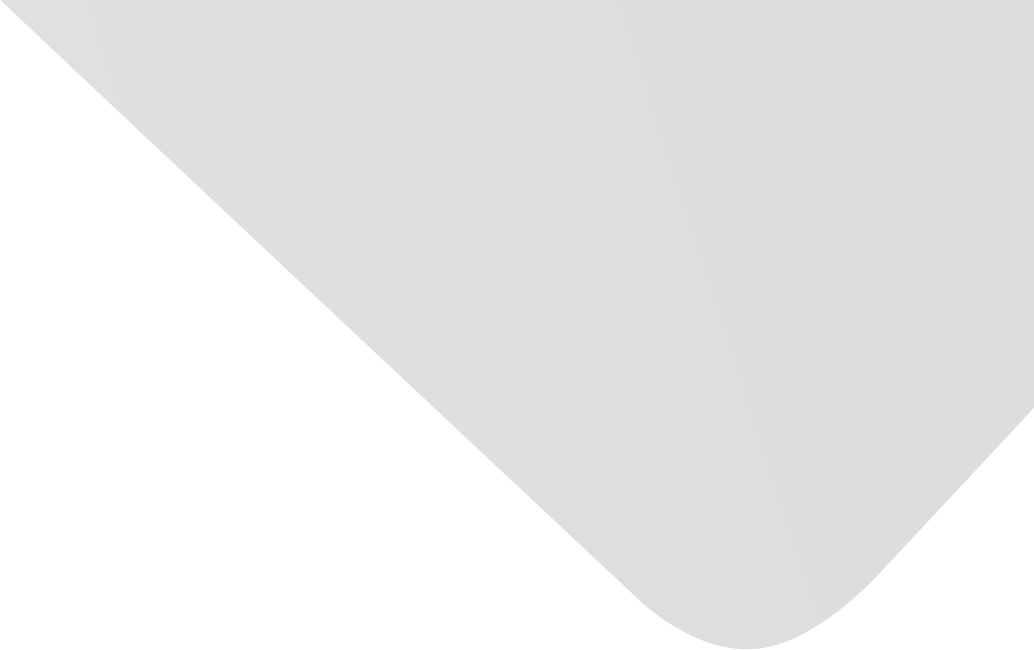
Very short term load forecasting based on meteorological with modelling k-NN-feed forward neural network
Joint Authors
Kartini, Unit Three
Ardianto, Dwi
Wardani, Laili
Source
Issue
Vol. 15, Issue 1 (31 Mar. 2019), pp.1-16, 16 p.
Publisher
Publication Date
2019-03-31
Country of Publication
Algeria
No. of Pages
16
Main Subjects
Topics
Abstract EN
This paper proposes a novel methodology for very short term load forecasting of hourly.
The proposed methodology is based on meteorology data i.e.
temperature, humidity, especially for optimizing the operation of power generating electricity from thermal unit generation.
This modelling methodology is a combination of k-nearest neighbor (k-NN) method and feed forward- Neural Network (Feed-Forward-NN) method.
The k-NN-Feed-Forward NN model is designed to prediction load for 1 hour ahead based on meteorology data for the target Thermal Unit Generation which position adjacent by twelve hydro thermal unit generation.
The novelty of this model is taking into account the meteorology data.
A set of load measurement samples was available from the hydro thermal unit generation in Indonesia Region 4 which is used as test data.
The first model implements k-NN as a input data preprocessing technique prior to feed forward NN model.
The error statistical indicators of k-NN-Feed-Forward-NN method The mean absolute deviation error statistical indicators of k-NN model is 103.48 MW and MAPE is 18.8%.
On the other hand, the error statistical indicator for proposed model (Euclidean k-NNfeed forward-NN model) MAD is 19.37 MW and MAPE is 2.21%.
Note that the highest mean absolute deviation (MAD) was 75,11 MW and mean absolute percentage error (MAPE) was 10.38% during the twelve period.
The models forecasts are then compared to measured data and simulation results indicate that the k-NN-Feed Forward NN-based method presented in this research can calculate hourly load with satisfactory accuracy.
American Psychological Association (APA)
Kartini, Unit Three& Ardianto, Dwi& Wardani, Laili. 2019. Very short term load forecasting based on meteorological with modelling k-NN-feed forward neural network. Journal of Electrical Systems،Vol. 15, no. 1, pp.1-16.
https://search.emarefa.net/detail/BIM-861510
Modern Language Association (MLA)
Kartini, Unit Three…[et al.]. Very short term load forecasting based on meteorological with modelling k-NN-feed forward neural network. Journal of Electrical Systems Vol. 15, no. 1 (Mar. 2019), pp.1-16.
https://search.emarefa.net/detail/BIM-861510
American Medical Association (AMA)
Kartini, Unit Three& Ardianto, Dwi& Wardani, Laili. Very short term load forecasting based on meteorological with modelling k-NN-feed forward neural network. Journal of Electrical Systems. 2019. Vol. 15, no. 1, pp.1-16.
https://search.emarefa.net/detail/BIM-861510
Data Type
Journal Articles
Language
English
Notes
Includes bibliographical references : p. 15-16
Record ID
BIM-861510