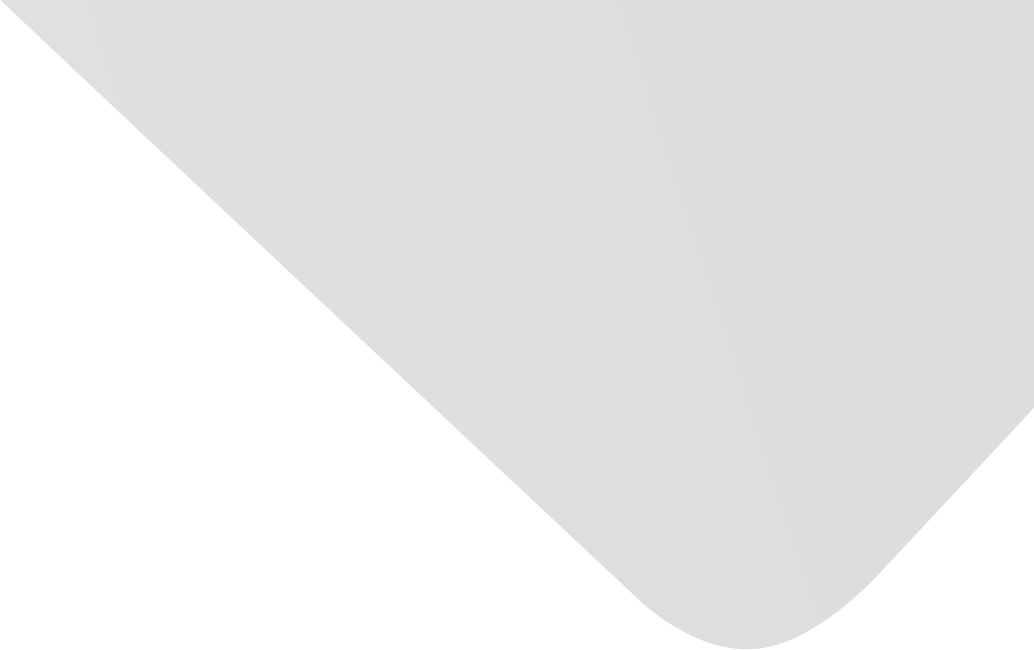
Effect Improved for High-Dimensional and Unbalanced Data Anomaly Detection Model Based on KNN-SMOTE-LSTM
Joint Authors
Bao, Fuguang
Wu, Yongqiang
Li, Zhaogang
Li, Yongzhao
Chen, Guanyu
Liu, Lili
Source
Issue
Vol. 2020, Issue 2020 (31 Dec. 2020), pp.1-17, 17 p.
Publisher
Hindawi Publishing Corporation
Publication Date
2020-09-17
Country of Publication
Egypt
No. of Pages
17
Main Subjects
Abstract EN
High-dimensional and unbalanced data anomaly detection is common.
Effective anomaly detection is essential for problem or disaster early warning and maintaining system reliability.
A significant research issue related to the data analysis of the sensor is the detection of anomalies.
The anomaly detection is essentially an unbalanced sequence binary classification.
The data of this type contains characteristics of large scale, high complex computation, unbalanced data distribution, and sequence relationship among data.
This paper uses long short-term memory networks (LSTMs) combined with historical sequence data; also, it integrates the synthetic minority oversampling technique (SMOTE) algorithm and K-nearest neighbors (kNN), and it designs and constructs an anomaly detection network model based on kNN-SMOTE-LSTM in accordance with the data characteristic of being unbalanced.
This model can continuously filter out and securely generate samples to improve the performance of the model through kNN discriminant classifier and avoid the blindness and limitations of the SMOTE algorithm in generating new samples.
The experiments demonstrated that the structured kNN-SMOTE-LSTM model can significantly improve the performance of the unbalanced sequence binary classification.
American Psychological Association (APA)
Bao, Fuguang& Wu, Yongqiang& Li, Zhaogang& Li, Yongzhao& Liu, Lili& Chen, Guanyu. 2020. Effect Improved for High-Dimensional and Unbalanced Data Anomaly Detection Model Based on KNN-SMOTE-LSTM. Complexity،Vol. 2020, no. 2020, pp.1-17.
https://search.emarefa.net/detail/BIM-1145394
Modern Language Association (MLA)
Bao, Fuguang…[et al.]. Effect Improved for High-Dimensional and Unbalanced Data Anomaly Detection Model Based on KNN-SMOTE-LSTM. Complexity No. 2020 (2020), pp.1-17.
https://search.emarefa.net/detail/BIM-1145394
American Medical Association (AMA)
Bao, Fuguang& Wu, Yongqiang& Li, Zhaogang& Li, Yongzhao& Liu, Lili& Chen, Guanyu. Effect Improved for High-Dimensional and Unbalanced Data Anomaly Detection Model Based on KNN-SMOTE-LSTM. Complexity. 2020. Vol. 2020, no. 2020, pp.1-17.
https://search.emarefa.net/detail/BIM-1145394
Data Type
Journal Articles
Language
English
Notes
Includes bibliographical references
Record ID
BIM-1145394