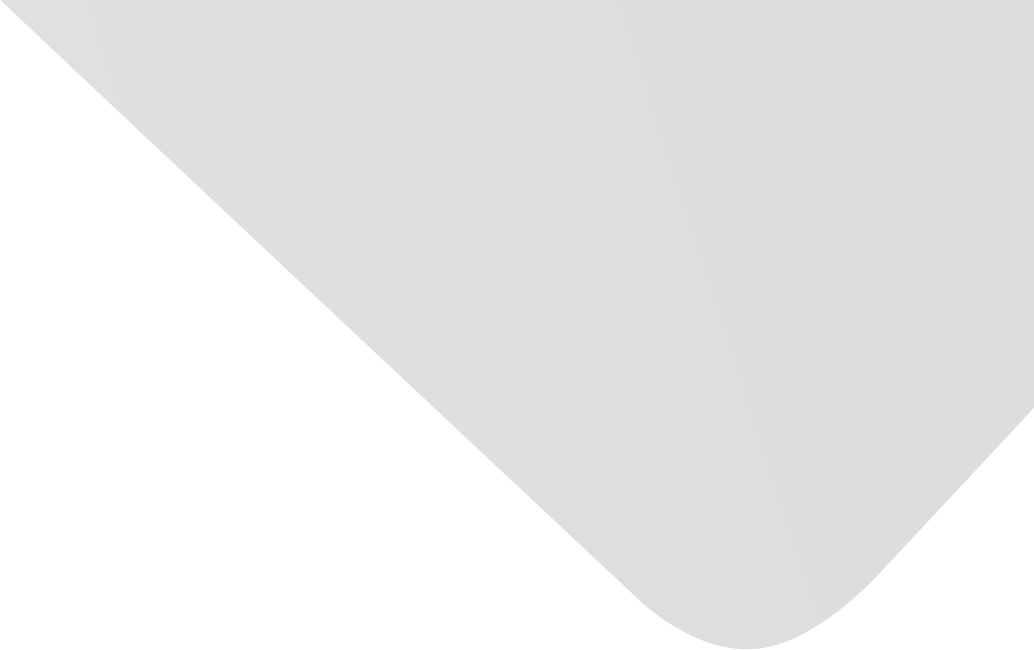
Prediction for Chaotic Time Series-Based AE-CNN and Transfer Learning
المؤلفون المشاركون
المصدر
العدد
المجلد 2020، العدد 2020 (31 ديسمبر/كانون الأول 2020)، ص ص. 1-9، 9ص.
الناشر
Hindawi Publishing Corporation
تاريخ النشر
2020-09-16
دولة النشر
مصر
عدد الصفحات
9
التخصصات الرئيسية
الملخص EN
It has been a hot and challenging topic to predict the chaotic time series in the medium-to-long term.
We combine autoencoders and convolutional neural networks (AE-CNN) to capture the intrinsic certainty of chaotic time series.
We utilize the transfer learning (TL) theory to improve the prediction performance in medium-to-long term.
Thus, we develop a prediction scheme for chaotic time series-based AE-CNN and TL named AE-CNN-TL.
Our experimental results show that the proposed AE-CNN-TL has much better prediction performance than any one of the following: AE-CNN, ARMA, and LSTM.
نمط استشهاد جمعية علماء النفس الأمريكية (APA)
Xin, Baogui& Peng, Wei. 2020. Prediction for Chaotic Time Series-Based AE-CNN and Transfer Learning. Complexity،Vol. 2020, no. 2020, pp.1-9.
https://search.emarefa.net/detail/BIM-1141145
نمط استشهاد الجمعية الأمريكية للغات الحديثة (MLA)
Xin, Baogui& Peng, Wei. Prediction for Chaotic Time Series-Based AE-CNN and Transfer Learning. Complexity No. 2020 (2020), pp.1-9.
https://search.emarefa.net/detail/BIM-1141145
نمط استشهاد الجمعية الطبية الأمريكية (AMA)
Xin, Baogui& Peng, Wei. Prediction for Chaotic Time Series-Based AE-CNN and Transfer Learning. Complexity. 2020. Vol. 2020, no. 2020, pp.1-9.
https://search.emarefa.net/detail/BIM-1141145
نوع البيانات
مقالات
لغة النص
الإنجليزية
الملاحظات
Includes bibliographical references
رقم السجل
BIM-1141145
قاعدة معامل التأثير والاستشهادات المرجعية العربي "ارسيف Arcif"
أضخم قاعدة بيانات عربية للاستشهادات المرجعية للمجلات العلمية المحكمة الصادرة في العالم العربي
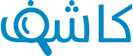
تقوم هذه الخدمة بالتحقق من التشابه أو الانتحال في الأبحاث والمقالات العلمية والأطروحات الجامعية والكتب والأبحاث باللغة العربية، وتحديد درجة التشابه أو أصالة الأعمال البحثية وحماية ملكيتها الفكرية. تعرف اكثر
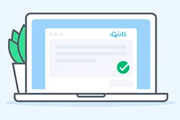