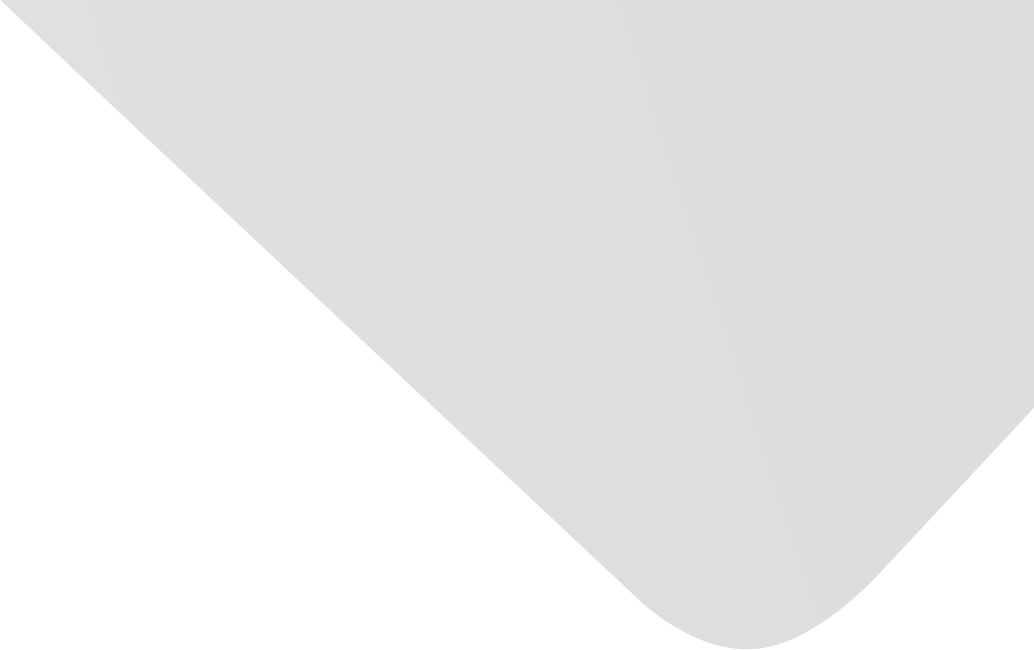
Comparison of Three Supervised Learning Methods for Digital Soil Mapping : Application to a Complex Terrain in the Ecuadorian Andes
Joint Authors
Source
Applied and Environmental Soil Science
Issue
Vol. 2014, Issue 2014 (31 Dec. 2014), pp.1-12, 12 p.
Publisher
Hindawi Publishing Corporation
Publication Date
2014-05-20
Country of Publication
Egypt
No. of Pages
12
Main Subjects
Earth Science , Water and Environment
Abstract EN
A digital soil mapping approach is applied to a complex, mountainous terrain in the Ecuadorian Andes.
Relief features are derived from a digital elevation model and used as predictors for topsoil texture classes sand, silt, and clay.
The performance of three statistical learning methods is compared: linear regression, random forest, and stochastic gradient boosting of regression trees.
In linear regression, a stepwise backward variable selection procedure is applied and overfitting is controlled by minimizing Mallow’s Cp.
For random forest and boosting, the effect of predictor selection and tuning procedures is assessed.
100-fold repetitions of a 5-fold cross-validation of the selected modelling procedures are employed for validation, uncertainty assessment, and method comparison.
Absolute assessment of model performance is achieved by comparing the prediction error of the selected method and the mean.
Boosting performs best, providing predictions that are reliably better than the mean.
The median reduction of the root mean square error is around 5%.
Elevation is the most important predictor.
All models clearly distinguish ridges and slopes.
The predicted texture patterns are interpreted as result of catena sequences (eluviation of fine particles on slope shoulders) and landslides (mixing up mineral soil horizons on slopes).
American Psychological Association (APA)
Hitziger, Martin& Ließ, Mareike. 2014. Comparison of Three Supervised Learning Methods for Digital Soil Mapping : Application to a Complex Terrain in the Ecuadorian Andes. Applied and Environmental Soil Science،Vol. 2014, no. 2014, pp.1-12.
https://search.emarefa.net/detail/BIM-499832
Modern Language Association (MLA)
Hitziger, Martin& Ließ, Mareike. Comparison of Three Supervised Learning Methods for Digital Soil Mapping : Application to a Complex Terrain in the Ecuadorian Andes. Applied and Environmental Soil Science No. 2014 (2014), pp.1-12.
https://search.emarefa.net/detail/BIM-499832
American Medical Association (AMA)
Hitziger, Martin& Ließ, Mareike. Comparison of Three Supervised Learning Methods for Digital Soil Mapping : Application to a Complex Terrain in the Ecuadorian Andes. Applied and Environmental Soil Science. 2014. Vol. 2014, no. 2014, pp.1-12.
https://search.emarefa.net/detail/BIM-499832
Data Type
Journal Articles
Language
English
Notes
Includes bibliographical references
Record ID
BIM-499832