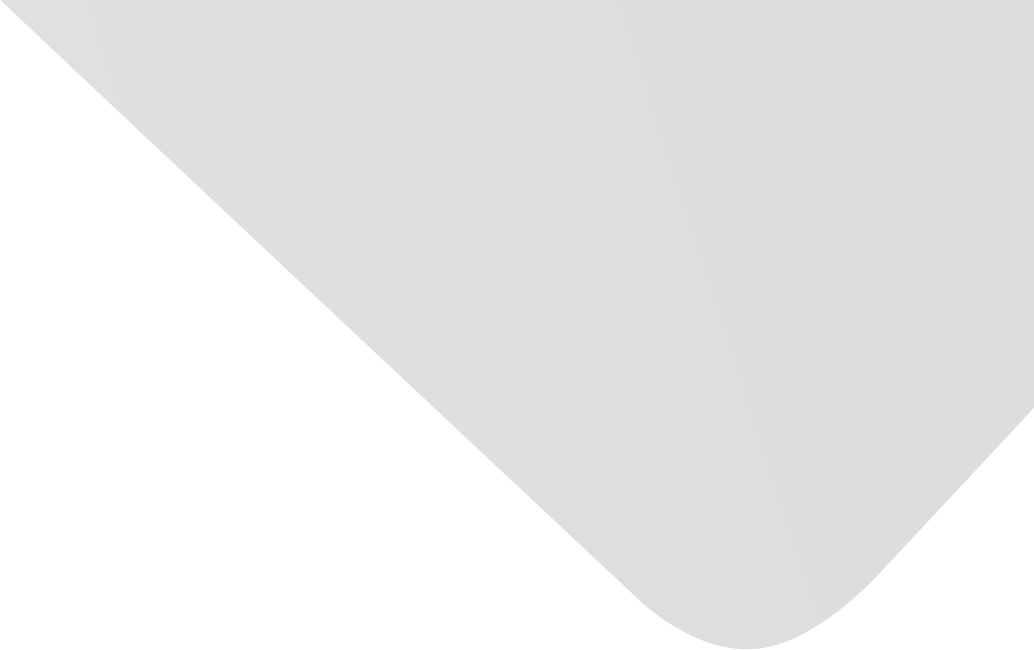
Density Problem and Approximation Error in Learning Theory
Author
Source
Issue
Vol. 2013, Issue 2013 (31 Dec. 2013), pp.1-13, 13 p.
Publisher
Hindawi Publishing Corporation
Publication Date
2013-10-07
Country of Publication
Egypt
No. of Pages
13
Main Subjects
Abstract EN
We study the density problem and approximation error of reproducing kernel Hilbert spaces for the purpose of learning theory.
For a Mercer kernel K on a compact metric space (X, d), a characterization for the generated reproducing kernel Hilbert space (RKHS) ℋK to be dense in C(X) is given.
As a corollary, we show that the density is always true for convolution type kernels.
Some estimates for the rate of convergence of interpolation schemes are presented for general Mercer kernels.
These are then used to establish for convolution type kernels quantitative analysis for the approximation error in learning theory.
Finally, we show by the example of Gaussian kernels with varying variances that the approximation error can be improved when we adaptively change the value of the parameter for the used kernel.
This confirms the method of choosing varying parameters which is used often in many applications of learning theory.
American Psychological Association (APA)
Zhou, Ding-Xuan. 2013. Density Problem and Approximation Error in Learning Theory. Abstract and Applied Analysis،Vol. 2013, no. 2013, pp.1-13.
https://search.emarefa.net/detail/BIM-492824
Modern Language Association (MLA)
Zhou, Ding-Xuan. Density Problem and Approximation Error in Learning Theory. Abstract and Applied Analysis No. 2013 (2013), pp.1-13.
https://search.emarefa.net/detail/BIM-492824
American Medical Association (AMA)
Zhou, Ding-Xuan. Density Problem and Approximation Error in Learning Theory. Abstract and Applied Analysis. 2013. Vol. 2013, no. 2013, pp.1-13.
https://search.emarefa.net/detail/BIM-492824
Data Type
Journal Articles
Language
English
Notes
Includes bibliographical references
Record ID
BIM-492824