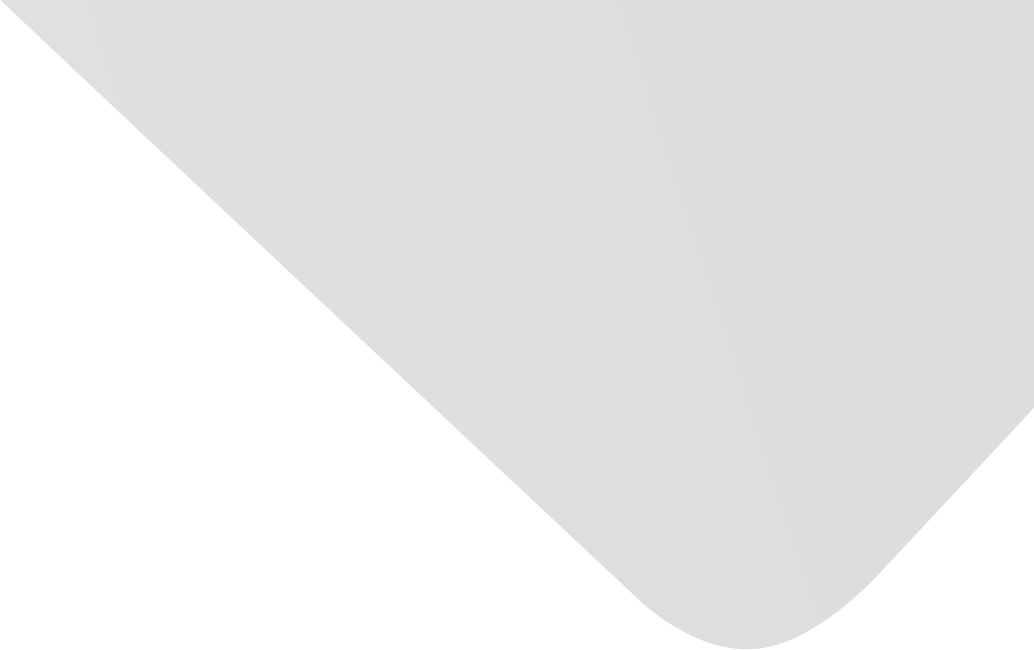
Improving Rolling Bearing Fault Diagnosis by DS Evidence Theory Based Fusion Model
المؤلفون المشاركون
Yao, Xuemei
Li, Shaobo
Hu, Jianjun
المصدر
العدد
المجلد 2017، العدد 2017 (31 ديسمبر/كانون الأول 2017)، ص ص. 1-14، 14ص.
الناشر
Hindawi Publishing Corporation
تاريخ النشر
2017-10-22
دولة النشر
مصر
عدد الصفحات
14
التخصصات الرئيسية
الملخص EN
Rolling bearing plays an important role in rotating machinery and its working condition directly affects the equipment efficiency.
While dozens of methods have been proposed for real-time bearing fault diagnosis and monitoring, the fault classification accuracy of existing algorithms is still not satisfactory.
This work presents a novel algorithm fusion model based on principal component analysis and Dempster-Shafer evidence theory for rolling bearing fault diagnosis.
It combines the advantages of the learning vector quantization (LVQ) neural network model and the decision tree model.
Experiments under three different spinning bearing speeds and two different crack sizes show that our fusion model has better performance and higher accuracy than either of the base classification models for rolling bearing fault diagnosis, which is achieved via synergic prediction from both types of models.
نمط استشهاد جمعية علماء النفس الأمريكية (APA)
Yao, Xuemei& Li, Shaobo& Hu, Jianjun. 2017. Improving Rolling Bearing Fault Diagnosis by DS Evidence Theory Based Fusion Model. Journal of Sensors،Vol. 2017, no. 2017, pp.1-14.
https://search.emarefa.net/detail/BIM-1187225
نمط استشهاد الجمعية الأمريكية للغات الحديثة (MLA)
Yao, Xuemei…[et al.]. Improving Rolling Bearing Fault Diagnosis by DS Evidence Theory Based Fusion Model. Journal of Sensors No. 2017 (2017), pp.1-14.
https://search.emarefa.net/detail/BIM-1187225
نمط استشهاد الجمعية الطبية الأمريكية (AMA)
Yao, Xuemei& Li, Shaobo& Hu, Jianjun. Improving Rolling Bearing Fault Diagnosis by DS Evidence Theory Based Fusion Model. Journal of Sensors. 2017. Vol. 2017, no. 2017, pp.1-14.
https://search.emarefa.net/detail/BIM-1187225
نوع البيانات
مقالات
لغة النص
الإنجليزية
الملاحظات
Includes bibliographical references
رقم السجل
BIM-1187225
قاعدة معامل التأثير والاستشهادات المرجعية العربي "ارسيف Arcif"
أضخم قاعدة بيانات عربية للاستشهادات المرجعية للمجلات العلمية المحكمة الصادرة في العالم العربي
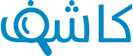
تقوم هذه الخدمة بالتحقق من التشابه أو الانتحال في الأبحاث والمقالات العلمية والأطروحات الجامعية والكتب والأبحاث باللغة العربية، وتحديد درجة التشابه أو أصالة الأعمال البحثية وحماية ملكيتها الفكرية. تعرف اكثر
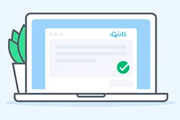