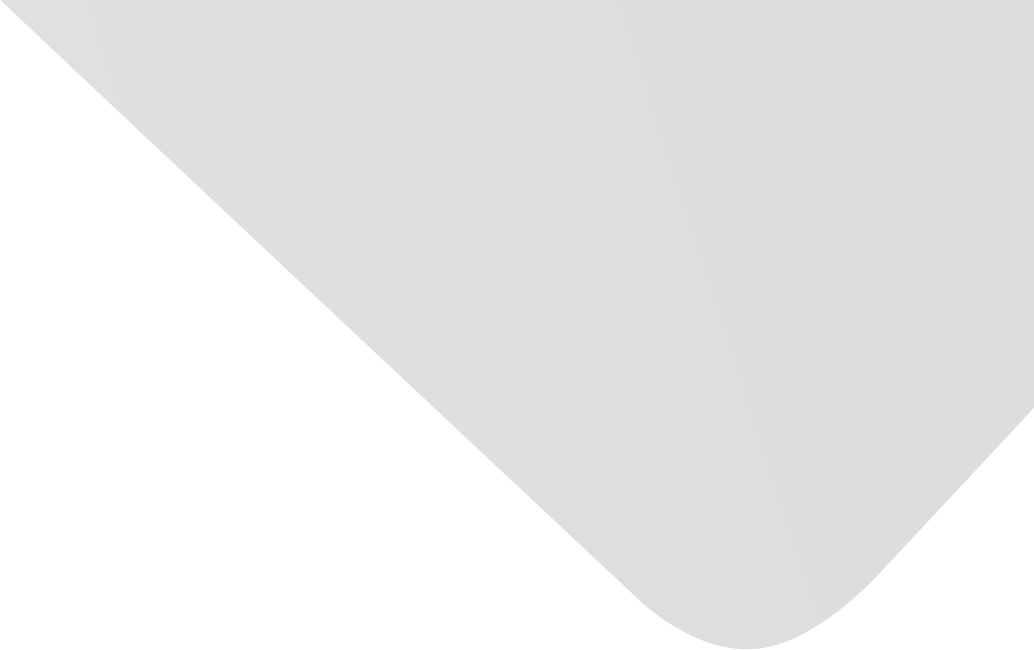
Robust Reservoir Generation by Correlation-Based Learning
Joint Authors
Tanaka, Shigeru
Yamazaki, Tadashi
Source
Advances in Artificial Neural Systems
Issue
Vol. 2009, Issue 2009 (31 Dec. 2009), pp.1-7, 7 p.
Publisher
Hindawi Publishing Corporation
Publication Date
2008-10-27
Country of Publication
Egypt
No. of Pages
7
Main Subjects
Information Technology and Computer Science
Abstract EN
Reservoir computing (RC) is a new framework for neural computation.
A reservoir is usually a recurrent neural network with fixed random connections.
In this article, we propose an RC model in which the connections in the reservoir are modifiable.
Specifically, we consider correlation-based learning (CBL), which modifies the connection weight between a given pair of neurons according to the correlation in their activities.
We demonstrate that CBL enables the reservoir to reproduce almost the same spatiotemporal activity patterns in response to an identical input stimulus in the presence of noise.
This result suggests that CBL enhances the robustness in the generation of the spatiotemporal activity pattern against noise in input signals.
We apply our RC model to trace eyeblink conditioning.
The reservoir bridged the gap of an interstimulus interval between the conditioned and unconditioned stimuli, and a readout neuron was able to learn and express the timed conditioned response.
American Psychological Association (APA)
Yamazaki, Tadashi& Tanaka, Shigeru. 2008. Robust Reservoir Generation by Correlation-Based Learning. Advances in Artificial Neural Systems،Vol. 2009, no. 2009, pp.1-7.
https://search.emarefa.net/detail/BIM-473789
Modern Language Association (MLA)
Yamazaki, Tadashi& Tanaka, Shigeru. Robust Reservoir Generation by Correlation-Based Learning. Advances in Artificial Neural Systems No. 2009 (2009), pp.1-7.
https://search.emarefa.net/detail/BIM-473789
American Medical Association (AMA)
Yamazaki, Tadashi& Tanaka, Shigeru. Robust Reservoir Generation by Correlation-Based Learning. Advances in Artificial Neural Systems. 2008. Vol. 2009, no. 2009, pp.1-7.
https://search.emarefa.net/detail/BIM-473789
Data Type
Journal Articles
Language
English
Notes
Includes bibliographical references
Record ID
BIM-473789