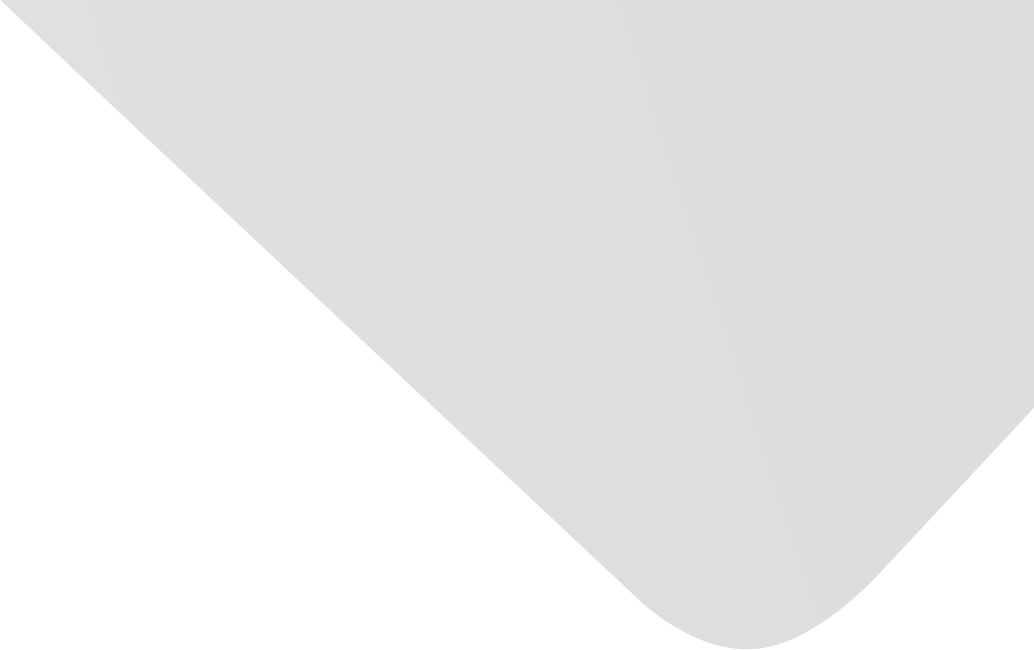
A Comparison Study of Extreme Learning Machine and Least Squares Support Vector Machine for Structural Impact Localization
Author
Source
Mathematical Problems in Engineering
Issue
Vol. 2014, Issue 2014 (31 Dec. 2014), pp.1-8, 8 p.
Publisher
Hindawi Publishing Corporation
Publication Date
2014-07-14
Country of Publication
Egypt
No. of Pages
8
Main Subjects
Abstract EN
Extreme learning machine (ELM) is a learning algorithm for single-hidden layer feedforward neural network dedicated to an extremely fast learning.
However, the performance of ELM in structural impact localization is unknown yet.
In this paper, a comparison study of ELM with least squares support vector machine (LSSVM) is presented for the application on impact localization of a plate structure with surface-mounted piezoelectric sensors.
Both basic and kernel-based ELM regression models have been developed for the location prediction.
Comparative studies of the basic ELM, kernel-based ELM, and LSSVM models are carried out.
Results show that the kernel-based ELM requires the shortest learning time and it is capable of producing suboptimal localization accuracy among the three models.
Hence, ELM paves a promising way in structural impact detection.
American Psychological Association (APA)
Xu, Qingsong. 2014. A Comparison Study of Extreme Learning Machine and Least Squares Support Vector Machine for Structural Impact Localization. Mathematical Problems in Engineering،Vol. 2014, no. 2014, pp.1-8.
https://search.emarefa.net/detail/BIM-507037
Modern Language Association (MLA)
Xu, Qingsong. A Comparison Study of Extreme Learning Machine and Least Squares Support Vector Machine for Structural Impact Localization. Mathematical Problems in Engineering No. 2014 (2014), pp.1-8.
https://search.emarefa.net/detail/BIM-507037
American Medical Association (AMA)
Xu, Qingsong. A Comparison Study of Extreme Learning Machine and Least Squares Support Vector Machine for Structural Impact Localization. Mathematical Problems in Engineering. 2014. Vol. 2014, no. 2014, pp.1-8.
https://search.emarefa.net/detail/BIM-507037
Data Type
Journal Articles
Language
English
Notes
Includes bibliographical references
Record ID
BIM-507037