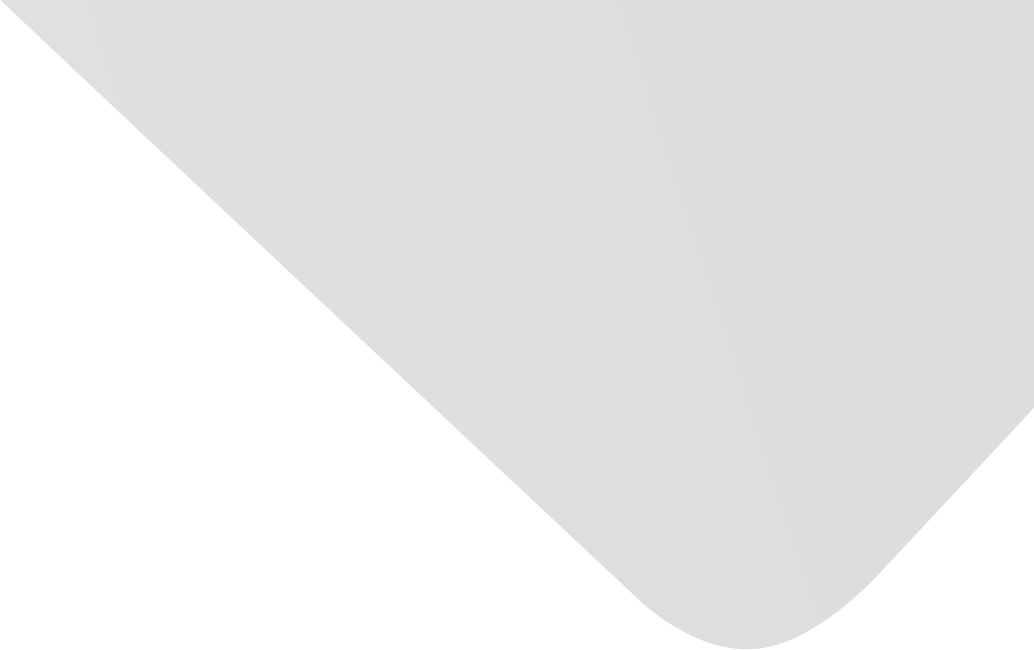
On the Brittleness of Handwritten Digit Recognition Models
Author
Source
Issue
Vol. 2012, Issue 2012 (31 Dec. 2012), pp.1-10, 10 p.
Publisher
Hindawi Publishing Corporation
Publication Date
2011-11-30
Country of Publication
Egypt
No. of Pages
10
Main Subjects
Civil Engineering
Information Technology and Computer Science
Abstract EN
Handwritten digit recognition is an important benchmark task in computer vision.
Learning algorithms and feature representations which offer excellent performance for this task have been known for some time.
Here, we focus on two major practical considerations: the relationship between the the amount of training data and error rate (corresponding to the effort to collect training data to build a model with a given maximum error rate) and the transferability of models' expertise between different datasets (corresponding to the usefulness for general handwritten digit recognition).
While the relationship between amount of training data and error rate is very stable and to some extent independent of the specific dataset used—only the classifier and feature representation have significant effect—it has proven to be impossible to transfer low error rates on one or two pooled datasets to similarly low error rates on another dataset.
We have called this weakness brittleness, inspired by an old Artificial Intelligence term that means the same thing.
This weakness may be a general weakness of trained image classification systems.
American Psychological Association (APA)
Seewald, Alexander K.. 2011. On the Brittleness of Handwritten Digit Recognition Models. ISRN Machine Vision،Vol. 2012, no. 2012, pp.1-10.
https://search.emarefa.net/detail/BIM-501806
Modern Language Association (MLA)
Seewald, Alexander K.. On the Brittleness of Handwritten Digit Recognition Models. ISRN Machine Vision No. 2012 (2012), pp.1-10.
https://search.emarefa.net/detail/BIM-501806
American Medical Association (AMA)
Seewald, Alexander K.. On the Brittleness of Handwritten Digit Recognition Models. ISRN Machine Vision. 2011. Vol. 2012, no. 2012, pp.1-10.
https://search.emarefa.net/detail/BIM-501806
Data Type
Journal Articles
Language
English
Notes
Includes bibliographical references
Record ID
BIM-501806