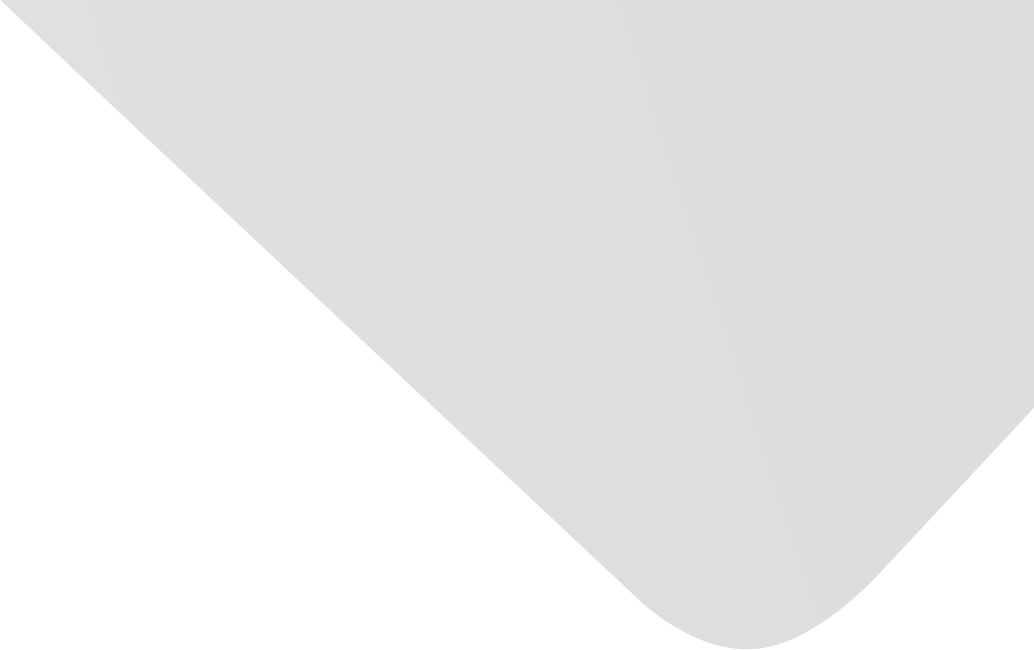
Hybrid Online and Offline Reinforcement Learning for Tibetan Jiu Chess
المؤلفون المشاركون
Li, Xiali
Lv, Zhengyu
Wu, Licheng
Zhao, Yue
Xu, Xiaona
المصدر
العدد
المجلد 2020، العدد 2020 (31 ديسمبر/كانون الأول 2020)، ص ص. 1-11، 11ص.
الناشر
Hindawi Publishing Corporation
تاريخ النشر
2020-05-11
دولة النشر
مصر
عدد الصفحات
11
التخصصات الرئيسية
الملخص EN
In this study, hybrid state-action-reward-state-action (SARSAλ) and Q-learning algorithms are applied to different stages of an upper confidence bound applied to tree search for Tibetan Jiu chess.
Q-learning is also used to update all the nodes on the search path when each game ends.
A learning strategy that uses SARSAλ and Q-learning algorithms combining domain knowledge for a feedback function for layout and battle stages is proposed.
An improved deep neural network based on ResNet18 is used for self-play training.
Experimental results show that hybrid online and offline reinforcement learning with a deep neural network can improve the game program’s learning efficiency and understanding ability for Tibetan Jiu chess.
نمط استشهاد جمعية علماء النفس الأمريكية (APA)
Li, Xiali& Lv, Zhengyu& Wu, Licheng& Zhao, Yue& Xu, Xiaona. 2020. Hybrid Online and Offline Reinforcement Learning for Tibetan Jiu Chess. Complexity،Vol. 2020, no. 2020, pp.1-11.
https://search.emarefa.net/detail/BIM-1142046
نمط استشهاد الجمعية الأمريكية للغات الحديثة (MLA)
Li, Xiali…[et al.]. Hybrid Online and Offline Reinforcement Learning for Tibetan Jiu Chess. Complexity No. 2020 (2020), pp.1-11.
https://search.emarefa.net/detail/BIM-1142046
نمط استشهاد الجمعية الطبية الأمريكية (AMA)
Li, Xiali& Lv, Zhengyu& Wu, Licheng& Zhao, Yue& Xu, Xiaona. Hybrid Online and Offline Reinforcement Learning for Tibetan Jiu Chess. Complexity. 2020. Vol. 2020, no. 2020, pp.1-11.
https://search.emarefa.net/detail/BIM-1142046
نوع البيانات
مقالات
لغة النص
الإنجليزية
الملاحظات
Includes bibliographical references
رقم السجل
BIM-1142046
قاعدة معامل التأثير والاستشهادات المرجعية العربي "ارسيف Arcif"
أضخم قاعدة بيانات عربية للاستشهادات المرجعية للمجلات العلمية المحكمة الصادرة في العالم العربي
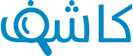
تقوم هذه الخدمة بالتحقق من التشابه أو الانتحال في الأبحاث والمقالات العلمية والأطروحات الجامعية والكتب والأبحاث باللغة العربية، وتحديد درجة التشابه أو أصالة الأعمال البحثية وحماية ملكيتها الفكرية. تعرف اكثر
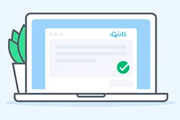