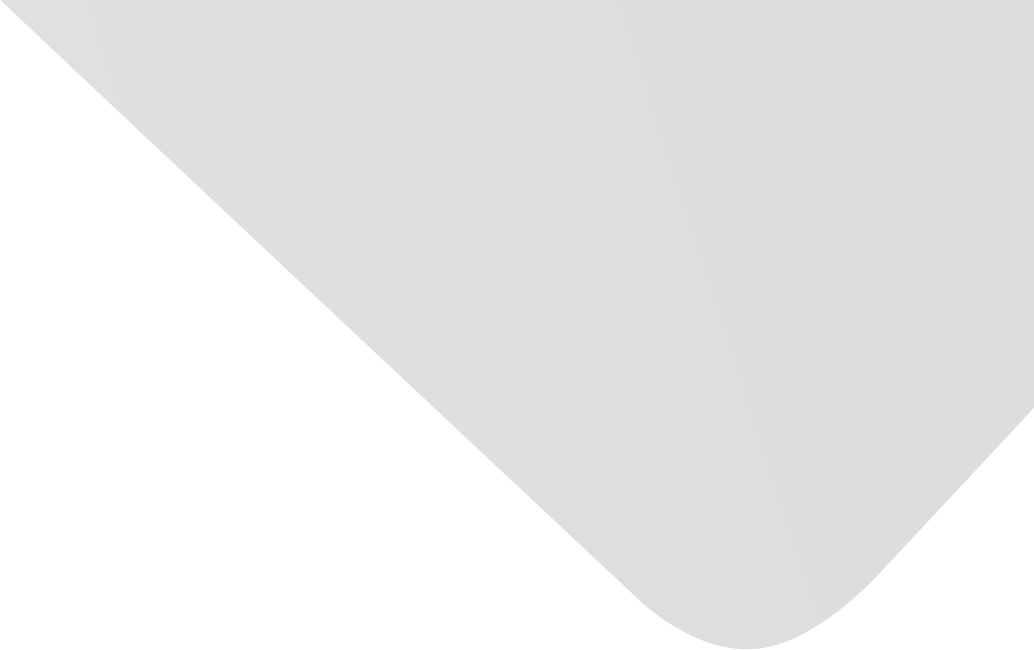
Evolutionary Voting-Based Extreme Learning Machines
Joint Authors
Cao, Jiuwen
Liu, Nan
Pek, Pin Pin
Koh, Zhi Xiong
Lin, Zhiping
Ong, Marcus Eng Hock
Source
Mathematical Problems in Engineering
Issue
Vol. 2014, Issue 2014 (31 Dec. 2014), pp.1-7, 7 p.
Publisher
Hindawi Publishing Corporation
Publication Date
2014-08-14
Country of Publication
Egypt
No. of Pages
7
Main Subjects
Abstract EN
Voting-based extreme learning machine (V-ELM) was proposed to improve learning efficiency where majority voting was employed.
V-ELM assumes that all individual classifiers contribute equally to the decision ensemble.
However, in many real-world scenarios, this assumption does not work well.
In this paper, we aim to enhance V-ELM by introducing weights to distinguish the importance of each individual ELM classifier in decision making.
Genetic algorithm is used for optimizing these weights.
This evolutionary V-ELM is named as EV-ELM.
Results on several benchmark databases show that EV-ELM achieves the highest classification accuracy compared with V-ELM and ELM.
American Psychological Association (APA)
Liu, Nan& Cao, Jiuwen& Lin, Zhiping& Pek, Pin Pin& Koh, Zhi Xiong& Ong, Marcus Eng Hock. 2014. Evolutionary Voting-Based Extreme Learning Machines. Mathematical Problems in Engineering،Vol. 2014, no. 2014, pp.1-7.
https://search.emarefa.net/detail/BIM-499727
Modern Language Association (MLA)
Liu, Nan…[et al.]. Evolutionary Voting-Based Extreme Learning Machines. Mathematical Problems in Engineering No. 2014 (2014), pp.1-7.
https://search.emarefa.net/detail/BIM-499727
American Medical Association (AMA)
Liu, Nan& Cao, Jiuwen& Lin, Zhiping& Pek, Pin Pin& Koh, Zhi Xiong& Ong, Marcus Eng Hock. Evolutionary Voting-Based Extreme Learning Machines. Mathematical Problems in Engineering. 2014. Vol. 2014, no. 2014, pp.1-7.
https://search.emarefa.net/detail/BIM-499727
Data Type
Journal Articles
Language
English
Notes
Includes bibliographical references
Record ID
BIM-499727