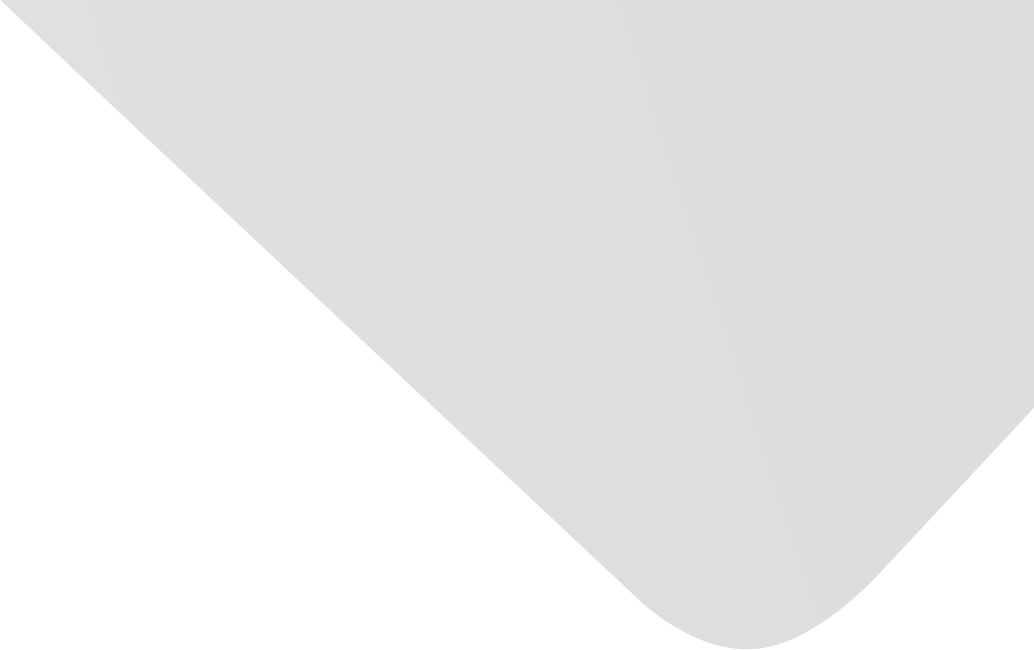
Single Directional SMO Algorithm for Least Squares Support Vector Machines
Joint Authors
Liao, Bifeng
Wu, Kun
Shao, Xigao
Source
Computational Intelligence and Neuroscience
Issue
Vol. 2013, Issue 2013 (31 Dec. 2013), pp.1-7, 7 p.
Publisher
Hindawi Publishing Corporation
Publication Date
2013-02-18
Country of Publication
Egypt
No. of Pages
7
Main Subjects
Abstract EN
Working set selection is a major step in decomposition methods for training least squares support vector machines (LS-SVMs).
In this paper, a new technique for the selection of working set in sequential minimal optimization- (SMO-) type decomposition methods is proposed.
By the new method, we can select a single direction to achieve the convergence of the optimality condition.
A simple asymptotic convergence proof for the new algorithm is given.
Experimental comparisons demonstrate that the classification accuracy of the new method is not largely different from the existing methods, but the training speed is faster than existing ones.
American Psychological Association (APA)
Shao, Xigao& Wu, Kun& Liao, Bifeng. 2013. Single Directional SMO Algorithm for Least Squares Support Vector Machines. Computational Intelligence and Neuroscience،Vol. 2013, no. 2013, pp.1-7.
https://search.emarefa.net/detail/BIM-512205
Modern Language Association (MLA)
Shao, Xigao…[et al.]. Single Directional SMO Algorithm for Least Squares Support Vector Machines. Computational Intelligence and Neuroscience No. 2013 (2013), pp.1-7.
https://search.emarefa.net/detail/BIM-512205
American Medical Association (AMA)
Shao, Xigao& Wu, Kun& Liao, Bifeng. Single Directional SMO Algorithm for Least Squares Support Vector Machines. Computational Intelligence and Neuroscience. 2013. Vol. 2013, no. 2013, pp.1-7.
https://search.emarefa.net/detail/BIM-512205
Data Type
Journal Articles
Language
English
Notes
Includes bibliographical references
Record ID
BIM-512205