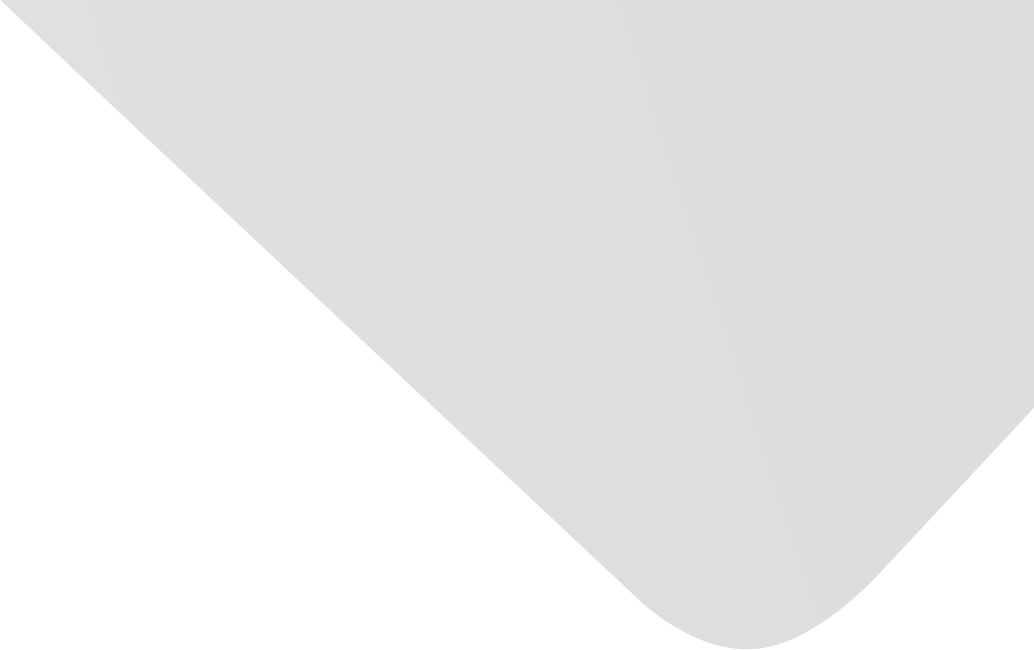
HMM based POS tagging system for 8 different languages and several tagsets
Other Title(s)
نظام ترميز أقسام الكلام معتمدا موديل ماركوف المخفي لثمان لغات و عدة مجاميع ترميز
Joint Authors
Alawi, Ahmad Husayn
Radi, Rasul Ali
Hamid, Hiba Sartil
Source
Engineering and Technology Journal
Issue
Vol. 33, Issue 2 (28 Feb. 2015), pp.326-337, 12 p.
Publisher
Publication Date
2015-02-28
Country of Publication
Iraq
No. of Pages
12
Main Subjects
Information Technology and Computer Science
Abstract AR
نقترح في بحثنا نظام ترميز الكلمات بأقسام الكلام باستخدام طريقة HMM لعدة لغات.
طبقنا HMM باستخدام خوارزمية Viterbi على ثمان لغات هي اللغة الإنجليزية و الهندية و التلوكو و البنكالية و المهاراتية و الصينية القياسية و البرتغالية و الإسبانية.
البيانات لهذه اللغات أخذناها من ذخائر (مدونات) موجودة بشكل مجاني و هي Floresta, Sinica, NPS-Chat Indiana, Brown و CESS-ESP.
HMM هي من أكثر طرق التعلم المستخدمة في تطبيقات كثيرة لمعالجة اللغات الطبيعية خصوصا الترميز بأقسام الكلام, و أن بعض الباحثين الآخرين نفذوا مرمز HMM على لغات كثيرة حيث كل باحث نفذها على لغته.
تنفيذنا للنظام تم من خلال تقسيم كل ذخيرة (البيانات) إلى 99 % للتدريب و 1 % للفحص، هذه العملية تعاد لعشرة مرات من خلال تغيير بيانات التدريب و الفحص، و كانت الدقة (كمعدل لجميع الفحوصات) للغة الإنجليزية (مجموعتي ترميز 40 و 472 رمز) و الإنكليزية (ذخيرة NPS-Chat) و الهندية و التلوكو و البنكالية و الصينية القياسية و البرتغالية (مجموعتي ترميز 32 و 269 رمز) و الإسبانية (مجموعتي ترميز 14 و 289 رمز) هي (95.3 % و 92.39 %)، 87.17 %، 81.3 %، 74.03 %، 72.01 %، 69.56 %، 87.59 %، (84.56 % و 83.95 %) و (94.26 %، 92.08 %) على الترتيب.
