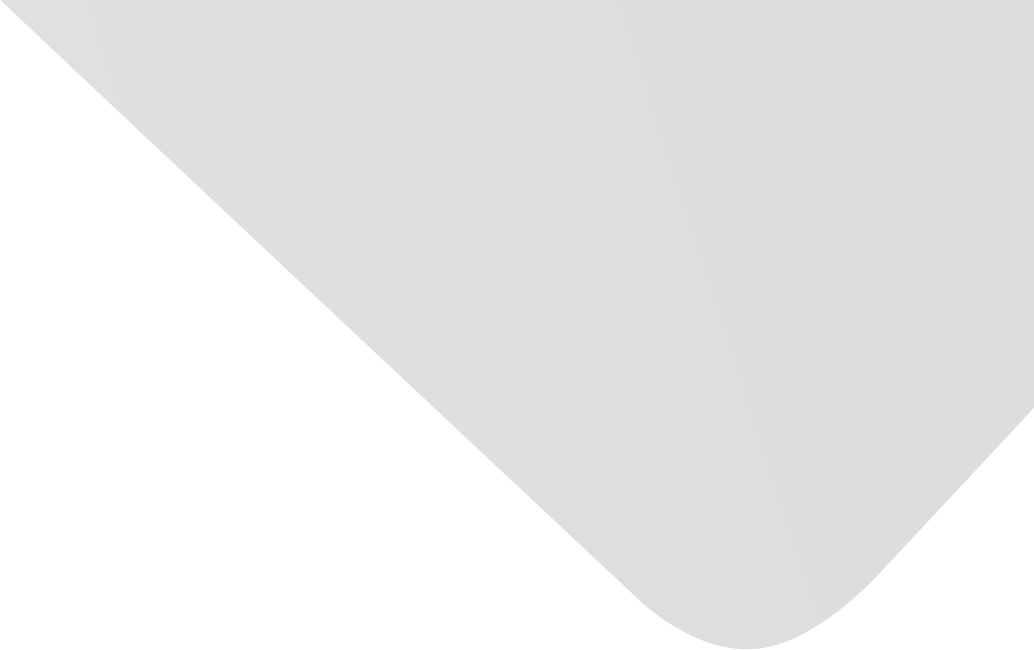
Effect Improved for High-Dimensional and Unbalanced Data Anomaly Detection Model Based on KNN-SMOTE-LSTM
المؤلفون المشاركون
Bao, Fuguang
Wu, Yongqiang
Li, Zhaogang
Li, Yongzhao
Chen, Guanyu
Liu, Lili
المصدر
العدد
المجلد 2020، العدد 2020 (31 ديسمبر/كانون الأول 2020)، ص ص. 1-17، 17ص.
الناشر
Hindawi Publishing Corporation
تاريخ النشر
2020-09-17
دولة النشر
مصر
عدد الصفحات
17
التخصصات الرئيسية
الملخص EN
High-dimensional and unbalanced data anomaly detection is common.
Effective anomaly detection is essential for problem or disaster early warning and maintaining system reliability.
A significant research issue related to the data analysis of the sensor is the detection of anomalies.
The anomaly detection is essentially an unbalanced sequence binary classification.
The data of this type contains characteristics of large scale, high complex computation, unbalanced data distribution, and sequence relationship among data.
This paper uses long short-term memory networks (LSTMs) combined with historical sequence data; also, it integrates the synthetic minority oversampling technique (SMOTE) algorithm and K-nearest neighbors (kNN), and it designs and constructs an anomaly detection network model based on kNN-SMOTE-LSTM in accordance with the data characteristic of being unbalanced.
This model can continuously filter out and securely generate samples to improve the performance of the model through kNN discriminant classifier and avoid the blindness and limitations of the SMOTE algorithm in generating new samples.
The experiments demonstrated that the structured kNN-SMOTE-LSTM model can significantly improve the performance of the unbalanced sequence binary classification.
نمط استشهاد جمعية علماء النفس الأمريكية (APA)
Bao, Fuguang& Wu, Yongqiang& Li, Zhaogang& Li, Yongzhao& Liu, Lili& Chen, Guanyu. 2020. Effect Improved for High-Dimensional and Unbalanced Data Anomaly Detection Model Based on KNN-SMOTE-LSTM. Complexity،Vol. 2020, no. 2020, pp.1-17.
https://search.emarefa.net/detail/BIM-1145394
نمط استشهاد الجمعية الأمريكية للغات الحديثة (MLA)
Bao, Fuguang…[et al.]. Effect Improved for High-Dimensional and Unbalanced Data Anomaly Detection Model Based on KNN-SMOTE-LSTM. Complexity No. 2020 (2020), pp.1-17.
https://search.emarefa.net/detail/BIM-1145394
نمط استشهاد الجمعية الطبية الأمريكية (AMA)
Bao, Fuguang& Wu, Yongqiang& Li, Zhaogang& Li, Yongzhao& Liu, Lili& Chen, Guanyu. Effect Improved for High-Dimensional and Unbalanced Data Anomaly Detection Model Based on KNN-SMOTE-LSTM. Complexity. 2020. Vol. 2020, no. 2020, pp.1-17.
https://search.emarefa.net/detail/BIM-1145394
نوع البيانات
مقالات
لغة النص
الإنجليزية
الملاحظات
Includes bibliographical references
رقم السجل
BIM-1145394
قاعدة معامل التأثير والاستشهادات المرجعية العربي "ارسيف Arcif"
أضخم قاعدة بيانات عربية للاستشهادات المرجعية للمجلات العلمية المحكمة الصادرة في العالم العربي
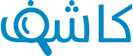
تقوم هذه الخدمة بالتحقق من التشابه أو الانتحال في الأبحاث والمقالات العلمية والأطروحات الجامعية والكتب والأبحاث باللغة العربية، وتحديد درجة التشابه أو أصالة الأعمال البحثية وحماية ملكيتها الفكرية. تعرف اكثر
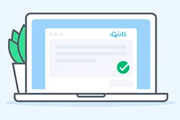