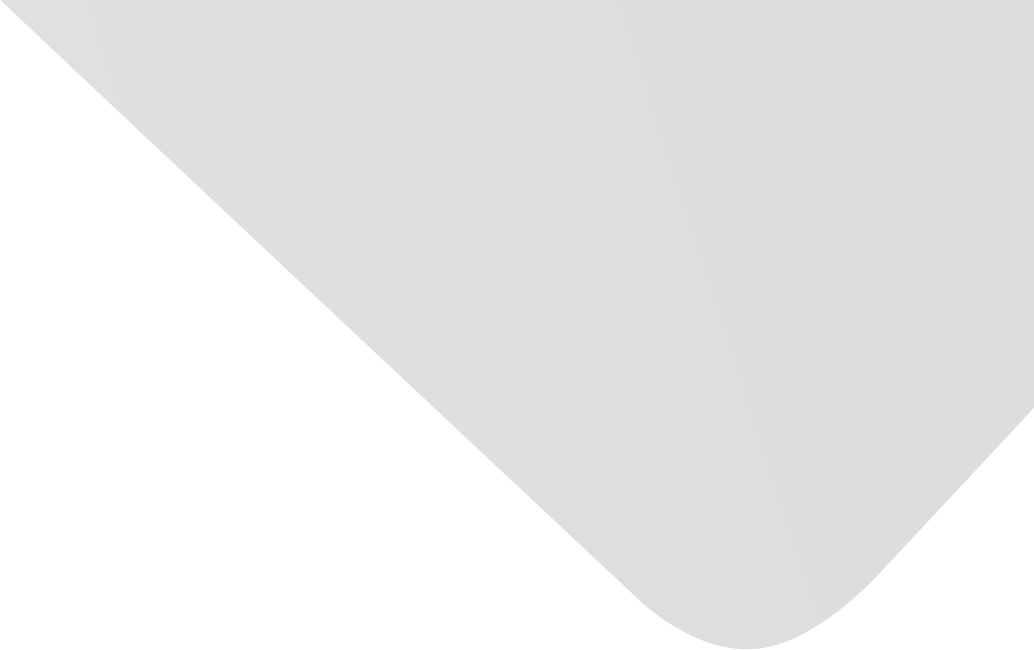
Effectiveness of Partition and Graph Theoretic Clustering Algorithms for Multiple Source Partial Discharge Pattern Classification Using Probabilistic Neural Network and Its Adaptive Version : A Critique Based on Experimental Studies
Joint Authors
Gopal, S.
Venkatesh, S.
Kannan, K.
Source
Journal of Electrical and Computer Engineering
Issue
Vol. 2012, Issue 2012 (31 Dec. 2012), pp.1-19, 19 p.
Publisher
Hindawi Publishing Corporation
Publication Date
2012-10-18
Country of Publication
Egypt
No. of Pages
19
Main Subjects
Engineering Sciences and Information Technology
Information Technology and Computer Science
Abstract EN
Partial discharge (PD) is a major cause of failure of power apparatus and hence its measurement and analysis have emerged as a vital field in assessing the condition of the insulation system.
Several efforts have been undertaken by researchers to classify PD pulses utilizing artificial intelligence techniques.
Recently, the focus has shifted to the identification of multiple sources of PD since it is often encountered in real-time measurements.
Studies have indicated that classification of multi-source PD becomes difficult with the degree of overlap and that several techniques such as mixed Weibull functions, neural networks, and wavelet transformation have been attempted with limited success.
Since digital PD acquisition systems record data for a substantial period, the database becomes large, posing considerable difficulties during classification.
This research work aims firstly at analyzing aspects concerning classification capability during the discrimination of multisource PD patterns.
Secondly, it attempts at extending the previous work of the authors in utilizing the novel approach of probabilistic neural network versions for classifying moderate sets of PD sources to that of large sets.
The third focus is on comparing the ability of partition-based algorithms, namely, the labelled (learning vector quantization) and unlabelled (K-means) versions, with that of a novel hypergraph-based clustering method in providing parsimonious sets of centers during classification.
American Psychological Association (APA)
Venkatesh, S.& Gopal, S.& Kannan, K.. 2012. Effectiveness of Partition and Graph Theoretic Clustering Algorithms for Multiple Source Partial Discharge Pattern Classification Using Probabilistic Neural Network and Its Adaptive Version : A Critique Based on Experimental Studies. Journal of Electrical and Computer Engineering،Vol. 2012, no. 2012, pp.1-19.
https://search.emarefa.net/detail/BIM-474837
Modern Language Association (MLA)
Venkatesh, S.…[et al.]. Effectiveness of Partition and Graph Theoretic Clustering Algorithms for Multiple Source Partial Discharge Pattern Classification Using Probabilistic Neural Network and Its Adaptive Version : A Critique Based on Experimental Studies. Journal of Electrical and Computer Engineering No. 2012 (2012), pp.1-19.
https://search.emarefa.net/detail/BIM-474837
American Medical Association (AMA)
Venkatesh, S.& Gopal, S.& Kannan, K.. Effectiveness of Partition and Graph Theoretic Clustering Algorithms for Multiple Source Partial Discharge Pattern Classification Using Probabilistic Neural Network and Its Adaptive Version : A Critique Based on Experimental Studies. Journal of Electrical and Computer Engineering. 2012. Vol. 2012, no. 2012, pp.1-19.
https://search.emarefa.net/detail/BIM-474837
Data Type
Journal Articles
Language
English
Notes
Includes bibliographical references
Record ID
BIM-474837