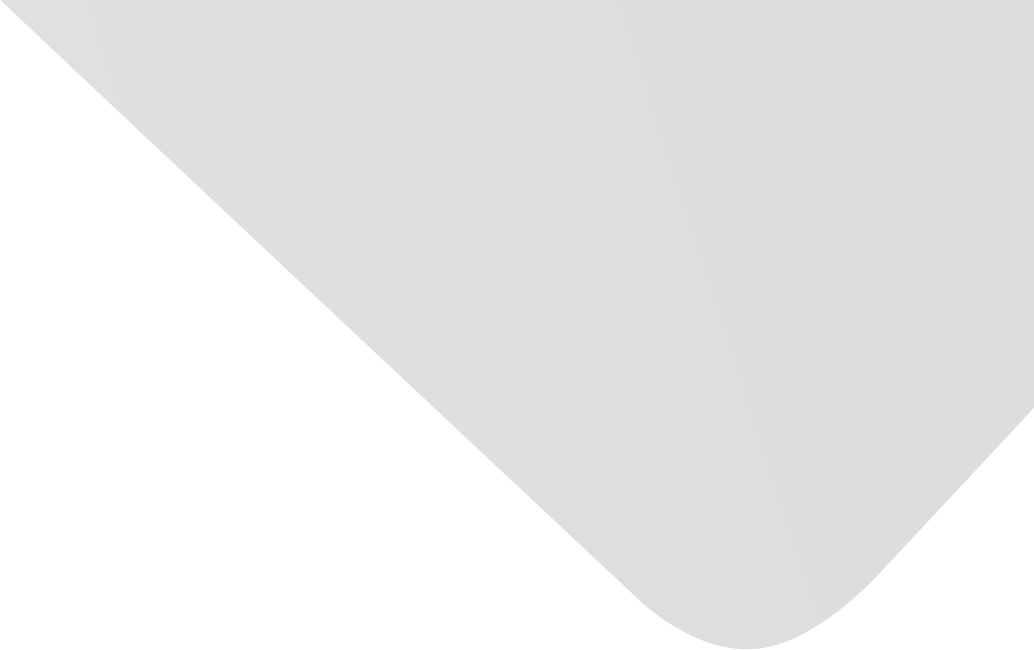
A New Video-Based Crash Detection Method: Balancing Speed and Accuracy Using a Feature Fusion Deep Learning Framework
المؤلفون المشاركون
Lu, Zhenbo
Zhou, Wei
Zhang, Shixiang
Wang, Chen
المصدر
Journal of Advanced Transportation
العدد
المجلد 2020، العدد 2020 (31 ديسمبر/كانون الأول 2020)، ص ص. 1-12، 12ص.
الناشر
Hindawi Publishing Corporation
تاريخ النشر
2020-11-16
دولة النشر
مصر
عدد الصفحات
12
التخصصات الرئيسية
الملخص EN
Quick and accurate crash detection is important for saving lives and improved traffic incident management.
In this paper, a feature fusion-based deep learning framework was developed for video-based urban traffic crash detection task, aiming at achieving a balance between detection speed and accuracy with limited computing resource.
In this framework, a residual neural network (ResNet) combined with attention modules was proposed to extract crash-related appearance features from urban traffic videos (i.e., a crash appearance feature extractor), which were further fed to a spatiotemporal feature fusion model, Conv-LSTM (Convolutional Long Short-Term Memory), to simultaneously capture appearance (static) and motion (dynamic) crash features.
The proposed model was trained by a set of video clips covering 330 crash and 342 noncrash events.
In general, the proposed model achieved an accuracy of 87.78% on the testing dataset and an acceptable detection speed (FPS > 30 with GTX 1060).
Thanks to the attention module, the proposed model can capture the localized appearance features (e.g., vehicle damage and pedestrian fallen-off) of crashes better than conventional convolutional neural networks.
The Conv-LSTM module outperformed conventional LSTM in terms of capturing motion features of crashes, such as the roadway congestion and pedestrians gathering after crashes.
Compared to traditional motion-based crash detection model, the proposed model achieved higher detection accuracy.
Moreover, it could detect crashes much faster than other feature fusion-based models (e.g., C3D).
The results show that the proposed model is a promising video-based urban traffic crash detection algorithm that could be used in practice in the future.
نمط استشهاد جمعية علماء النفس الأمريكية (APA)
Lu, Zhenbo& Zhou, Wei& Zhang, Shixiang& Wang, Chen. 2020. A New Video-Based Crash Detection Method: Balancing Speed and Accuracy Using a Feature Fusion Deep Learning Framework. Journal of Advanced Transportation،Vol. 2020, no. 2020, pp.1-12.
https://search.emarefa.net/detail/BIM-1176593
نمط استشهاد الجمعية الأمريكية للغات الحديثة (MLA)
Lu, Zhenbo…[et al.]. A New Video-Based Crash Detection Method: Balancing Speed and Accuracy Using a Feature Fusion Deep Learning Framework. Journal of Advanced Transportation No. 2020 (2020), pp.1-12.
https://search.emarefa.net/detail/BIM-1176593
نمط استشهاد الجمعية الطبية الأمريكية (AMA)
Lu, Zhenbo& Zhou, Wei& Zhang, Shixiang& Wang, Chen. A New Video-Based Crash Detection Method: Balancing Speed and Accuracy Using a Feature Fusion Deep Learning Framework. Journal of Advanced Transportation. 2020. Vol. 2020, no. 2020, pp.1-12.
https://search.emarefa.net/detail/BIM-1176593
نوع البيانات
مقالات
لغة النص
الإنجليزية
الملاحظات
Includes bibliographical references
رقم السجل
BIM-1176593
قاعدة معامل التأثير والاستشهادات المرجعية العربي "ارسيف Arcif"
أضخم قاعدة بيانات عربية للاستشهادات المرجعية للمجلات العلمية المحكمة الصادرة في العالم العربي
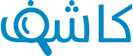
تقوم هذه الخدمة بالتحقق من التشابه أو الانتحال في الأبحاث والمقالات العلمية والأطروحات الجامعية والكتب والأبحاث باللغة العربية، وتحديد درجة التشابه أو أصالة الأعمال البحثية وحماية ملكيتها الفكرية. تعرف اكثر
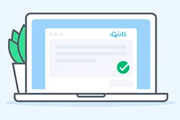