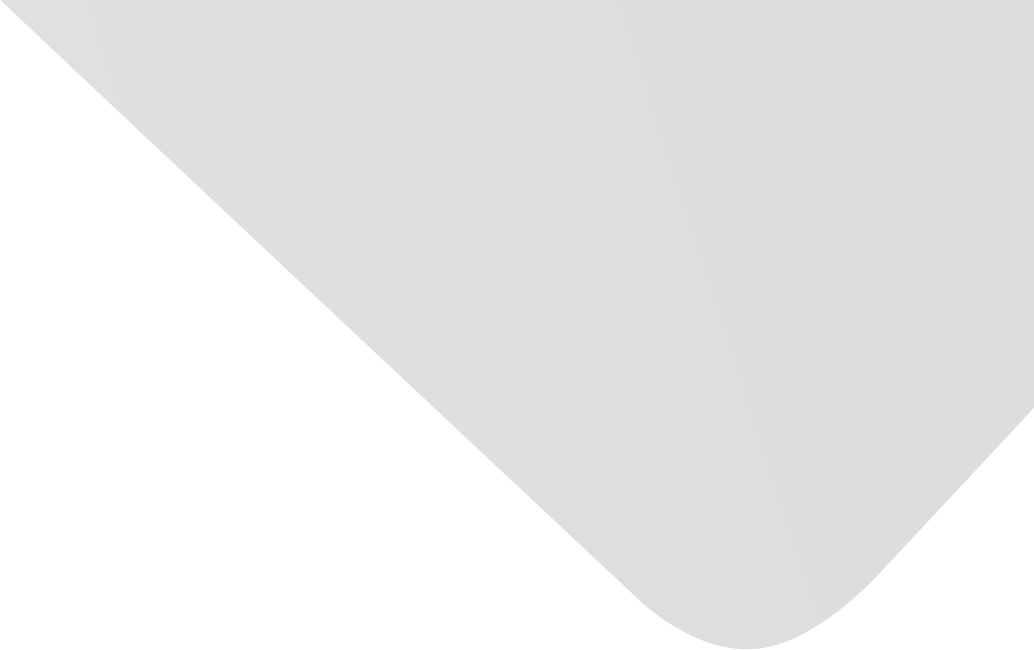
Predicting Cooling Loads for the Next 24 Hours Based on General Regression Neural Network : Methods and Results
Joint Authors
Pan, Song
Wang, Wei
Sun, Yuying
Zhao, Yaohua
Source
Advances in Mechanical Engineering
Issue
Vol. 2013, Issue 2013 (31 Dec. 2013), pp.1-8, 8 p.
Publisher
Hindawi Publishing Corporation
Publication Date
2013-11-18
Country of Publication
Egypt
No. of Pages
8
Main Subjects
Abstract EN
Predicting cooling load for the next 24 hours is essential for the optimal control of air-conditioning systems that use thermal cool storage.
This study investigated modeling methods of applying the general regression neural network (GRNN) technology to predict load.
The single stage (SS) and double stage (DS) prediction methods were introduced.
Two SS and two DS models were set up for forecasting the next 24 hours’ cooling load.
Measured data collected from two five star hotels located in Sanya, China, were used to train and test these models.
The results demonstrate that the SS method, which can eliminate the necessity for measuring and predicting meteorological data, is much simpler and reliable for predicting the cooling load in practical applications.
American Psychological Association (APA)
Sun, Yuying& Wang, Wei& Zhao, Yaohua& Pan, Song. 2013. Predicting Cooling Loads for the Next 24 Hours Based on General Regression Neural Network : Methods and Results. Advances in Mechanical Engineering،Vol. 2013, no. 2013, pp.1-8.
https://search.emarefa.net/detail/BIM-511133
Modern Language Association (MLA)
Sun, Yuying…[et al.]. Predicting Cooling Loads for the Next 24 Hours Based on General Regression Neural Network : Methods and Results. Advances in Mechanical Engineering No. 2013 (2013), pp.1-8.
https://search.emarefa.net/detail/BIM-511133
American Medical Association (AMA)
Sun, Yuying& Wang, Wei& Zhao, Yaohua& Pan, Song. Predicting Cooling Loads for the Next 24 Hours Based on General Regression Neural Network : Methods and Results. Advances in Mechanical Engineering. 2013. Vol. 2013, no. 2013, pp.1-8.
https://search.emarefa.net/detail/BIM-511133
Data Type
Journal Articles
Language
English
Notes
Includes bibliographical references
Record ID
BIM-511133