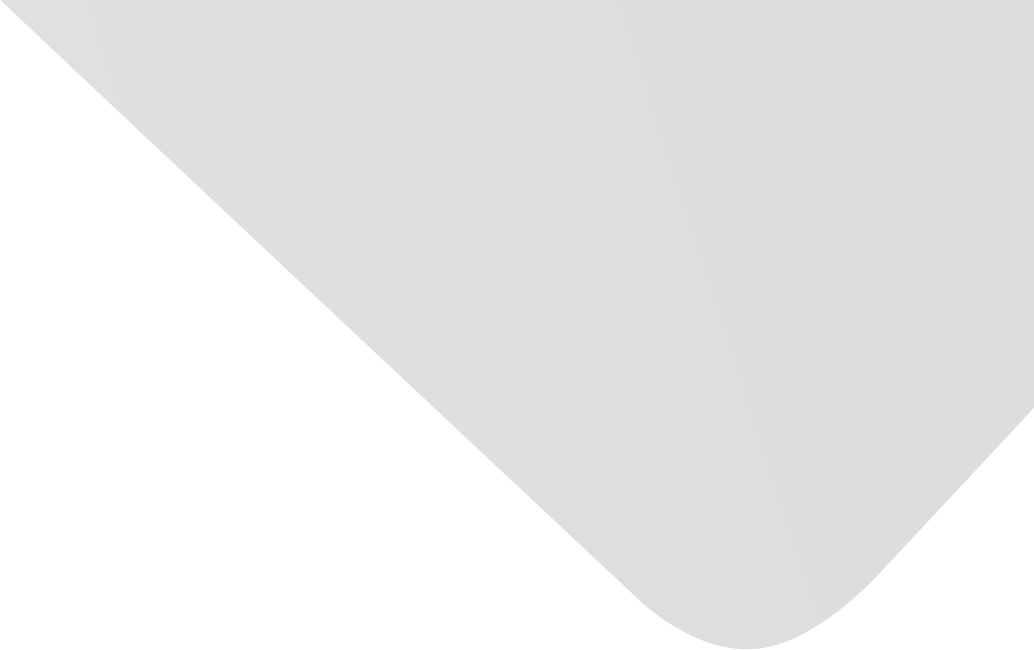
Effect of successive convolution layers to detect gender
Other Title(s)
تأثير الطبقات التلافيفية المتعاقبة لمكشف عن الجنس
Joint Authors
Ahmad, Hana Muhsin
Mahmud, Halah Hasan
Source
Issue
Vol. 59, Issue 3C (30 Sep. 2018), pp.1717-1732, 16 p.
Publisher
University of Baghdad College of Science
Publication Date
2018-09-30
Country of Publication
Iraq
No. of Pages
16
Main Subjects
Information Technology and Computer Science
Abstract EN
Image classification can be defined as one of the most important tasks in the area of machine learning.
recently, deep neural networks, especially deep convolution networks, have participated greatly in end-to-end learning which reduce need for human designed features in the image recognition like convolution neural network.
it is offers the computation models which are made up of several processing layers for learning data representations with several abstraction levels.
in this work, a pre-trained deep cnn is utilized according to some parameters like filter size, no of convolution, pooling, fully connected and type of activation function which includes 300 images for training and predict 100 image gender using probability measures.
results in classification and precision accuracy equal to 0.68 and 0.3225 respectively.
American Psychological Association (APA)
Ahmad, Hana Muhsin& Mahmud, Halah Hasan. 2018. Effect of successive convolution layers to detect gender. Iraqi Journal of Science،Vol. 59, no. 3C, pp.1717-1732.
https://search.emarefa.net/detail/BIM-876172
Modern Language Association (MLA)
Ahmad, Hana Muhsin& Mahmud, Halah Hasan. Effect of successive convolution layers to detect gender. Iraqi Journal of Science Vol. 59, no. 3C (2018), pp.1717-1732.
https://search.emarefa.net/detail/BIM-876172
American Medical Association (AMA)
Ahmad, Hana Muhsin& Mahmud, Halah Hasan. Effect of successive convolution layers to detect gender. Iraqi Journal of Science. 2018. Vol. 59, no. 3C, pp.1717-1732.
https://search.emarefa.net/detail/BIM-876172
Data Type
Journal Articles
Language
English
Notes
Text in English ; abstracts in English and Arabic.
Record ID
BIM-876172