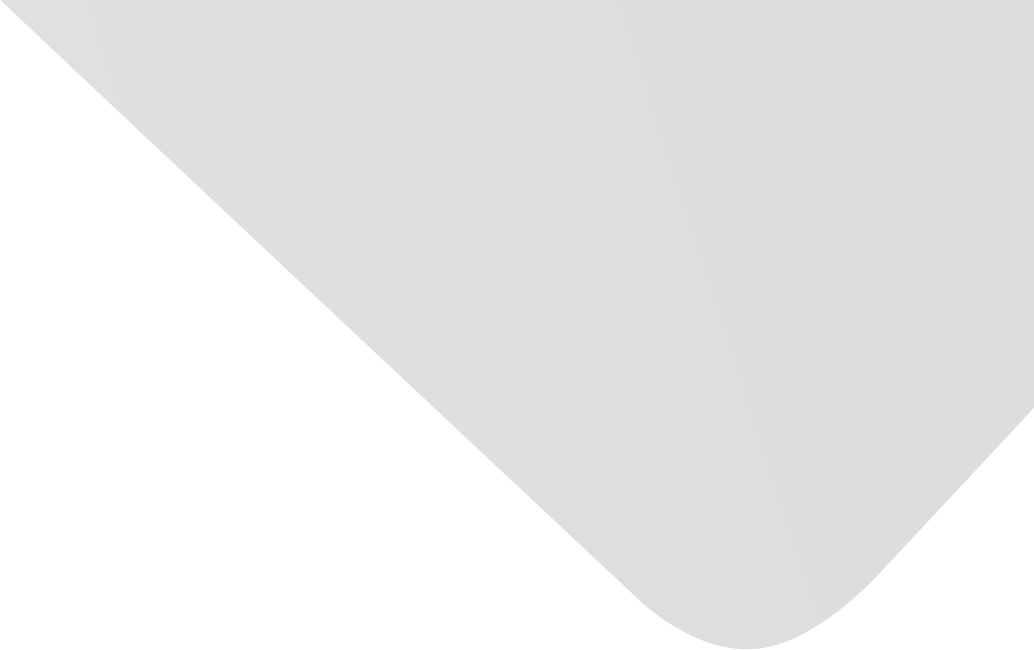
Automated flower species detection and recognition from digital images
Other Title(s)
الكشف و التمييز الآلي لأنواع الزهور من الصور الرقمية
Dissertant
Thesis advisor
Comitee Members
Muhanna, Muhanna
Kabnah, Khalid Abd al-Hafiz
al-Halabi, Yahya
University
Princess Sumaya University for Technology
Faculty
King Hussein Faculty for Computing Sciences
Department
Department of Computer Sciences
University Country
Jordan
Degree
Master
Degree Date
2016
English Abstract
Automated flower species recognition has been studied for many years.
Differences between these studies come from features which were extracted from the flower image, and the recognition algorithm that was used to recognize the flower species.
A new automated system was adapted to detect the flower region from the image and recognize its species.
By using a modified segmentation method, the features were extracted from the interest part only, so this increased the recognition accuracy.
The study aims at improving the flower recognition accuracy by extracting features from flowers’ leaf image.
Leaves are served as an important addition to the flower features, which is not been addressed by most of literatures.
Two datasets have been built, the first is Aalaa19 Dataset for flower only, and contains flowers from Jordan.
Including Iris Nigricans the national flower of Jordan, which is not addressed even in the famous Oxford17 dataset.
The second is FlowerAndLeaf21 Dataset for flowers with their leaves to study their effects on recognition accuracy.
Region growing segmentation was applied to extract flower and leaf region from their images.
Features based on color, texture, and shape have been extracted and used to train the Stochastic Gradient Descent (SGD) classifier with one versus all approach.
The results showed that the recognition accuracy for Aalaa19 Dataset is 92%.
The result of the second dataset FlowerAndLeaf21 showed that considering leaf in recognition increased the recognition accuracy from 71.1% to 77.31% with SGD classifier, while using random forest classifier increased the recognition accuracy from 83.5% to 85.5%.
Our proposed system outperforms several methods on Oxfoed17 Dataset; a class average recognition accuracy of 83.52% was achieved.
Main Subjects
Information Technology and Computer Science
No. of Pages
84
Table of Contents
Table of contents.
Abstract.
Abstract in Arabic.
Chapter One : Introduction.
Chapter Two : Literature review.
Chapter Three : Background.
Chapter Four : Proposed system overview.
Chapter Five : Experiments.
Chapter Six : Results and discussion.
Chapter Seven : Conclusion and future work.
References.
American Psychological Association (APA)
al-Badarinah, Ala Abd Allah. (2016). Automated flower species detection and recognition from digital images. (Master's theses Theses and Dissertations Master). Princess Sumaya University for Technology, Jordan
https://search.emarefa.net/detail/BIM-693652
Modern Language Association (MLA)
al-Badarinah, Ala Abd Allah. Automated flower species detection and recognition from digital images. (Master's theses Theses and Dissertations Master). Princess Sumaya University for Technology. (2016).
https://search.emarefa.net/detail/BIM-693652
American Medical Association (AMA)
al-Badarinah, Ala Abd Allah. (2016). Automated flower species detection and recognition from digital images. (Master's theses Theses and Dissertations Master). Princess Sumaya University for Technology, Jordan
https://search.emarefa.net/detail/BIM-693652
Language
English
Data Type
Arab Theses
Record ID
BIM-693652