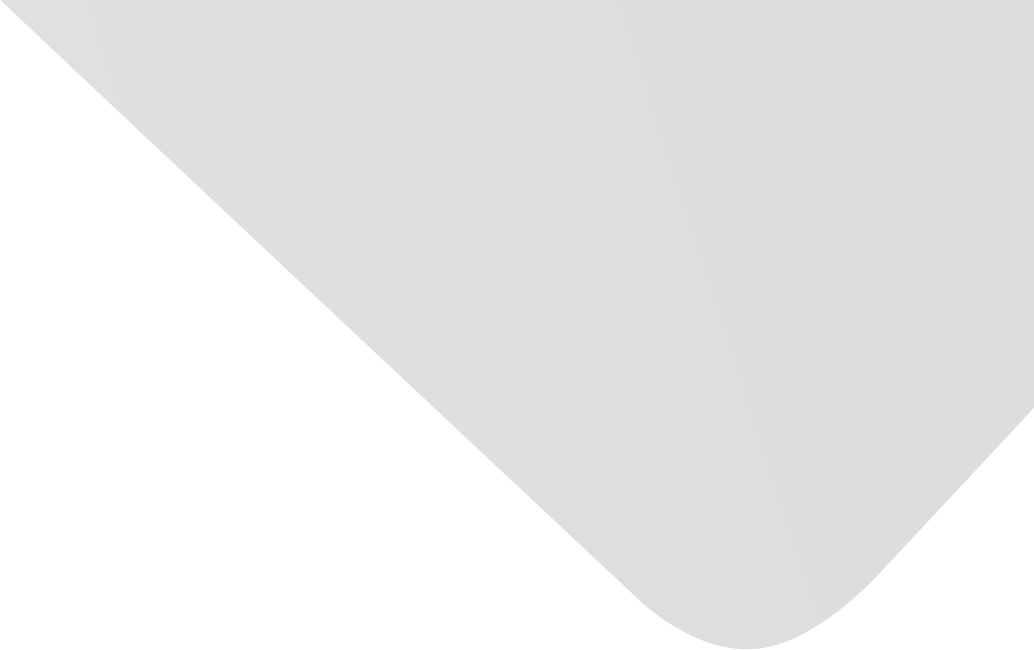
Effect-Size Estimation Using Semiparametric Hierarchical Mixture Models in Disease-Association Studies with Neuroimaging Data
المؤلفون المشاركون
Matsui, Shigeyuki
Emoto, Ryo
Takahashi, Kunihiko
Kawaguchi, Atsushi
المصدر
Computational and Mathematical Methods in Medicine
العدد
المجلد 2020، العدد 2020 (31 ديسمبر/كانون الأول 2020)، ص ص. 1-11، 11ص.
الناشر
Hindawi Publishing Corporation
تاريخ النشر
2020-12-09
دولة النشر
مصر
عدد الصفحات
11
التخصصات الرئيسية
الملخص EN
In disease-association studies using neuroimaging data, evaluating the biological or clinical significance of individual associations requires not only detection of disease-associated areas of the brain but also estimation of the magnitudes of the associations or effect sizes for individual brain areas.
In this paper, we propose a model-based framework for voxel-based inferences under spatial dependency in neuroimaging data.
Specifically, we employ hierarchical mixture models with a hidden Markov random field structure to incorporate the spatial dependency between voxels.
A nonparametric specification is proposed for the effect size distribution to flexibly estimate the underlying effect size distribution.
Simulation experiments demonstrate that compared with a naive estimation method, the proposed methods can substantially reduce the selection bias in the effect size estimates of the selected voxels with the greatest observed associations.
An application to neuroimaging data from an Alzheimer’s disease study is provided.
نمط استشهاد جمعية علماء النفس الأمريكية (APA)
Emoto, Ryo& Kawaguchi, Atsushi& Takahashi, Kunihiko& Matsui, Shigeyuki. 2020. Effect-Size Estimation Using Semiparametric Hierarchical Mixture Models in Disease-Association Studies with Neuroimaging Data. Computational and Mathematical Methods in Medicine،Vol. 2020, no. 2020, pp.1-11.
https://search.emarefa.net/detail/BIM-1139569
نمط استشهاد الجمعية الأمريكية للغات الحديثة (MLA)
Emoto, Ryo…[et al.]. Effect-Size Estimation Using Semiparametric Hierarchical Mixture Models in Disease-Association Studies with Neuroimaging Data. Computational and Mathematical Methods in Medicine No. 2020 (2020), pp.1-11.
https://search.emarefa.net/detail/BIM-1139569
نمط استشهاد الجمعية الطبية الأمريكية (AMA)
Emoto, Ryo& Kawaguchi, Atsushi& Takahashi, Kunihiko& Matsui, Shigeyuki. Effect-Size Estimation Using Semiparametric Hierarchical Mixture Models in Disease-Association Studies with Neuroimaging Data. Computational and Mathematical Methods in Medicine. 2020. Vol. 2020, no. 2020, pp.1-11.
https://search.emarefa.net/detail/BIM-1139569
نوع البيانات
مقالات
لغة النص
الإنجليزية
الملاحظات
Includes bibliographical references
رقم السجل
BIM-1139569
قاعدة معامل التأثير والاستشهادات المرجعية العربي "ارسيف Arcif"
أضخم قاعدة بيانات عربية للاستشهادات المرجعية للمجلات العلمية المحكمة الصادرة في العالم العربي
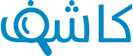
تقوم هذه الخدمة بالتحقق من التشابه أو الانتحال في الأبحاث والمقالات العلمية والأطروحات الجامعية والكتب والأبحاث باللغة العربية، وتحديد درجة التشابه أو أصالة الأعمال البحثية وحماية ملكيتها الفكرية. تعرف اكثر
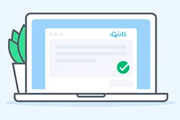