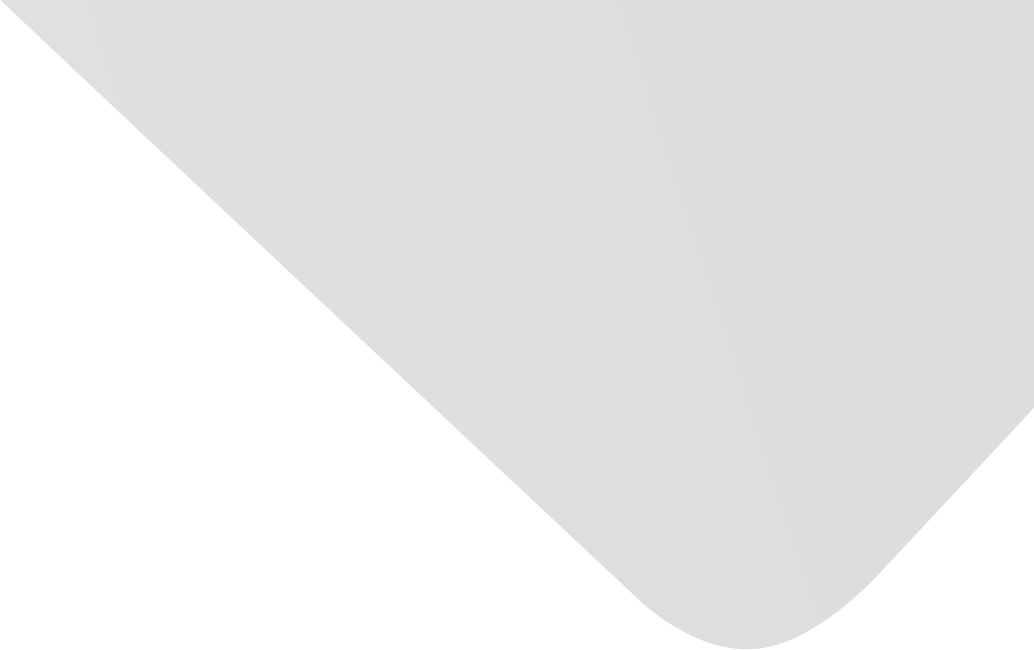
Stock Price Prediction Based on Procedural Neural Networks
Joint Authors
Liang, Jiuzhen
Song, Wei
Wang, Mei
Source
Advances in Artificial Neural Systems
Issue
Vol. 2011, Issue 2011 (31 Dec. 2011), pp.1-11, 11 p.
Publisher
Hindawi Publishing Corporation
Publication Date
2011-06-15
Country of Publication
Egypt
No. of Pages
11
Main Subjects
Information Technology and Computer Science
Abstract EN
We present a spatiotemporal model, namely, procedural neural networks for stock price prediction.
Compared with some successful traditional models on simulating stock market, such as BNN (backpropagation neural networks, HMM (hidden Markov model) and SVM (support vector machine)), the procedural neural network model processes both spacial and temporal information synchronously without slide time window, which is typically used in the well-known recurrent neural networks.
Two different structures of procedural neural networks are constructed for modeling multidimensional time series problems.
Learning algorithms for training the models and sustained improvement of learning are presented and discussed.
Experiments on Yahoo stock market of the past decade years are implemented, and simulation results are compared by PNN, BNN, HMM, and SVM.
American Psychological Association (APA)
Liang, Jiuzhen& Song, Wei& Wang, Mei. 2011. Stock Price Prediction Based on Procedural Neural Networks. Advances in Artificial Neural Systems،Vol. 2011, no. 2011, pp.1-11.
https://search.emarefa.net/detail/BIM-500236
Modern Language Association (MLA)
Liang, Jiuzhen…[et al.]. Stock Price Prediction Based on Procedural Neural Networks. Advances in Artificial Neural Systems No. 2011 (2011), pp.1-11.
https://search.emarefa.net/detail/BIM-500236
American Medical Association (AMA)
Liang, Jiuzhen& Song, Wei& Wang, Mei. Stock Price Prediction Based on Procedural Neural Networks. Advances in Artificial Neural Systems. 2011. Vol. 2011, no. 2011, pp.1-11.
https://search.emarefa.net/detail/BIM-500236
Data Type
Journal Articles
Language
English
Notes
Includes bibliographical references
Record ID
BIM-500236