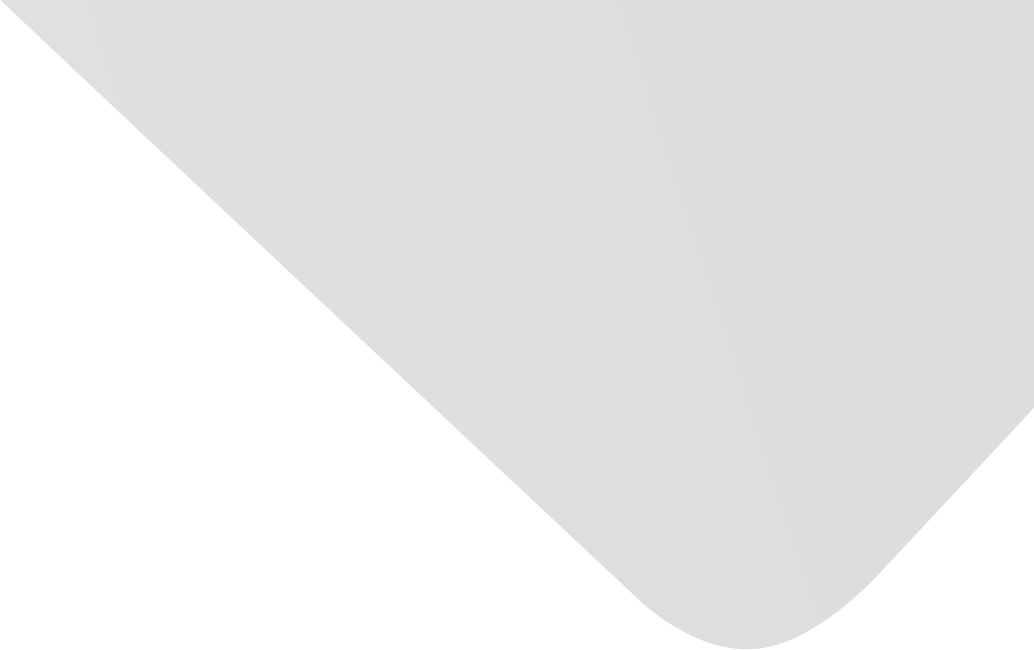
Neural network modeling of cutting force and chip thickness ratio for turning aluminum alloy 7075-T6
Other Title(s)
نمذجة قوة القطع و نسبة سمك النحاتة باستخدام الشبكات العصبية أثناء خراطة سبيكة الألمنيوم 7075-T6
Author
al-Khafaji, Muhannad Muhammad Husayn
Source
al-Khwarizmi Engineering Journal
Issue
Vol. 14, Issue 1 (31 Mar. 2018), pp.67-76, 10 p.
Publisher
University of Baghdad al-Khwarizmi College of Engineering
Publication Date
2018-03-31
Country of Publication
Iraq
No. of Pages
10
Main Subjects
Abstract EN
The turning process has various factors, which affecting machinability and should be investigated.
These are surface roughness, tool life, power consumption, cutting temperature, machining force components, tool wear, and chip thickness ratio.
These factors made the process nonlinear and complicated.
This work aims to build neural network models to correlate the cutting parameters, namely cutting speed, depth of cut and feed rate, to the machining force and chip thickness ratio.
The turning process was performed on high strength aluminum alloy 7075-T6.
Three radial basis neural networks are constructed for cutting force, passive force, and feed force.
In addition, a radial basis network is constructed to model the chip thickness ratio.
The inputs to all networks are cutting speed, depth of cut, and feed rate.
All networks performances (outputs) for all machining force components (cutting force, passive force and feed force) showed perfect match with the experimental data and the calculated correlation coefficients were equal to one.
The built network for the chip thickness ratio is giving correlation coefficient equal one too, when its output compared with the experimental results.
These networks (models) are used to optimize the cutting parameters that produce the lowest machining force and chip thickness ratio.
The models showed that the optimum machining force was (240.46 N) which can be produced when the cutting speed (683 m/min), depth of cut (3.18 mm) and feed rate (0.27 mm/rev).
The proposed network for the chip thickness ratio showed that the minimum chip thickness is (1.21), which is at cutting speed (683 m/min), depth of cut (3.18 mm) and feed rate (0.17 mm/rev).
American Psychological Association (APA)
al-Khafaji, Muhannad Muhammad Husayn. 2018. Neural network modeling of cutting force and chip thickness ratio for turning aluminum alloy 7075-T6. al-Khwarizmi Engineering Journal،Vol. 14, no. 1, pp.67-76.
https://search.emarefa.net/detail/BIM-831993
Modern Language Association (MLA)
al-Khafaji, Muhannad Muhammad Husayn. Neural network modeling of cutting force and chip thickness ratio for turning aluminum alloy 7075-T6. al-Khwarizmi Engineering Journal Vol. 14, no. 1 (Mar. 2018), pp.67-76.
https://search.emarefa.net/detail/BIM-831993
American Medical Association (AMA)
al-Khafaji, Muhannad Muhammad Husayn. Neural network modeling of cutting force and chip thickness ratio for turning aluminum alloy 7075-T6. al-Khwarizmi Engineering Journal. 2018. Vol. 14, no. 1, pp.67-76.
https://search.emarefa.net/detail/BIM-831993
Data Type
Journal Articles
Language
English
Notes
Includes bibliographical references : p.74-75
Record ID
BIM-831993