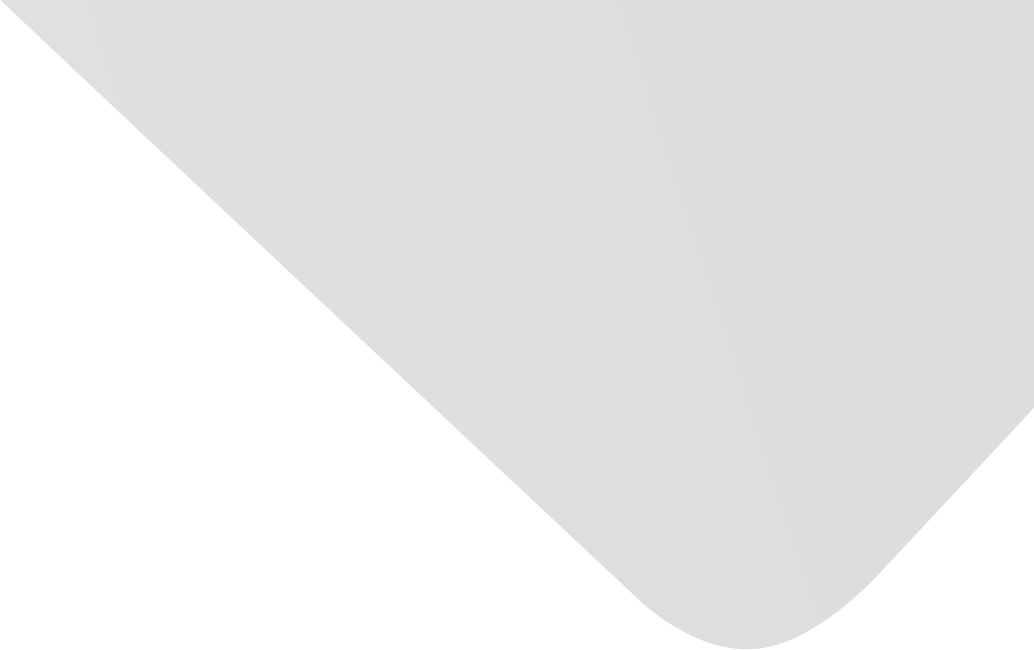
On the Variability of Neural Network Classification Measures in the Protein Secondary Structure Prediction Problem
Joint Authors
Source
Applied Computational Intelligence and Soft Computing
Issue
Vol. 2013, Issue 2013 (31 Dec. 2013), pp.1-9, 9 p.
Publisher
Hindawi Publishing Corporation
Publication Date
2013-01-31
Country of Publication
Egypt
No. of Pages
9
Main Subjects
Information Technology and Computer Science
Abstract EN
We revisit the protein secondary structure prediction problem using linear and backpropagation neural network architectures commonly applied in the literature.
In this context, neural network mappings are constructed between protein training set sequences and their assigned structure classes in order to analyze the class membership of test data and associated measures of significance.
We present numerical results demonstrating that classifier performance measures can vary significantly depending upon the classifier architecture and the structure class encoding technique.
Furthermore, an analytic formulation is introduced in order to substantiate the observed numerical data.
Finally, we analyze and discuss the ability of the neural network to accurately model fundamental attributes of protein secondary structure.
American Psychological Association (APA)
Sakk, Eric& Alexander, Ayanna. 2013. On the Variability of Neural Network Classification Measures in the Protein Secondary Structure Prediction Problem. Applied Computational Intelligence and Soft Computing،Vol. 2013, no. 2013, pp.1-9.
https://search.emarefa.net/detail/BIM-498676
Modern Language Association (MLA)
Sakk, Eric& Alexander, Ayanna. On the Variability of Neural Network Classification Measures in the Protein Secondary Structure Prediction Problem. Applied Computational Intelligence and Soft Computing No. 2013 (2013), pp.1-9.
https://search.emarefa.net/detail/BIM-498676
American Medical Association (AMA)
Sakk, Eric& Alexander, Ayanna. On the Variability of Neural Network Classification Measures in the Protein Secondary Structure Prediction Problem. Applied Computational Intelligence and Soft Computing. 2013. Vol. 2013, no. 2013, pp.1-9.
https://search.emarefa.net/detail/BIM-498676
Data Type
Journal Articles
Language
English
Notes
Includes bibliographical references
Record ID
BIM-498676