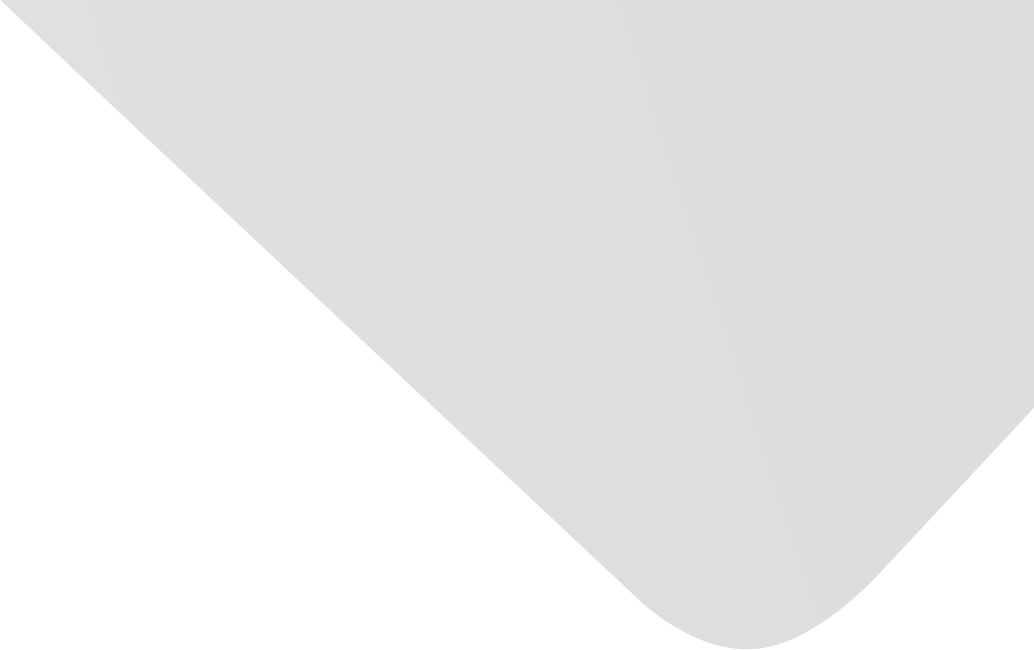
A Hybrid Sampling SVM Approach to Imbalanced Data Classification
Author
Source
Issue
Vol. 2014, Issue 2014 (31 Dec. 2014), pp.1-7, 7 p.
Publisher
Hindawi Publishing Corporation
Publication Date
2014-06-11
Country of Publication
Egypt
No. of Pages
7
Main Subjects
Abstract EN
Imbalanced datasets are frequently found in many real applications.
Resampling is one of the effective solutions due to generating a relatively balanced class distribution.
In this paper, a hybrid sampling SVM approach is proposed combining an oversampling technique and an undersampling technique for addressing the imbalanced data classification problem.
The proposed approach first uses an undersampling technique to delete some samples of the majority class with less classification information and then applies an oversampling technique to gradually create some new positive samples.
Thus, a balanced training dataset is generated to replace the original imbalanced training dataset.
Finally, through experimental results on the real-world datasets, our proposed approach has the ability to identify informative samples and deal with the imbalanced data classification problem.
American Psychological Association (APA)
Wang, Qiang. 2014. A Hybrid Sampling SVM Approach to Imbalanced Data Classification. Abstract and Applied Analysis،Vol. 2014, no. 2014, pp.1-7.
https://search.emarefa.net/detail/BIM-1015181
Modern Language Association (MLA)
Wang, Qiang. A Hybrid Sampling SVM Approach to Imbalanced Data Classification. Abstract and Applied Analysis No. 2014 (2014), pp.1-7.
https://search.emarefa.net/detail/BIM-1015181
American Medical Association (AMA)
Wang, Qiang. A Hybrid Sampling SVM Approach to Imbalanced Data Classification. Abstract and Applied Analysis. 2014. Vol. 2014, no. 2014, pp.1-7.
https://search.emarefa.net/detail/BIM-1015181
Data Type
Journal Articles
Language
English
Notes
Includes bibliographical references
Record ID
BIM-1015181