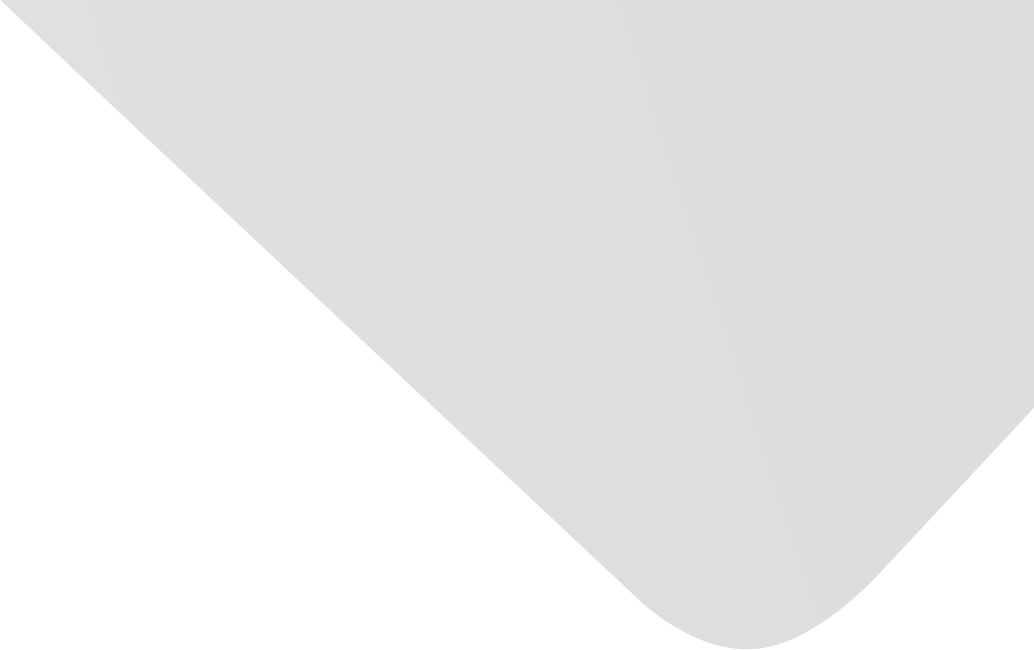
A multiple classifier system for supervised classification of remotely sensed data
Author
Source
Issue
Vol. 14, Issue 1 العلوم الصرفة و الهندسية (30 Jun. 2011), pp.260-273, 14 p.
Publisher
Publication Date
2011-06-30
Country of Publication
Iraq
No. of Pages
14
Main Subjects
Earth Sciences, Water and Environment
Abstract EN
In this paper a new scheme of multiple classifier system (MCS) for remote sensing data classification is proposed.
It includes four member classifiers; maximum likelihood, minimum distance and two differently trained supervised artificial neural networks of type adaptive resonance theory ART_II.
The system is based on newly developed method of integration, named Local Ranking (LK).
This method is categorized as dynamic classifier selection (DCS) approach and based on ranking the classifiers for each class on the basis of pre-estimation of the class mapping accuracy from training data.
The system is applied to multi-spectral image, taken by landsat-7 with ETM+ sensor, of Duhok city in Kurdistan region to classify seven cover types (residential area, water surface, dense vegetation, less dense vegetation, spars vegetation, wet soil and dry soil).
The results have shown the superiority of the system performance over the performance of the individual classifiers in term of class and average accuracy.
The increase in system performance for the seven classes compared to the highest class accuracy provided by any of the individual classifiers was 1.59%, 0.0%, 0.7%, 5.14%, 13.21%, 4.67%, 2.02% respectively.
While the increase in the average accuracy compared to the highest average accuracy provided by maximum likelihood classifier was 4.30%.
This increase correspond to an area of (10.15) km2.
The efficiency of the local ranking (LR) method is compared to the well known methods, local accuracy (LA) and majority voting (MV).
The results have shown the superiority of the developed method (LR) over LA and MV in terms of average and class accuracy.
The average accuracies of LR, LA and MV were (94.68%), (91.54%) and (91.57%) which correspond to (3.14%) and (3.11%) improvements in the favor of LR over LA and MV.
These improvements are equivalent to areas of (7.4) km2 and (7.34) km2.
For individual class accuracy, LR method provided highest accuracy for three classes, LA method provided highest accuracy for two classes and MV provided highest accuracy for only one class, while all three methods provided same accuracy for one class.
American Psychological Association (APA)
Tahir, Ahmad Ak.. 2011. A multiple classifier system for supervised classification of remotely sensed data. Journal of Dohuk University،Vol. 14, no. 1 العلوم الصرفة و الهندسية, pp.260-273.
https://search.emarefa.net/detail/BIM-761155
Modern Language Association (MLA)
Tahir, Ahmad Ak.. A multiple classifier system for supervised classification of remotely sensed data. Journal of Dohuk University Vol. 14, no. 1 Pure and Engineering Sciences (2011), pp.260-273.
https://search.emarefa.net/detail/BIM-761155
American Medical Association (AMA)
Tahir, Ahmad Ak.. A multiple classifier system for supervised classification of remotely sensed data. Journal of Dohuk University. 2011. Vol. 14, no. 1 العلوم الصرفة و الهندسية, pp.260-273.
https://search.emarefa.net/detail/BIM-761155
Data Type
Journal Articles
Language
English
Notes
Includes bibliographical references : p. 270-271
Record ID
BIM-761155