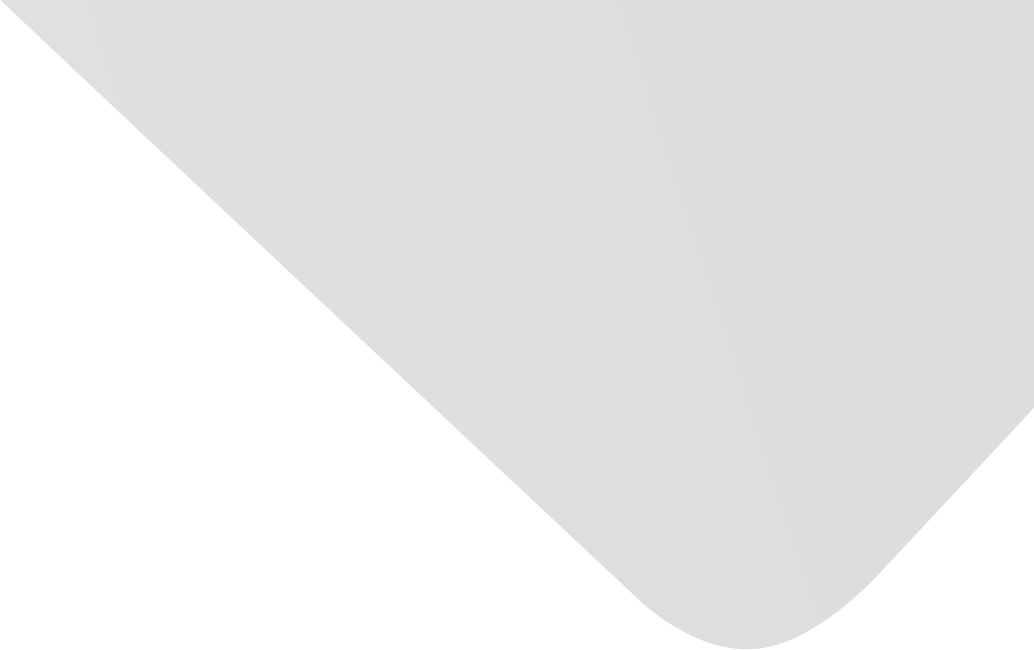
Online Detection of Abnormal Events in Video Streams
Joint Authors
Wang, Tian
Snoussi, Hichem
Chen, Jie
Source
Journal of Electrical and Computer Engineering
Issue
Vol. 2013, Issue 2013 (31 Dec. 2013), pp.1-12, 12 p.
Publisher
Hindawi Publishing Corporation
Publication Date
2013-12-24
Country of Publication
Egypt
No. of Pages
12
Main Subjects
Engineering Sciences and Information Technology
Information Technology and Computer Science
Abstract EN
We propose an algorithm to handle the problem of detecting abnormal events, which is a challenging but important subject in video surveillance.
The algorithm consists of an image descriptor and online nonlinear classification method.
We introduce the covariance matrix of the optical flow and image intensity as a descriptor encoding moving information.
The nonlinear online support vector machine (SVM) firstly learns a limited set of the training frames to provide a basic reference model then updates the model and detects abnormal events in the current frame.
We finally apply the method to detect abnormal events on a benchmark video surveillance dataset to demonstrate the effectiveness of the proposed technique.
American Psychological Association (APA)
Wang, Tian& Chen, Jie& Snoussi, Hichem. 2013. Online Detection of Abnormal Events in Video Streams. Journal of Electrical and Computer Engineering،Vol. 2013, no. 2013, pp.1-12.
https://search.emarefa.net/detail/BIM-502093
Modern Language Association (MLA)
Wang, Tian…[et al.]. Online Detection of Abnormal Events in Video Streams. Journal of Electrical and Computer Engineering No. 2013 (2013), pp.1-12.
https://search.emarefa.net/detail/BIM-502093
American Medical Association (AMA)
Wang, Tian& Chen, Jie& Snoussi, Hichem. Online Detection of Abnormal Events in Video Streams. Journal of Electrical and Computer Engineering. 2013. Vol. 2013, no. 2013, pp.1-12.
https://search.emarefa.net/detail/BIM-502093
Data Type
Journal Articles
Language
English
Notes
Includes bibliographical references
Record ID
BIM-502093