اللغات المختلفة أخذناها لغرض تسجيل تحديدات مرمز HMM على لغات مختلفة كما سنرى، و هذا يعني تسجيل التحديدات باستخدام طريقة واحدة على عدة لغات.
كذلك أخذنا نفس الذخيرة معنونة بمجموعة رموز مختلفة لغرض دراسة تأثير حجم مجموعة الرموز، بالإضافة إلى ذلك أخذنا ذخيرتين مختلفتين لنفس اللغة، فحسب معلوماتنا ليس هناك دراسة معمقة منفذة على مرمز بنفس الحالات المأخوذة في هذا العمل.
و فرنا أيضا برنامج تطبيقي لترميز جميع الكلمات لأي جملة من أي من اللغات المستخدمة في عملنا.
الكلمات الغير معروفة (غير موجودة في بيانات التدريب) عالجناها بطريقة بسيطة جداً و هي Laplace smoothing.
Abstract EN
We propose, in this paper, Part-Of-Speech (POS) tagging system is proposed which based on Hidden Markov Model (HMM) for several languages.
HMM is implemented using Viterbi algorithm on 8 languages ; English, Hindi, Telugu, Bangla (Bengali), Marathi, Standard Chinese, Portuguese and Spanish.
The data for these languages were taken from the freely available corpora : Brown, NPS-Chat, Indiana, Sinica, Floresta and CESS-ESP Corpora.
HMM is the most learning method used in many NLP applications, especially POS tagging.
HMM tagger was implemented by other researchers for a lot of languages, where each one take his mother tongue language.
system testing is done by splitting each corpus to 99 % training and 1% testing.
This test is repeated for 10 times by changing the training and test data.
The accuracies (average for all 10 tests) for English (using two tagsets of 40 tags and 472 tags), English (NPS corpus), Hindi, Telugu, Bangla or Bengali, Marathi, Standard Chinese, Portuguese (using two tagsets of 32 tags and 269 tags), and Spanish (using two tagsets of 14 tags and 289 tags) are (95.3 % & 92.39 %), 87.17 %, 81.3 %, 74.03 %, 72.01 %, 69.56 %, 87.59 %, (84.56 % & 83.95 %), and (94.26 % & 92.08 %) respectively.
Several languages are taken for recording the limitations of HMM tagger on different languages as will be seen, I.e, the limitations of using one method on many different languages are recorded.
Same corpus annotated with different tagsets is taken for studying the effect of tagset’s size.
Also two different corpora, for the same language, are taken.
According to our knowledge, there isn’t study implemented HMM on such various cases as in our work.
We provide an executable application1 for tagging all words in any sentence for any of the used 8 languages in our work.
The unknown words (words not exist in the trained data) are manipulated by a simple method as Laplace smoothing.
American Psychological Association (APA)
Alawi, Ahmad Husayn& Radi, Rasul Ali& Hamid, Hiba Sartil. 2015. HMM based POS tagging system for 8 different languages and several tagsets. Engineering and Technology Journal،Vol. 33, no. 2, pp.326-337.
https://search.emarefa.net/detail/BIM-568212
Modern Language Association (MLA)
Alawi, Ahmad Husayn…[et al.]. HMM based POS tagging system for 8 different languages and several tagsets. Engineering and Technology Journal Vol. 33, no. 2 (2015), pp.326-337.
https://search.emarefa.net/detail/BIM-568212
American Medical Association (AMA)
Alawi, Ahmad Husayn& Radi, Rasul Ali& Hamid, Hiba Sartil. HMM based POS tagging system for 8 different languages and several tagsets. Engineering and Technology Journal. 2015. Vol. 33, no. 2, pp.326-337.
https://search.emarefa.net/detail/BIM-568212
Data Type
Journal Articles
Language
English
Notes
Includes bibliographical references : p. 337
Record ID
BIM-568212