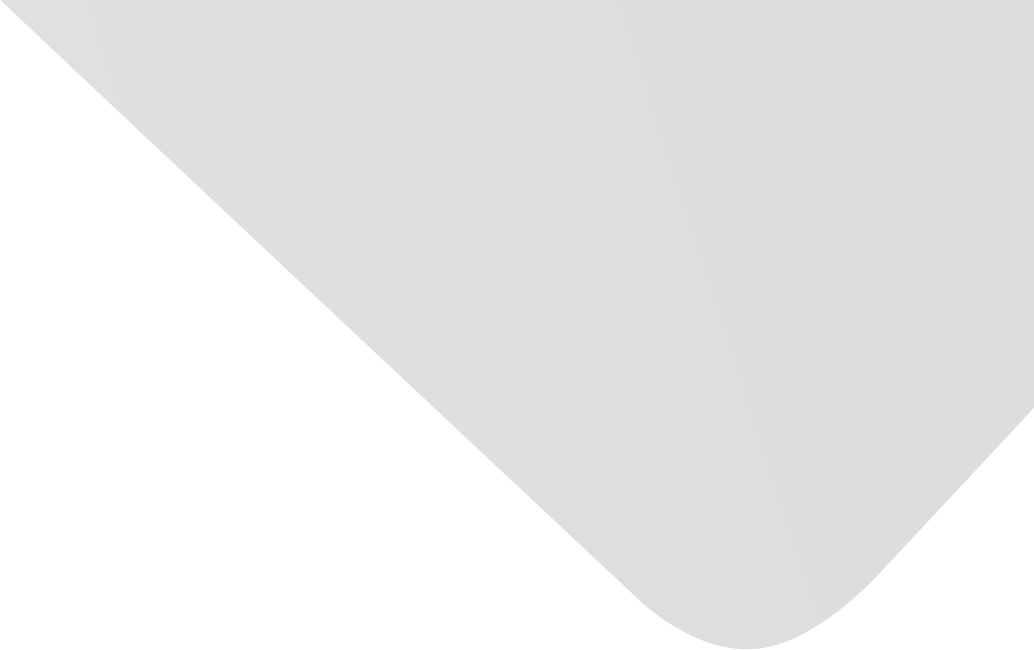
Hybrid Online and Offline Reinforcement Learning for Tibetan Jiu Chess
Joint Authors
Li, Xiali
Lv, Zhengyu
Wu, Licheng
Zhao, Yue
Xu, Xiaona
Source
Issue
Vol. 2020, Issue 2020 (31 Dec. 2020), pp.1-11, 11 p.
Publisher
Hindawi Publishing Corporation
Publication Date
2020-05-11
Country of Publication
Egypt
No. of Pages
11
Main Subjects
Abstract EN
In this study, hybrid state-action-reward-state-action (SARSAλ) and Q-learning algorithms are applied to different stages of an upper confidence bound applied to tree search for Tibetan Jiu chess.
Q-learning is also used to update all the nodes on the search path when each game ends.
A learning strategy that uses SARSAλ and Q-learning algorithms combining domain knowledge for a feedback function for layout and battle stages is proposed.
An improved deep neural network based on ResNet18 is used for self-play training.
Experimental results show that hybrid online and offline reinforcement learning with a deep neural network can improve the game program’s learning efficiency and understanding ability for Tibetan Jiu chess.
American Psychological Association (APA)
Li, Xiali& Lv, Zhengyu& Wu, Licheng& Zhao, Yue& Xu, Xiaona. 2020. Hybrid Online and Offline Reinforcement Learning for Tibetan Jiu Chess. Complexity،Vol. 2020, no. 2020, pp.1-11.
https://search.emarefa.net/detail/BIM-1142046
Modern Language Association (MLA)
Li, Xiali…[et al.]. Hybrid Online and Offline Reinforcement Learning for Tibetan Jiu Chess. Complexity No. 2020 (2020), pp.1-11.
https://search.emarefa.net/detail/BIM-1142046
American Medical Association (AMA)
Li, Xiali& Lv, Zhengyu& Wu, Licheng& Zhao, Yue& Xu, Xiaona. Hybrid Online and Offline Reinforcement Learning for Tibetan Jiu Chess. Complexity. 2020. Vol. 2020, no. 2020, pp.1-11.
https://search.emarefa.net/detail/BIM-1142046
Data Type
Journal Articles
Language
English
Notes
Includes bibliographical references
Record ID
BIM-1142046