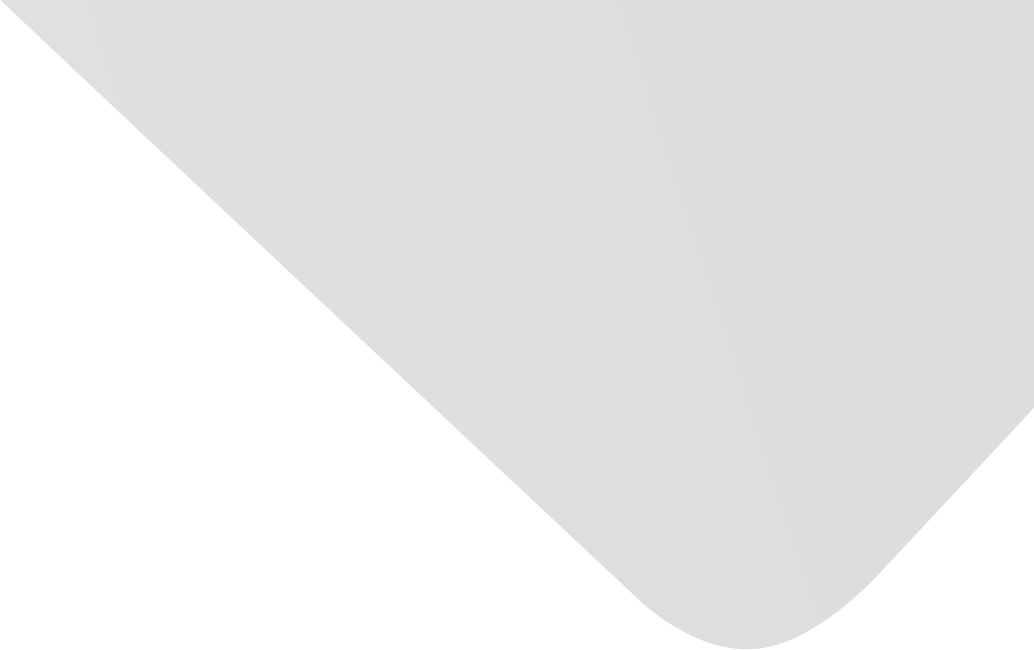
A Hybrid Sampling SVM Approach to Imbalanced Data Classification
المؤلف
المصدر
العدد
المجلد 2014، العدد 2014 (31 ديسمبر/كانون الأول 2014)، ص ص. 1-7، 7ص.
الناشر
Hindawi Publishing Corporation
تاريخ النشر
2014-06-11
دولة النشر
مصر
عدد الصفحات
7
التخصصات الرئيسية
الملخص EN
Imbalanced datasets are frequently found in many real applications.
Resampling is one of the effective solutions due to generating a relatively balanced class distribution.
In this paper, a hybrid sampling SVM approach is proposed combining an oversampling technique and an undersampling technique for addressing the imbalanced data classification problem.
The proposed approach first uses an undersampling technique to delete some samples of the majority class with less classification information and then applies an oversampling technique to gradually create some new positive samples.
Thus, a balanced training dataset is generated to replace the original imbalanced training dataset.
Finally, through experimental results on the real-world datasets, our proposed approach has the ability to identify informative samples and deal with the imbalanced data classification problem.
نمط استشهاد جمعية علماء النفس الأمريكية (APA)
Wang, Qiang. 2014. A Hybrid Sampling SVM Approach to Imbalanced Data Classification. Abstract and Applied Analysis،Vol. 2014, no. 2014, pp.1-7.
https://search.emarefa.net/detail/BIM-1015181
نمط استشهاد الجمعية الأمريكية للغات الحديثة (MLA)
Wang, Qiang. A Hybrid Sampling SVM Approach to Imbalanced Data Classification. Abstract and Applied Analysis No. 2014 (2014), pp.1-7.
https://search.emarefa.net/detail/BIM-1015181
نمط استشهاد الجمعية الطبية الأمريكية (AMA)
Wang, Qiang. A Hybrid Sampling SVM Approach to Imbalanced Data Classification. Abstract and Applied Analysis. 2014. Vol. 2014, no. 2014, pp.1-7.
https://search.emarefa.net/detail/BIM-1015181
نوع البيانات
مقالات
لغة النص
الإنجليزية
الملاحظات
Includes bibliographical references
رقم السجل
BIM-1015181
قاعدة معامل التأثير والاستشهادات المرجعية العربي "ارسيف Arcif"
أضخم قاعدة بيانات عربية للاستشهادات المرجعية للمجلات العلمية المحكمة الصادرة في العالم العربي
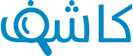
تقوم هذه الخدمة بالتحقق من التشابه أو الانتحال في الأبحاث والمقالات العلمية والأطروحات الجامعية والكتب والأبحاث باللغة العربية، وتحديد درجة التشابه أو أصالة الأعمال البحثية وحماية ملكيتها الفكرية. تعرف اكثر
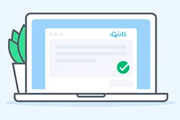