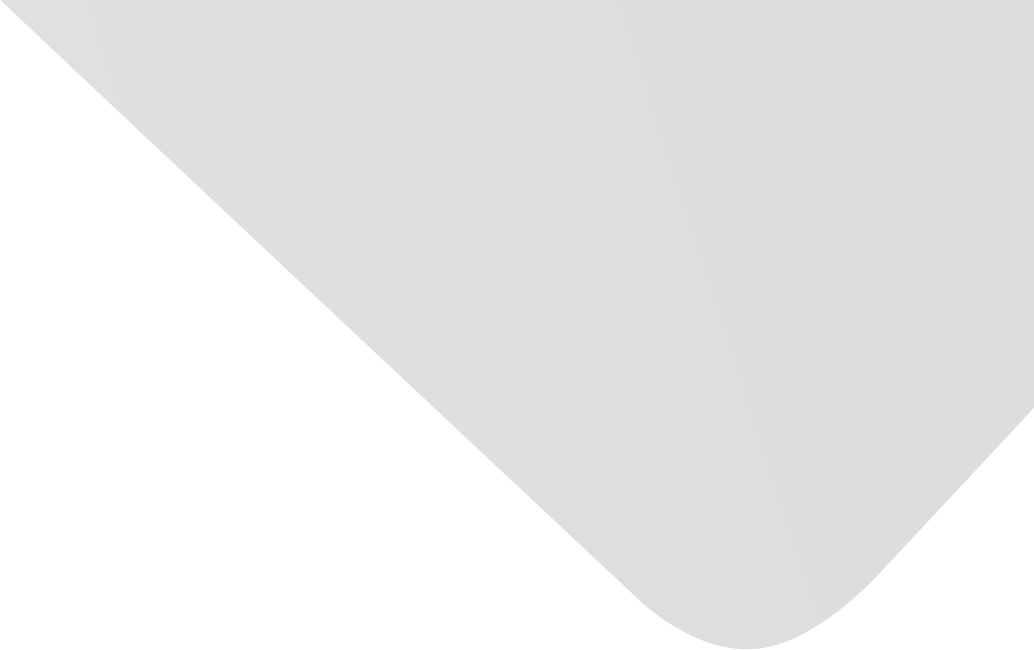
Effect-Size Estimation Using Semiparametric Hierarchical Mixture Models in Disease-Association Studies with Neuroimaging Data
Joint Authors
Matsui, Shigeyuki
Emoto, Ryo
Takahashi, Kunihiko
Kawaguchi, Atsushi
Source
Computational and Mathematical Methods in Medicine
Issue
Vol. 2020, Issue 2020 (31 Dec. 2020), pp.1-11, 11 p.
Publisher
Hindawi Publishing Corporation
Publication Date
2020-12-09
Country of Publication
Egypt
No. of Pages
11
Main Subjects
Abstract EN
In disease-association studies using neuroimaging data, evaluating the biological or clinical significance of individual associations requires not only detection of disease-associated areas of the brain but also estimation of the magnitudes of the associations or effect sizes for individual brain areas.
In this paper, we propose a model-based framework for voxel-based inferences under spatial dependency in neuroimaging data.
Specifically, we employ hierarchical mixture models with a hidden Markov random field structure to incorporate the spatial dependency between voxels.
A nonparametric specification is proposed for the effect size distribution to flexibly estimate the underlying effect size distribution.
Simulation experiments demonstrate that compared with a naive estimation method, the proposed methods can substantially reduce the selection bias in the effect size estimates of the selected voxels with the greatest observed associations.
An application to neuroimaging data from an Alzheimer’s disease study is provided.
American Psychological Association (APA)
Emoto, Ryo& Kawaguchi, Atsushi& Takahashi, Kunihiko& Matsui, Shigeyuki. 2020. Effect-Size Estimation Using Semiparametric Hierarchical Mixture Models in Disease-Association Studies with Neuroimaging Data. Computational and Mathematical Methods in Medicine،Vol. 2020, no. 2020, pp.1-11.
https://search.emarefa.net/detail/BIM-1139569
Modern Language Association (MLA)
Emoto, Ryo…[et al.]. Effect-Size Estimation Using Semiparametric Hierarchical Mixture Models in Disease-Association Studies with Neuroimaging Data. Computational and Mathematical Methods in Medicine No. 2020 (2020), pp.1-11.
https://search.emarefa.net/detail/BIM-1139569
American Medical Association (AMA)
Emoto, Ryo& Kawaguchi, Atsushi& Takahashi, Kunihiko& Matsui, Shigeyuki. Effect-Size Estimation Using Semiparametric Hierarchical Mixture Models in Disease-Association Studies with Neuroimaging Data. Computational and Mathematical Methods in Medicine. 2020. Vol. 2020, no. 2020, pp.1-11.
https://search.emarefa.net/detail/BIM-1139569
Data Type
Journal Articles
Language
English
Notes
Includes bibliographical references
Record ID
BIM-1139569