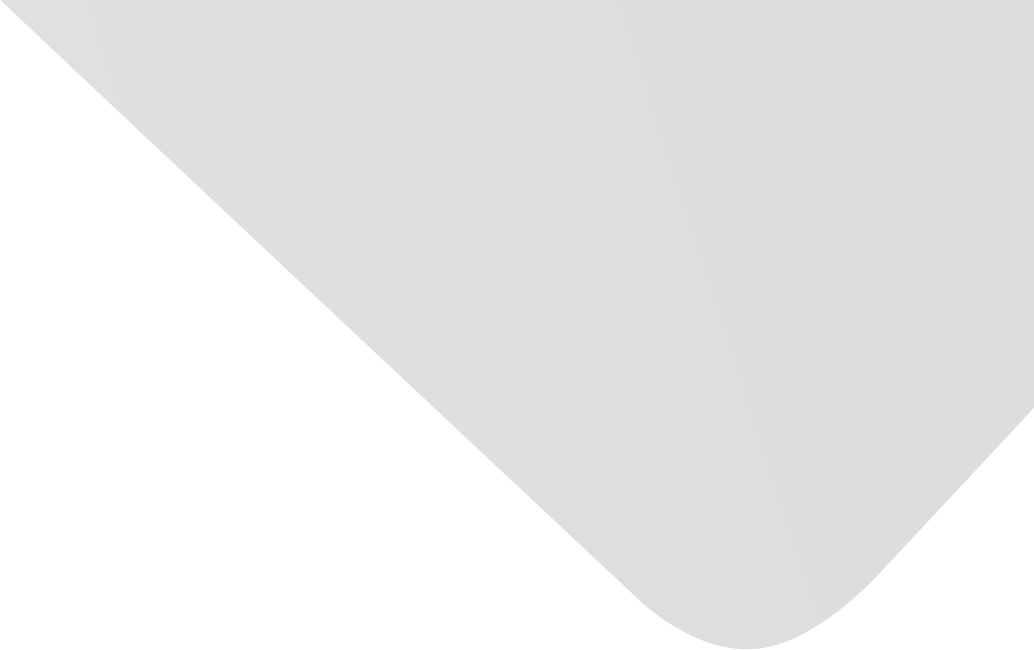
A New Video-Based Crash Detection Method: Balancing Speed and Accuracy Using a Feature Fusion Deep Learning Framework
Joint Authors
Lu, Zhenbo
Zhou, Wei
Zhang, Shixiang
Wang, Chen
Source
Journal of Advanced Transportation
Issue
Vol. 2020, Issue 2020 (31 Dec. 2020), pp.1-12, 12 p.
Publisher
Hindawi Publishing Corporation
Publication Date
2020-11-16
Country of Publication
Egypt
No. of Pages
12
Main Subjects
Abstract EN
Quick and accurate crash detection is important for saving lives and improved traffic incident management.
In this paper, a feature fusion-based deep learning framework was developed for video-based urban traffic crash detection task, aiming at achieving a balance between detection speed and accuracy with limited computing resource.
In this framework, a residual neural network (ResNet) combined with attention modules was proposed to extract crash-related appearance features from urban traffic videos (i.e., a crash appearance feature extractor), which were further fed to a spatiotemporal feature fusion model, Conv-LSTM (Convolutional Long Short-Term Memory), to simultaneously capture appearance (static) and motion (dynamic) crash features.
The proposed model was trained by a set of video clips covering 330 crash and 342 noncrash events.
In general, the proposed model achieved an accuracy of 87.78% on the testing dataset and an acceptable detection speed (FPS > 30 with GTX 1060).
Thanks to the attention module, the proposed model can capture the localized appearance features (e.g., vehicle damage and pedestrian fallen-off) of crashes better than conventional convolutional neural networks.
The Conv-LSTM module outperformed conventional LSTM in terms of capturing motion features of crashes, such as the roadway congestion and pedestrians gathering after crashes.
Compared to traditional motion-based crash detection model, the proposed model achieved higher detection accuracy.
Moreover, it could detect crashes much faster than other feature fusion-based models (e.g., C3D).
The results show that the proposed model is a promising video-based urban traffic crash detection algorithm that could be used in practice in the future.
American Psychological Association (APA)
Lu, Zhenbo& Zhou, Wei& Zhang, Shixiang& Wang, Chen. 2020. A New Video-Based Crash Detection Method: Balancing Speed and Accuracy Using a Feature Fusion Deep Learning Framework. Journal of Advanced Transportation،Vol. 2020, no. 2020, pp.1-12.
https://search.emarefa.net/detail/BIM-1176593
Modern Language Association (MLA)
Lu, Zhenbo…[et al.]. A New Video-Based Crash Detection Method: Balancing Speed and Accuracy Using a Feature Fusion Deep Learning Framework. Journal of Advanced Transportation No. 2020 (2020), pp.1-12.
https://search.emarefa.net/detail/BIM-1176593
American Medical Association (AMA)
Lu, Zhenbo& Zhou, Wei& Zhang, Shixiang& Wang, Chen. A New Video-Based Crash Detection Method: Balancing Speed and Accuracy Using a Feature Fusion Deep Learning Framework. Journal of Advanced Transportation. 2020. Vol. 2020, no. 2020, pp.1-12.
https://search.emarefa.net/detail/BIM-1176593
Data Type
Journal Articles
Language
English
Notes
Includes bibliographical references
Record ID
BIM-1176593