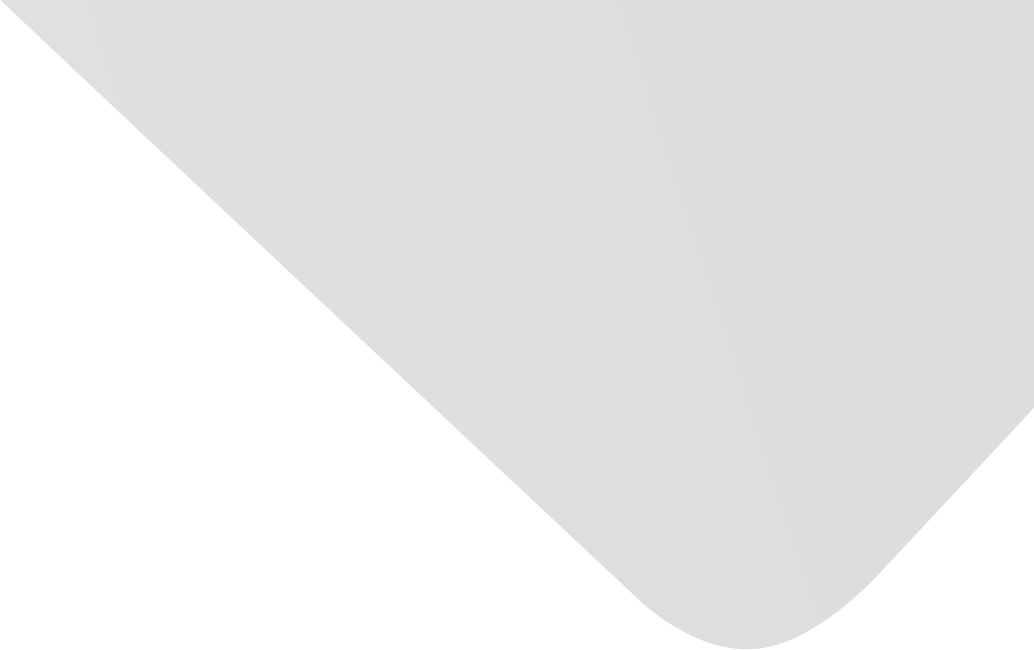
Prediction for Chaotic Time Series-Based AE-CNN and Transfer Learning
Joint Authors
Source
Issue
Vol. 2020, Issue 2020 (31 Dec. 2020), pp.1-9, 9 p.
Publisher
Hindawi Publishing Corporation
Publication Date
2020-09-16
Country of Publication
Egypt
No. of Pages
9
Main Subjects
Abstract EN
It has been a hot and challenging topic to predict the chaotic time series in the medium-to-long term.
We combine autoencoders and convolutional neural networks (AE-CNN) to capture the intrinsic certainty of chaotic time series.
We utilize the transfer learning (TL) theory to improve the prediction performance in medium-to-long term.
Thus, we develop a prediction scheme for chaotic time series-based AE-CNN and TL named AE-CNN-TL.
Our experimental results show that the proposed AE-CNN-TL has much better prediction performance than any one of the following: AE-CNN, ARMA, and LSTM.
American Psychological Association (APA)
Xin, Baogui& Peng, Wei. 2020. Prediction for Chaotic Time Series-Based AE-CNN and Transfer Learning. Complexity،Vol. 2020, no. 2020, pp.1-9.
https://search.emarefa.net/detail/BIM-1141145
Modern Language Association (MLA)
Xin, Baogui& Peng, Wei. Prediction for Chaotic Time Series-Based AE-CNN and Transfer Learning. Complexity No. 2020 (2020), pp.1-9.
https://search.emarefa.net/detail/BIM-1141145
American Medical Association (AMA)
Xin, Baogui& Peng, Wei. Prediction for Chaotic Time Series-Based AE-CNN and Transfer Learning. Complexity. 2020. Vol. 2020, no. 2020, pp.1-9.
https://search.emarefa.net/detail/BIM-1141145
Data Type
Journal Articles
Language
English
Notes
Includes bibliographical references
Record ID
BIM-1141145